AdsorbML: a leap in efficiency for adsorption energy calculations using generalizable machine learning potentials
npj Computational Materials(2023)
摘要
Computational catalysis is playing an increasingly significant role in the design of catalysts across a wide range of applications. A common task for many computational methods is the need to accurately compute the adsorption energy for an adsorbate and a catalyst surface of interest. Traditionally, the identification of low-energy adsorbate-surface configurations relies on heuristic methods and researcher intuition. As the desire to perform high-throughput screening increases, it becomes challenging to use heuristics and intuition alone. In this paper, we demonstrate machine learning potentials can be leveraged to identify low-energy adsorbate-surface configurations more accurately and efficiently. Our algorithm provides a spectrum of trade-offs between accuracy and efficiency, with one balanced option finding the lowest energy configuration 87.36% of the time, while achieving a ~2000× speedup in computation. To standardize benchmarking, we introduce the Open Catalyst Dense dataset containing nearly 1000 diverse surfaces and ~100,000 unique configurations.
更多查看译文
关键词
adsorption energy calculations,generalizable machine learning potentials,machine learning
AI 理解论文
溯源树
样例
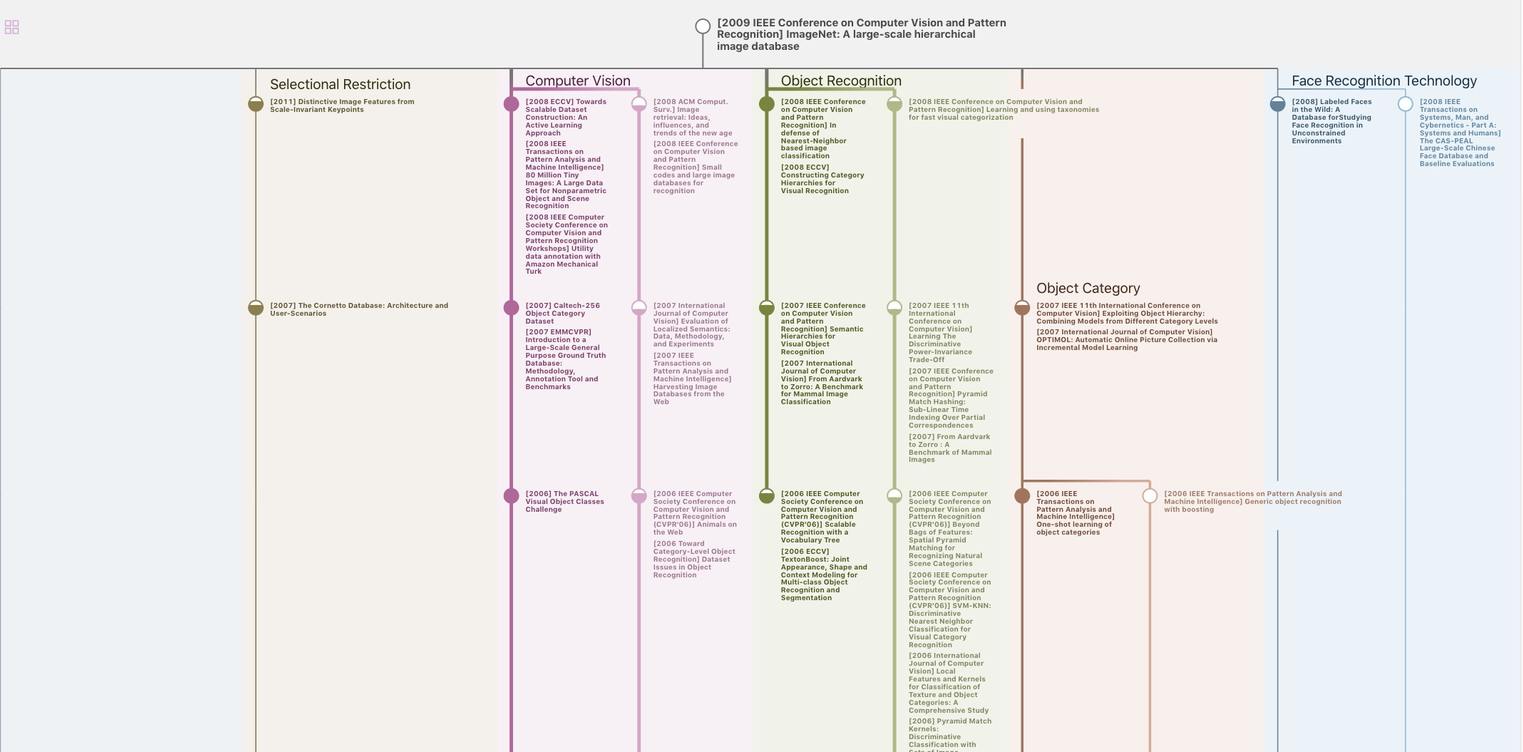
生成溯源树,研究论文发展脉络
Chat Paper
正在生成论文摘要