A comprehensive evaluation of large Language models on benchmark biomedical text processing tasks
COMPUTERS IN BIOLOGY AND MEDICINE(2024)
摘要
Recently, Large Language Models (LLMs) have demonstrated impressive capability to solve a wide range of tasks. However, despite their success across various tasks, no prior work has investigated their capability in the biomedical domain yet. To this end, this paper aims to evaluate the performance of LLMs on benchmark biomedical tasks. For this purpose, a comprehensive evaluation of 4 popular LLMs in 6 diverse biomedical tasks across 26 datasets has been conducted. To the best of our knowledge, this is the first work that conducts an extensive evaluation and comparison of various LLMs in the biomedical domain. Interestingly, we find based on our evaluation that in biomedical datasets that have smaller training sets, zero -shot LLMs even outperform the current state-of-the-art models when they were fine-tuned only on the training set of these datasets. This suggests that pre -training on large text corpora makes LLMs quite specialized even in the biomedical domain. We also find that not a single LLM can outperform other LLMs in all tasks, with the performance of different LLMs may vary depending on the task. While their performance is still quite poor in comparison to the biomedical models that were fine-tuned on large training sets, our findings demonstrate that LLMs have the potential to be a valuable tool for various biomedical tasks that lack large annotated data.
更多查看译文
关键词
Large language models,ChatGPT,PaLM,LLaMA,Claude,Transformer,Natural language processing,LLM evaluation
AI 理解论文
溯源树
样例
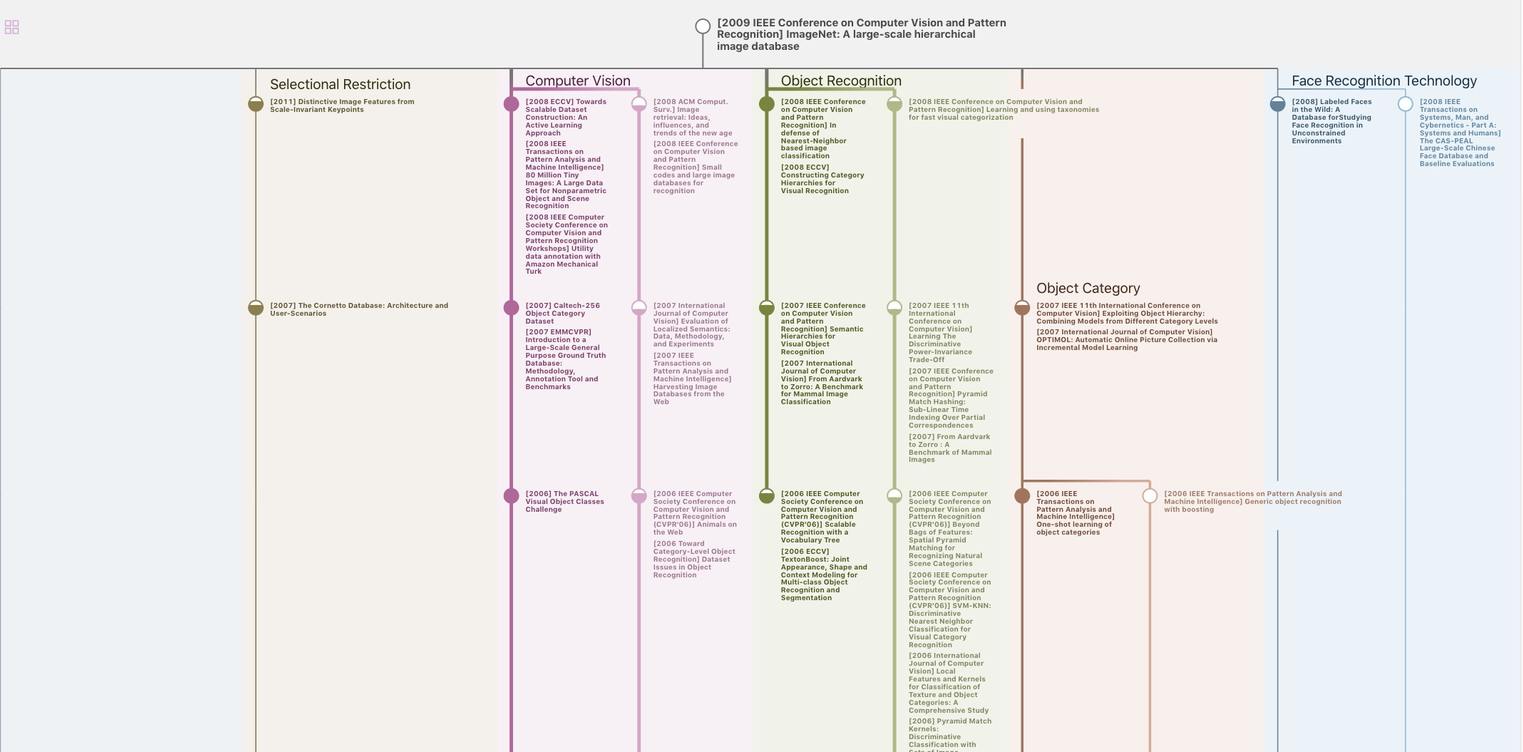
生成溯源树,研究论文发展脉络
Chat Paper
正在生成论文摘要