Beyond Co-Occurrence: Multi-Modal Session-Based Recommendation
IEEE TRANSACTIONS ON KNOWLEDGE AND DATA ENGINEERING(2024)
摘要
Session-based recommendation is devoted to characterizing preferences of anonymous users based on short sessions. Existing methods mostly focus on mining limited item co-occurrence patterns exposed by item ID within sessions, while ignoring what attracts users to engage with certain items is rich multi-modal information displayed on pages. Generally, the multi-modal information can be classified into two categories: descriptive information (e.g., item images and description text) and numerical information (e.g., price). In this paper, we aim to improve session-based recommendation by modeling the above multi-modal information holistically. There are mainly three issues to reveal user intent from multi-modal information: (1) How to extract relevant semantics from heterogeneous descriptive information with different noise? (2) How to fuse these heterogeneous descriptive information to comprehensively infer user interests? (3) How to handle probabilistic influence of numerical information on user behaviors? To solve above issues, we propose a novel multi-modal session-based recommendation (MMSBR) that models both descriptive and numerical information under a unified framework. Specifically, a pseudo-modality contrastive learning is devised to enhance the representation learning of descriptive information. Afterwards, a hierarchical pivot transformer is presented to fuse heterogeneous descriptive information. Moreover, we represent numerical information with Gaussian distribution and design a Wasserstein self-attention to handle the probabilistic influence mode. Extensive experiments on three real-world datasets demonstrate the effectiveness of the proposed MMSBR. Further analysis also proves that our MMSBR can alleviate the cold-start problem in SBR effectively.
更多查看译文
关键词
Probabilistic logic,Behavioral sciences,Numerical models,Transformers,Semantics,Graph neural networks,Fuses,Hierarchical pivot transformer,multi-modal learning,probabilistic modeling,pseudo-modality contrastive learning,session-based recommendation
AI 理解论文
溯源树
样例
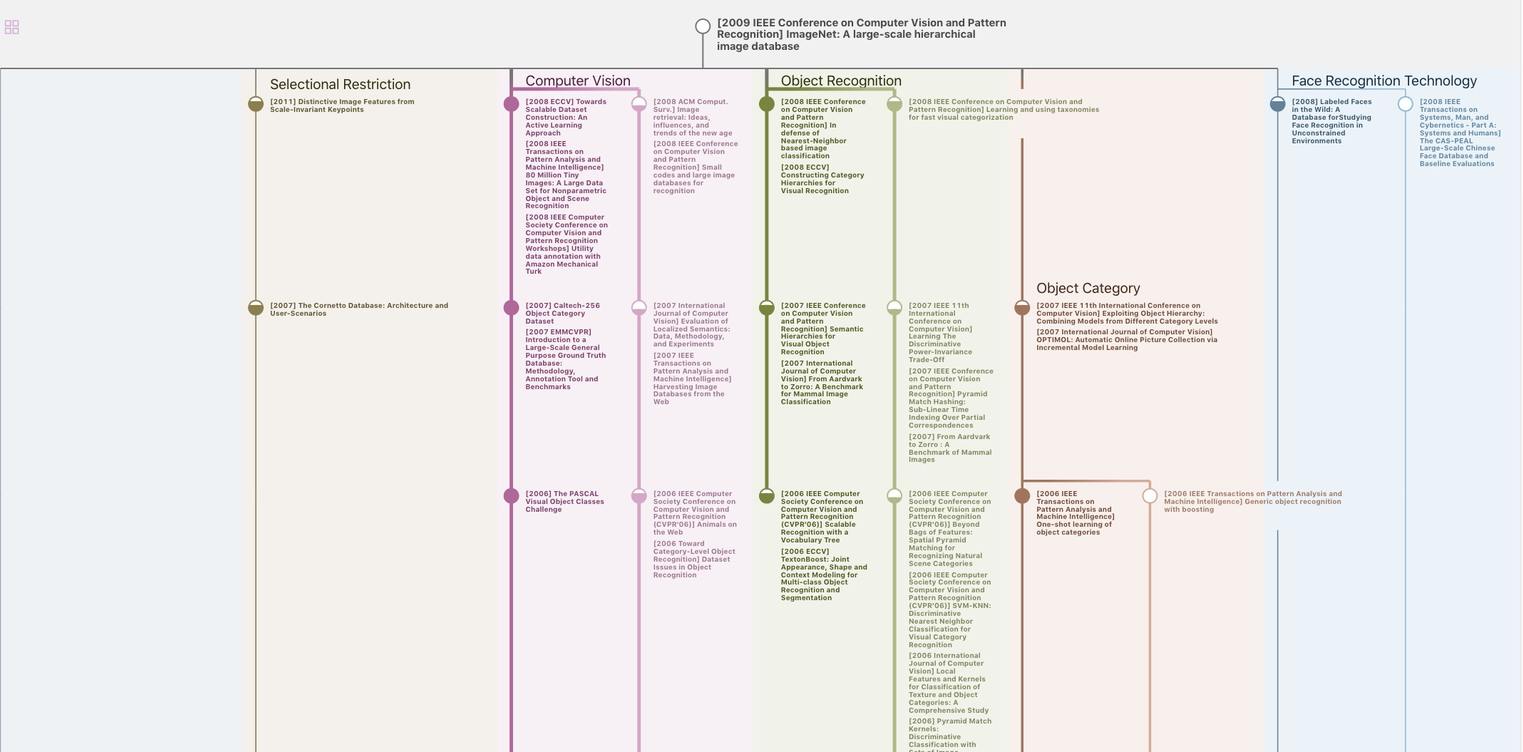
生成溯源树,研究论文发展脉络
Chat Paper
正在生成论文摘要