WeNet: Configurable Neural Network with Dynamic Weight-Enabling for Efficient Inference
2023 IEEE/ACM International Symposium on Low Power Electronics and Design (ISLPED)(2023)
摘要
Deep Neural Networks (DNN) are widely deployed in resource-limited edge devices. Due to the limitation of computational resources, it is important to meet the timing and energy constraints while maintaining a high level of accuracy. To deploy the same DNN model on different edge devices, one challenge is to train a dynamic neural network with the flexibility of balancing the trade-off between accuracy and efficiency at runtime. In this paper, we present a novel methodology, dynamic Weight-enabling Network (WeNet), where the weights of neural network can be dynamically enabled or disabled to switch between different sub-networks, so that we are able to balance the trade-off between inference time, energy consumption and model accuracy. We extend the methodology to convolutional layers using group convolution and channel shuffling. We also propose a design space exploration approach to search for the optimal sub-network for different scenarios. We thoroughly evaluate our methodology using a number of DNN architectures on different hardware platforms, showing that WeNet provides a large number of energy-efficient operation modes, 73.2 % of which provide better accuracy-efficiency trade-off compared to other methodologies.
更多查看译文
关键词
Deep learning, Energy-efficient application, Dynamic neural network
AI 理解论文
溯源树
样例
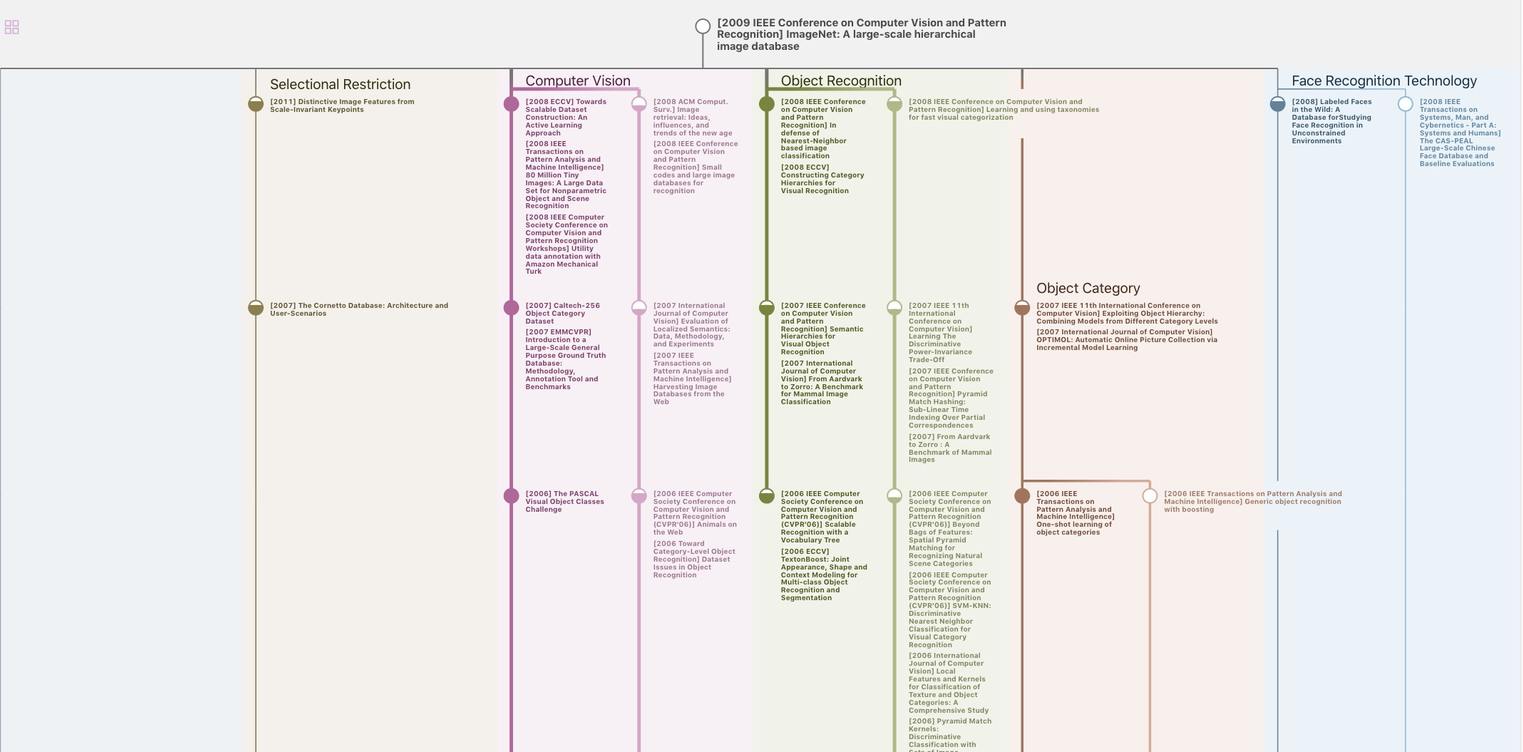
生成溯源树,研究论文发展脉络
Chat Paper
正在生成论文摘要