Leveraging Negative Signals with Self-Attention for Sequential Music Recommendation
CoRR(2023)
摘要
Music streaming services heavily rely on their recommendation engines to
continuously provide content to their consumers. Sequential recommendation
consequently has seen considerable attention in current literature, where state
of the art approaches focus on self-attentive models leveraging contextual
information such as long and short-term user history and item features;
however, most of these studies focus on long-form content domains (retail,
movie, etc.) rather than short-form, such as music. Additionally, many do not
explore incorporating negative session-level feedback during training. In this
study, we investigate the use of transformer-based self-attentive architectures
to learn implicit session-level information for sequential music
recommendation. We additionally propose a contrastive learning task to
incorporate negative feedback (e.g skipped tracks) to promote positive hits and
penalize negative hits. This task is formulated as a simple loss term that can
be incorporated into a variety of deep learning architectures for sequential
recommendation. Our experiments show that this results in consistent
performance gains over the baseline architectures ignoring negative user
feedback.
更多查看译文
关键词
sequential music,negative signals,self-attention
AI 理解论文
溯源树
样例
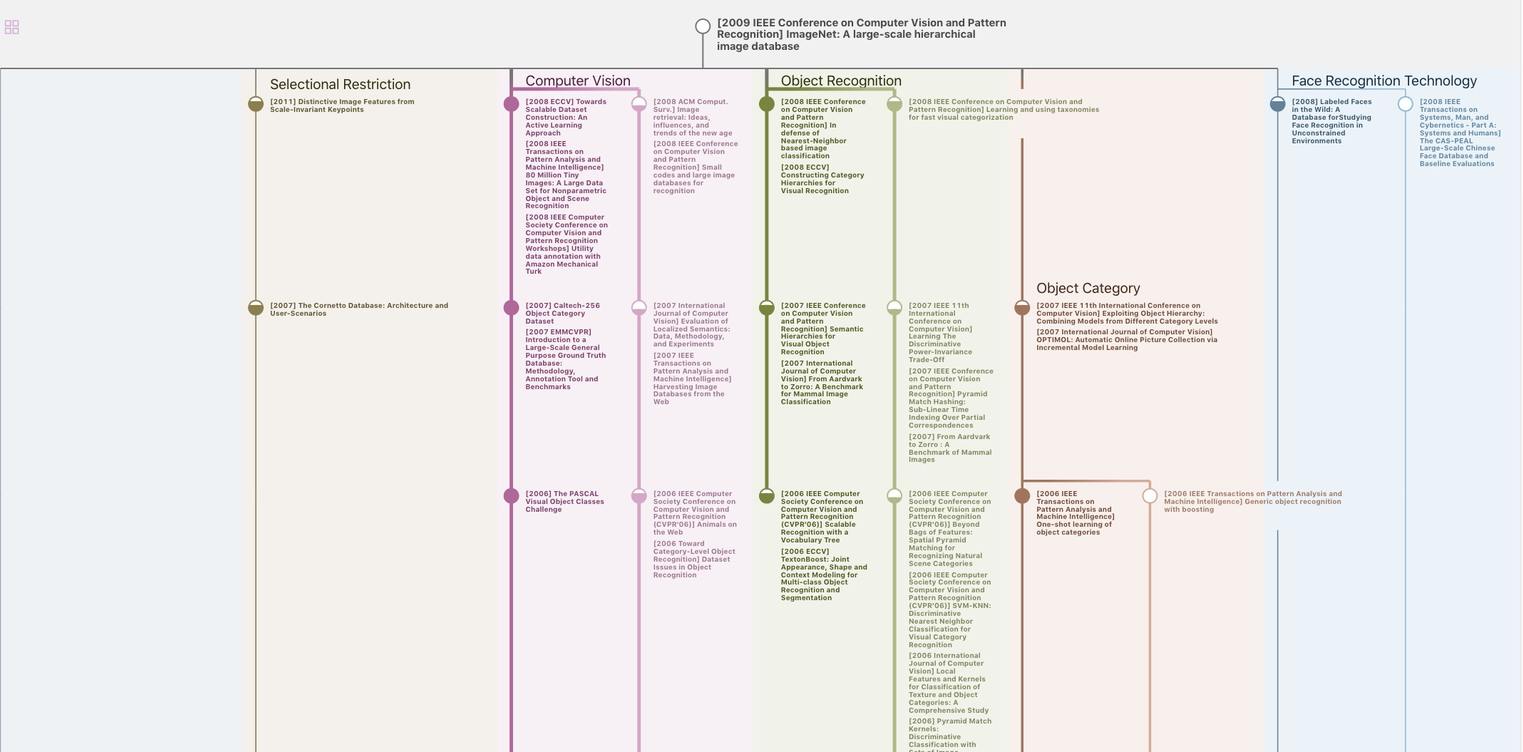
生成溯源树,研究论文发展脉络
Chat Paper
正在生成论文摘要