Cybersecurity and Privacy Attacks Detection in IoT Networks with Improved Data Engineering and Machine Learning Methods
2023 IEEE Ninth International Conference on Big Data Computing Service and Applications (BigDataService)(2023)
摘要
Presently, the global proliferation of Internet of Things (IoT) devices and applications has accelerated owing to their advantages in enhancing the business and industrial environment, as well as the daily lives of individuals. Nevertheless, IoT devices are vulnerable to malevolent network traffic, which can lead to undesirable outcomes and disrupt the operation of IoT devices. Consequently, it is imperative to devise a screening method for network traffic to identify and categorize malicious activity and reduce its harmful effects. In this regard, the present study proposes a framework and thoroughly compares single and ensemble machine learning models that are using predictive analytics to detect and classify network activity in an IoT network. Specifically, our framework and models distinguish between normal and anomalous network activity. Furthermore, network traffic is classified into eight categories, normal, denial of service (DoS) attack, Scan attack, Data Type Probing, Malicious Operation, Malicious Control, Spying attack and Wrong Setup based attack. The special focus of this paper is in identifying privacy related attacks in comparison with main cybersecurity related ones. Several supervised machine learning models have been herein implemented to characterize their performance in detecting and classifying network activities for IoT Networks based on the ensemble learning framework as well as on the single learning machines framework. Moreover, classifiers based on the statistical learning theory framework are herein involved too, including hybrid Bayesian theory models, decision trees (DT) and the ordinal Learning Modelling approach. The statistical pattern recognition based models as well as all supervised learning machines models have been evaluated on a broad benchmark dataset for IoT attacks. Besides, an improved data engineering feature processing model has been applied to the dataset under consideration to improve the accuracy of all models under comparison.
更多查看译文
关键词
Cybersecurity Attacks Detection,Privacy Attacks Detection,IoT Networks,Machine Learning,Data Engineering
AI 理解论文
溯源树
样例
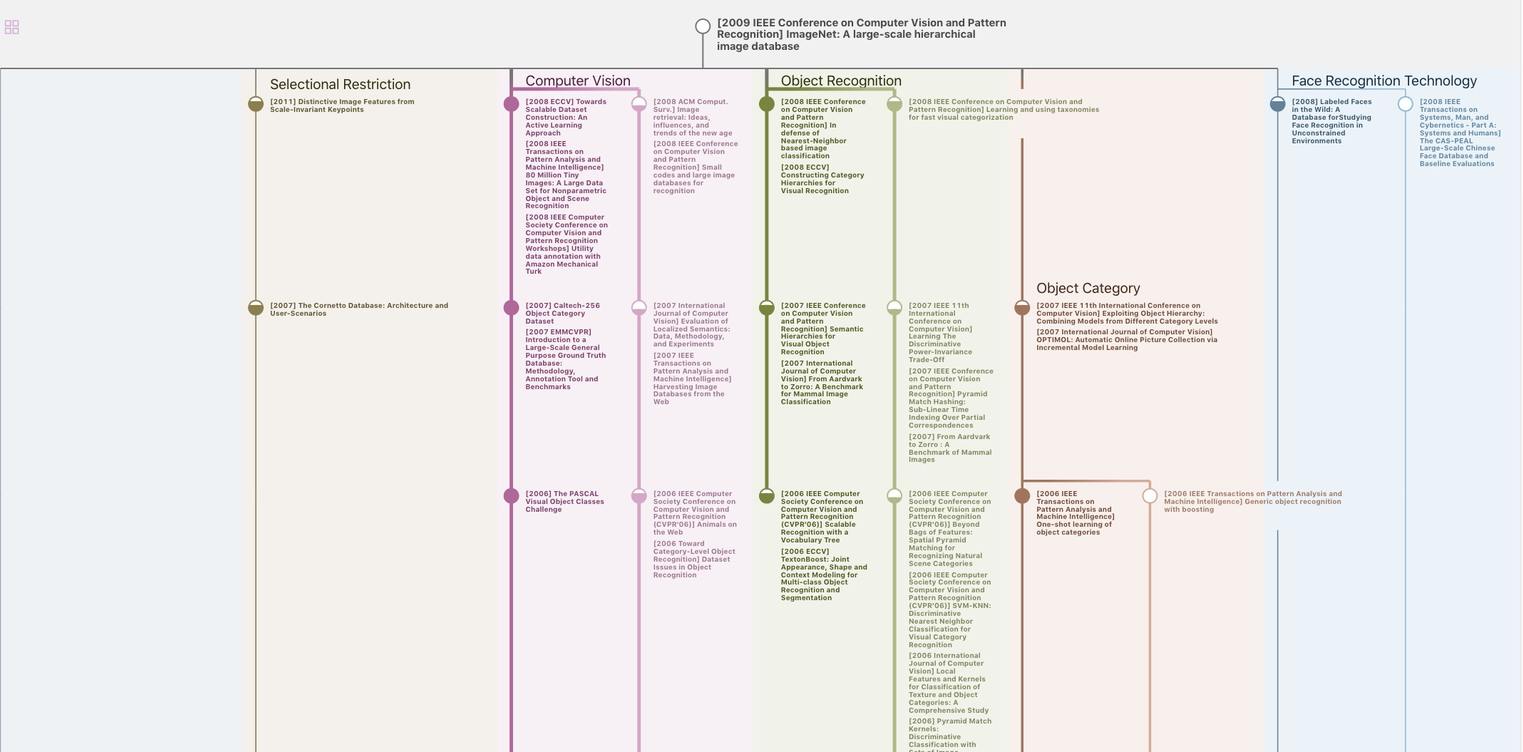
生成溯源树,研究论文发展脉络
Chat Paper
正在生成论文摘要