DSPIMM: A Fully Digital SParse In-Memory Matrix Vector Multiplier for Communication Applications
2023 60th ACM/IEEE Design Automation Conference (DAC)(2023)
摘要
Channel decoders are key computing modules in wired/wireless communication systems. Recently neural network (NN)-based decoders have shown their promising error-correcting performance because of their end-to-end learning capability. However, compared with the traditional approaches, the emerging neural belief propagation (NBP) solution suffers higher storage and computational complexity, limiting its hardware performance. To address this challenge and develop a channel decoder that can achieve high decoding performance and hardware performance simultaneously, in this paper we take a first step towards exploring SRAM-based in-memory computing for efficient NBP channel decoding. We first analyze the unique sparsity pattern in the NBP processing, and then propose an efficient and fully Digital Sparse In-Memory Matrix vector Multiplier (DSPIMM) computing platform. Extensive experiments demonstrate that our proposed DSPIMM achieves significantly higher energy efficiency and throughput than the state-of-the-art counterparts.
更多查看译文
关键词
Sparsity,In-Memory-Computing,SRAM,MAC,Neural Decoder
AI 理解论文
溯源树
样例
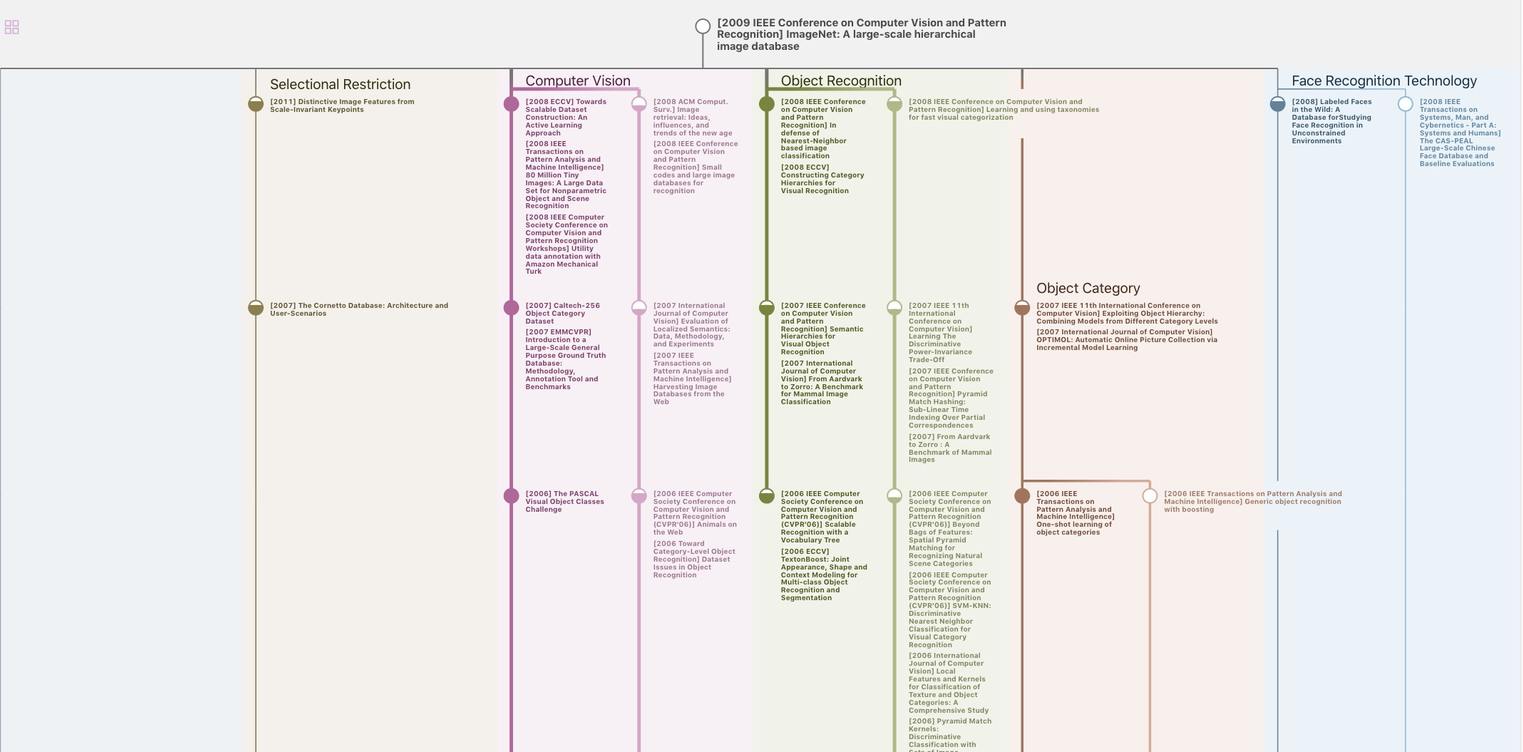
生成溯源树,研究论文发展脉络
Chat Paper
正在生成论文摘要