CauDR: A causality-inspired domain generalization framework for fundus-based diabetic retinopathy grading
Computers in Biology and Medicine(2024)
摘要
Diabetic retinopathy (DR) is the most common diabetic complication, which usually leads to retinal damage, vision loss, and even blindness. A computer-aided DR grading system has a significant impact on helping ophthalmologists with rapid screening and diagnosis. Recent advances in fundus photography have precipitated the development of novel retinal imaging cameras and their subsequent implementation in clinical practice. However, most deep learning-based algorithms for DR grading demonstrate limited generalization across domains. This inferior performance stems from variance in imaging protocols and devices inducing domain shifts. We posit that declining model performance between domains arises from learning spurious correlations in the data. Incorporating do-operations from causality analysis into model architectures may mitigate this issue and improve generalizability. Specifically, a novel universal structural causal model (SCM) was proposed to analyze spurious correlations in fundus imaging. Building on this, a causality-inspired diabetic retinopathy grading framework named CauDR was developed to eliminate spurious correlations and achieve more generalizable DR diagnostics. Furthermore, existing datasets were reorganized into 4DR benchmark for DG scenario. Results demonstrate the effectiveness and the state-of-the-art (SOTA) performance of CauDR. Diabetic retinopathy (DR) is the most common diabetic complication, which usually leads to retinal damage, vision loss, and even blindness. A computer-aided DR grading system has a significant impact on helping ophthalmologists with rapid screening and diagnosis. Recent advances in fundus photography have precipitated the development of novel retinal imaging cameras and their subsequent implementation in clinical practice. However, most deep learning-based algorithms for DR grading demonstrate limited generalization across domains. This inferior performance stems from variance in imaging protocols and devices inducing domain shifts. We posit that declining model performance between domains arises from learning spurious correlations in the data. Incorporating do-operations from causality analysis into model architectures may mitigate this issue and improve generalizability. Specifically, a novel universal structural causal model (SCM) was proposed to analyze spurious correlations in fundus imaging. Building on this, a causality-inspired diabetic retinopathy grading framework named CauDR was developed to eliminate spurious correlations and achieve more generalizable DR diagnostics. Furthermore, existing datasets were reorganized into 4DR benchmark for DG scenario. Results demonstrate the effectiveness and the state-of-the-art (SOTA) performance of CauDR.
更多查看译文
关键词
Diabetic retinopathy grading,Domain generalization,Causality-inspired model
AI 理解论文
溯源树
样例
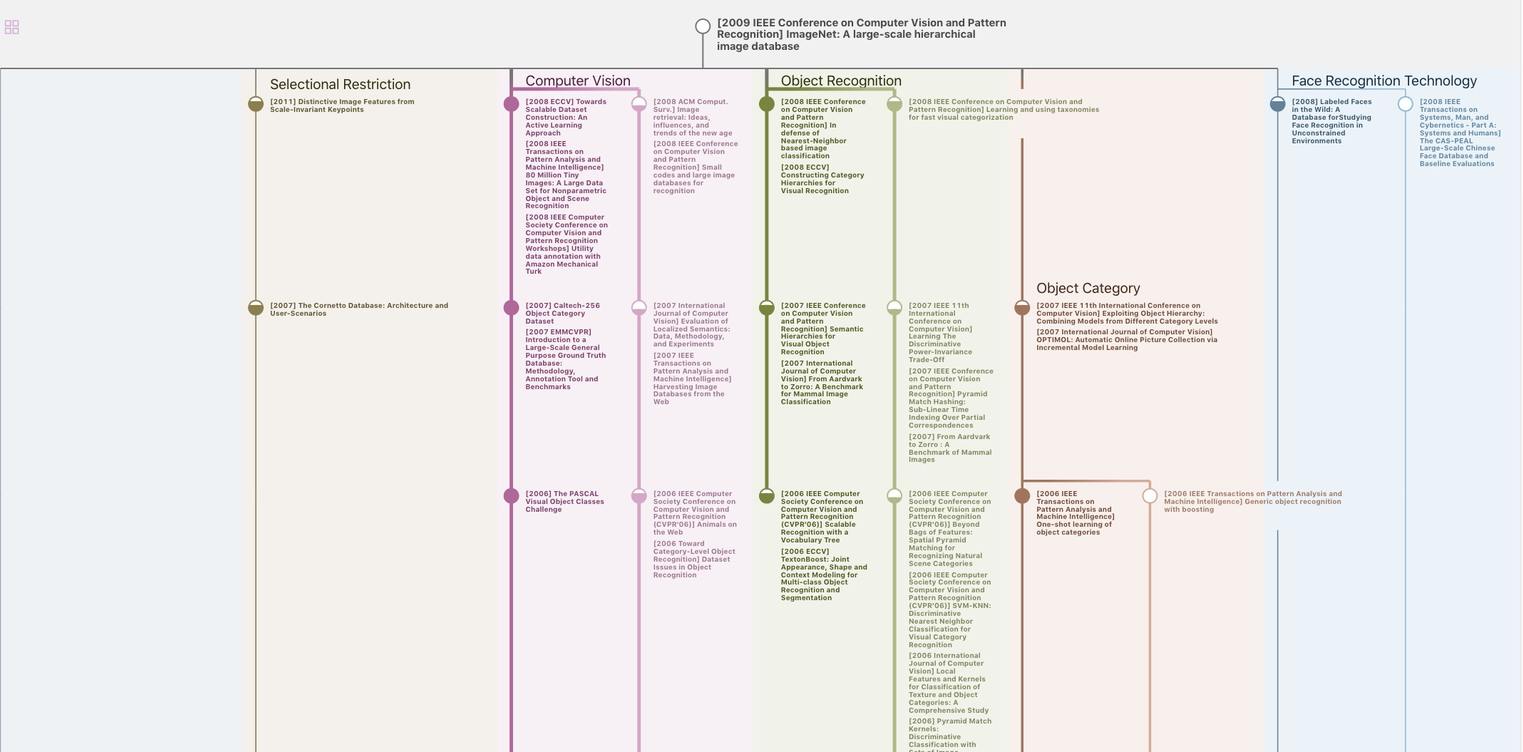
生成溯源树,研究论文发展脉络
Chat Paper
正在生成论文摘要