Uncertainty-aware hybrid paradigm of nonlinear MPC and model-based RL for offroad navigation: Exploration of transformers in the predictive model
CoRR(2023)
摘要
In this paper, we investigate a hybrid scheme that combines nonlinear model predictive control (MPC) and model-based reinforcement learning (RL) for navigation planning of an autonomous model car across offroad, unstructured terrains without relying on predefined maps. Our innovative approach takes inspiration from BADGR, an LSTM-based network that primarily concentrates on environment modeling, but distinguishes itself by substituting LSTM modules with transformers to greatly elevate the performance our model. Addressing uncertainty within the system, we train an ensemble of predictive models and estimate the mutual information between model weights and outputs, facilitating dynamic horizon planning through the introduction of variable speeds. Further enhancing our methodology, we incorporate a nonlinear MPC controller that accounts for the intricacies of the vehicle's model and states. The model-based RL facet produces steering angles and quantifies inherent uncertainty. At the same time, the nonlinear MPC suggests optimal throttle settings, striking a balance between goal attainment speed and managing model uncertainty influenced by velocity. In the conducted studies, our approach excels over the existing baseline by consistently achieving higher metric values in predicting future events and seamlessly integrating the vehicle's kinematic model for enhanced decision-making. The code and the evaluation data are available at https://github.com/FARAZLOTFI/offroad_autonomous_navigation/).
更多查看译文
关键词
offroad navigation,nonlinear mpc,uncertainty-aware,model-based
AI 理解论文
溯源树
样例
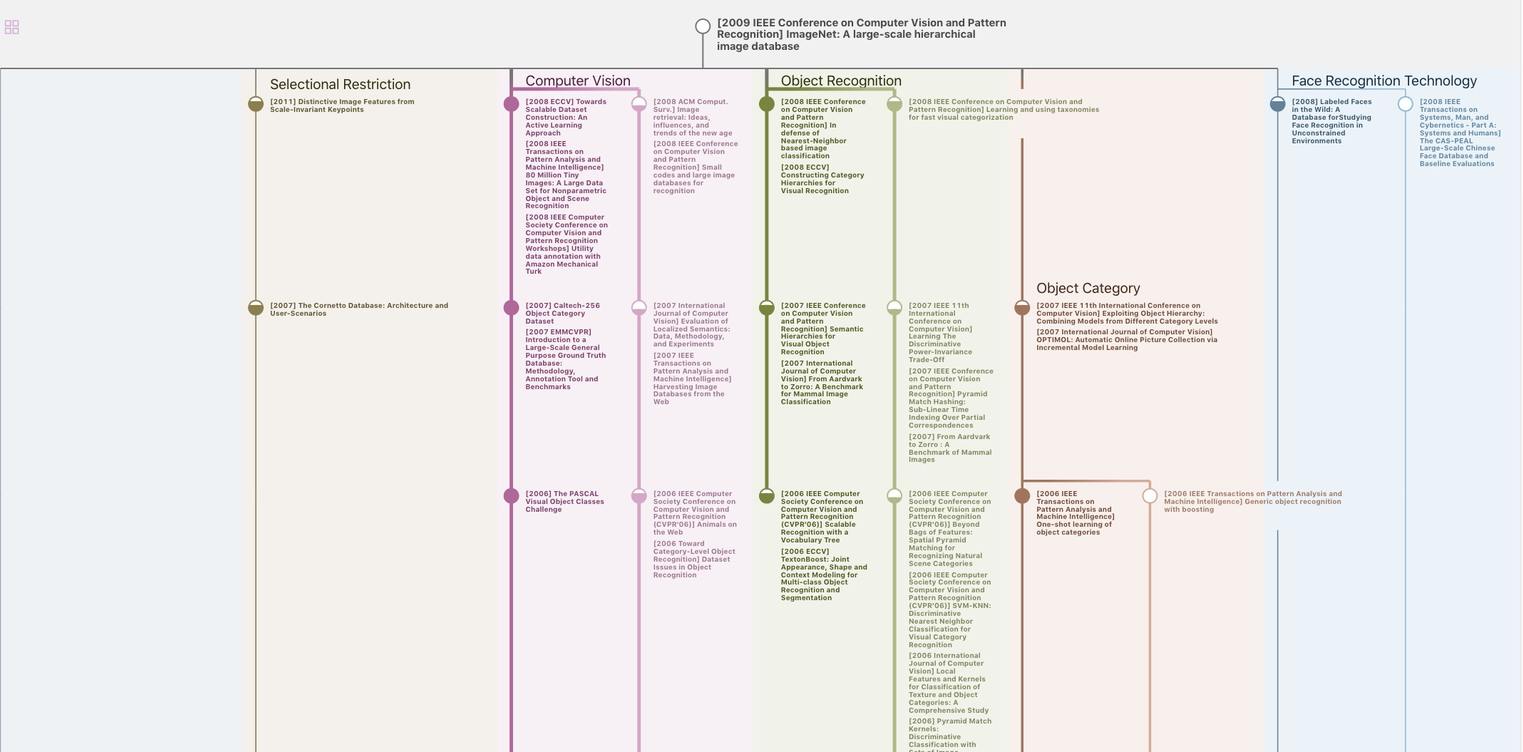
生成溯源树,研究论文发展脉络
Chat Paper
正在生成论文摘要