Training and inference of large language models using 8-bit floating point
CoRR(2023)
摘要
FP8 formats are gaining popularity to boost the computational efficiency for training and inference of large deep learning models. Their main challenge is that a careful choice of scaling is needed to prevent degradation due to the reduced dynamic range compared to higher-precision formats. Although there exists ample literature about selecting such scalings for INT formats, this critical aspect has yet to be addressed for FP8. This paper presents a methodology to select the scalings for FP8 linear layers, based on dynamically updating per-tensor scales for the weights, gradients and activations. We apply this methodology to train and validate large language models of the type of GPT and Llama 2 using FP8, for model sizes ranging from 111M to 70B. To facilitate the understanding of the FP8 dynamics, our results are accompanied by plots of the per-tensor scale distribution for weights, activations and gradients during both training and inference.
更多查看译文
关键词
large language models,inference
AI 理解论文
溯源树
样例
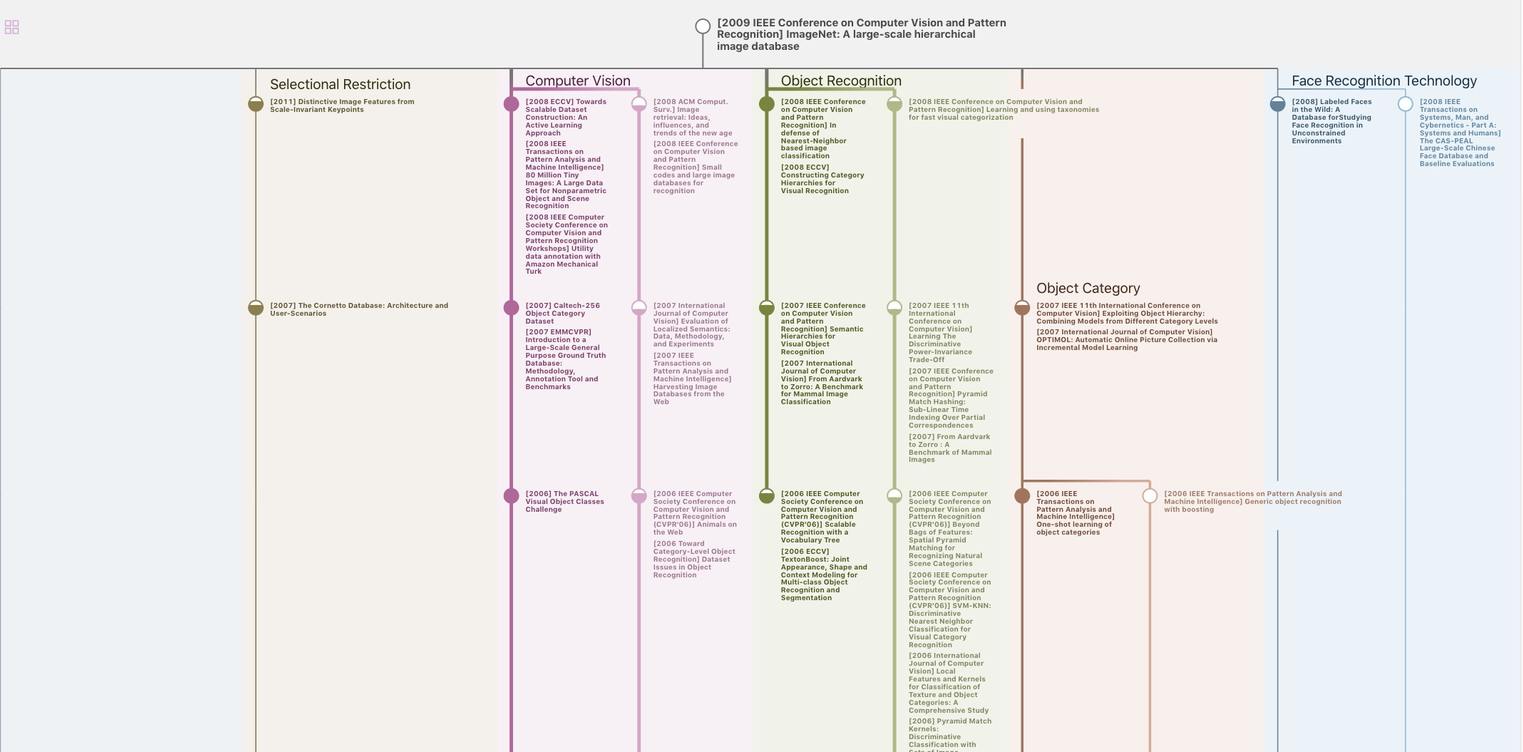
生成溯源树,研究论文发展脉络
Chat Paper
正在生成论文摘要