Comprehensive Integration of Hyperdimensional Computing with Deep Learning towards Neuro-Symbolic AI.
DAC(2023)
摘要
HD computing is a symbolic representation system which performs various learning tasks in a highly-parallelizable and binary-centric way by drawing inspiration from concepts in human long-term memory. However, the current HD computing is ineffective in extracting high-level feature information for image data. In this paper, we present a neuro-symbolic approach called NSHD, which integrates CNNs and Hyperdimensional (HD) learning techniques to provide efficient learning with state-of-the-art quality. We devise the HD training procedure, which fully integrates knowledge from the deep learning model through a distillation process with optimized computation costs due to the integration. Our experimental results show that NSHD provides high energy efficiency as compared to CNN, e.g., up to 64% with comparable accuracy, and can outperform the learning quality when more computing resources are allowed. We also show the symbolic nature of the NSHD can make the learning humnan-interpretable by exploiting the property of HD computing.
更多查看译文
关键词
HD Computing, Knowledge Distillation, Neuro-symbolic AI
AI 理解论文
溯源树
样例
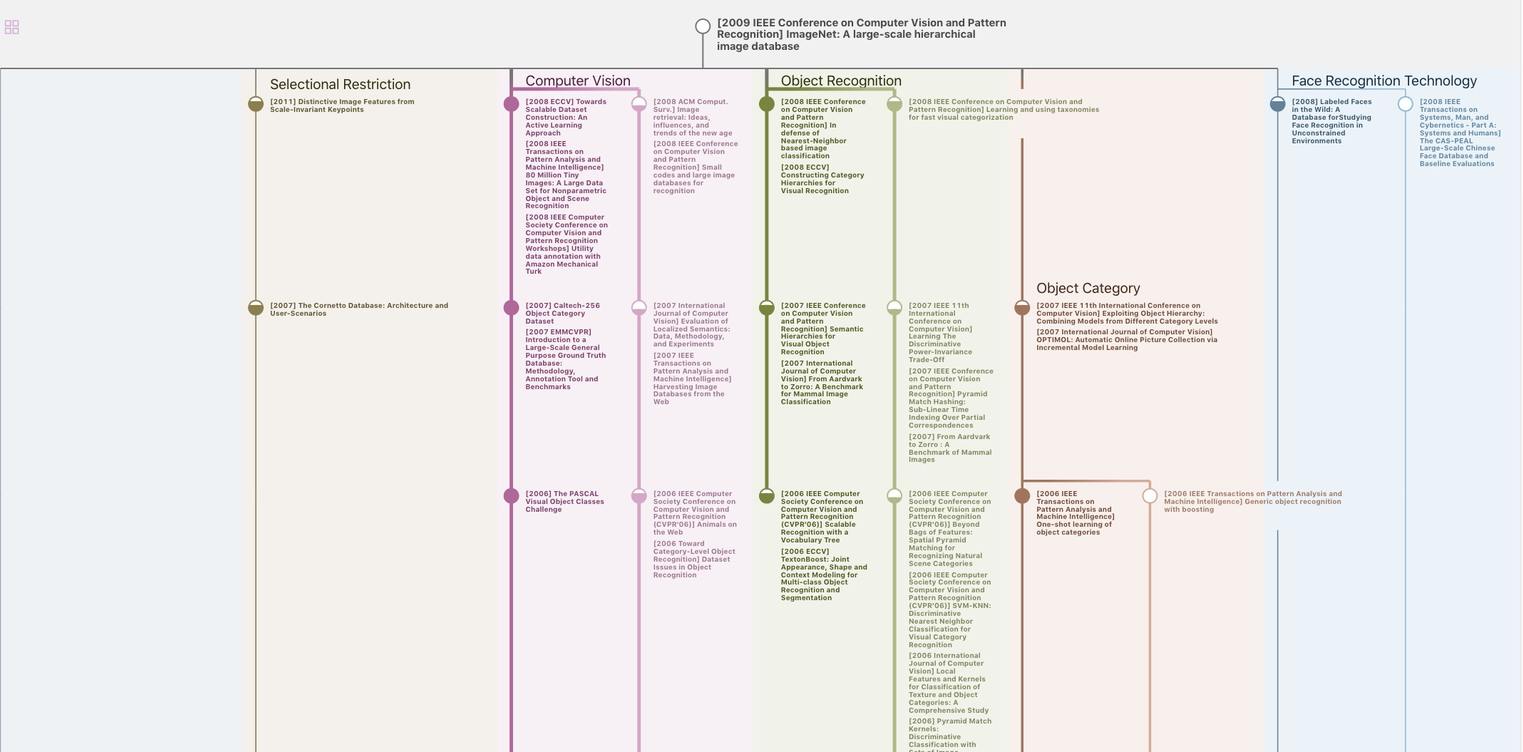
生成溯源树,研究论文发展脉络
Chat Paper
正在生成论文摘要