Revisiting Energy Based Models as Policies: Ranking Noise Contrastive Estimation and Interpolating Energy Models
CoRR(2023)
摘要
A crucial design decision for any robot learning pipeline is the choice of policy representation: what type of model should be used to generate the next set of robot actions? Owing to the inherent multi-modal nature of many robotic tasks, combined with the recent successes in generative modeling, researchers have turned to state-of-the-art probabilistic models such as diffusion models for policy representation. In this work, we revisit the choice of energy-based models (EBM) as a policy class. We show that the prevailing folklore -- that energy models in high dimensional continuous spaces are impractical to train -- is false. We develop a practical training objective and algorithm for energy models which combines several key ingredients: (i) ranking noise contrastive estimation (R-NCE), (ii) learnable negative samplers, and (iii) non-adversarial joint training. We prove that our proposed objective function is asymptotically consistent and quantify its limiting variance. On the other hand, we show that the Implicit Behavior Cloning (IBC) objective is actually biased even at the population level, providing a mathematical explanation for the poor performance of IBC trained energy policies in several independent follow-up works. We further extend our algorithm to learn a continuous stochastic process that bridges noise and data, modeling this process with a family of EBMs indexed by scale variable. In doing so, we demonstrate that the core idea behind recent progress in generative modeling is actually compatible with EBMs. Altogether, our proposed training algorithms enable us to train energy-based models as policies which compete with -- and even outperform -- diffusion models and other state-of-the-art approaches in several challenging multi-modal benchmarks: obstacle avoidance path planning and contact-rich block pushing.
更多查看译文
关键词
energy based models,ranking noise contrastive
AI 理解论文
溯源树
样例
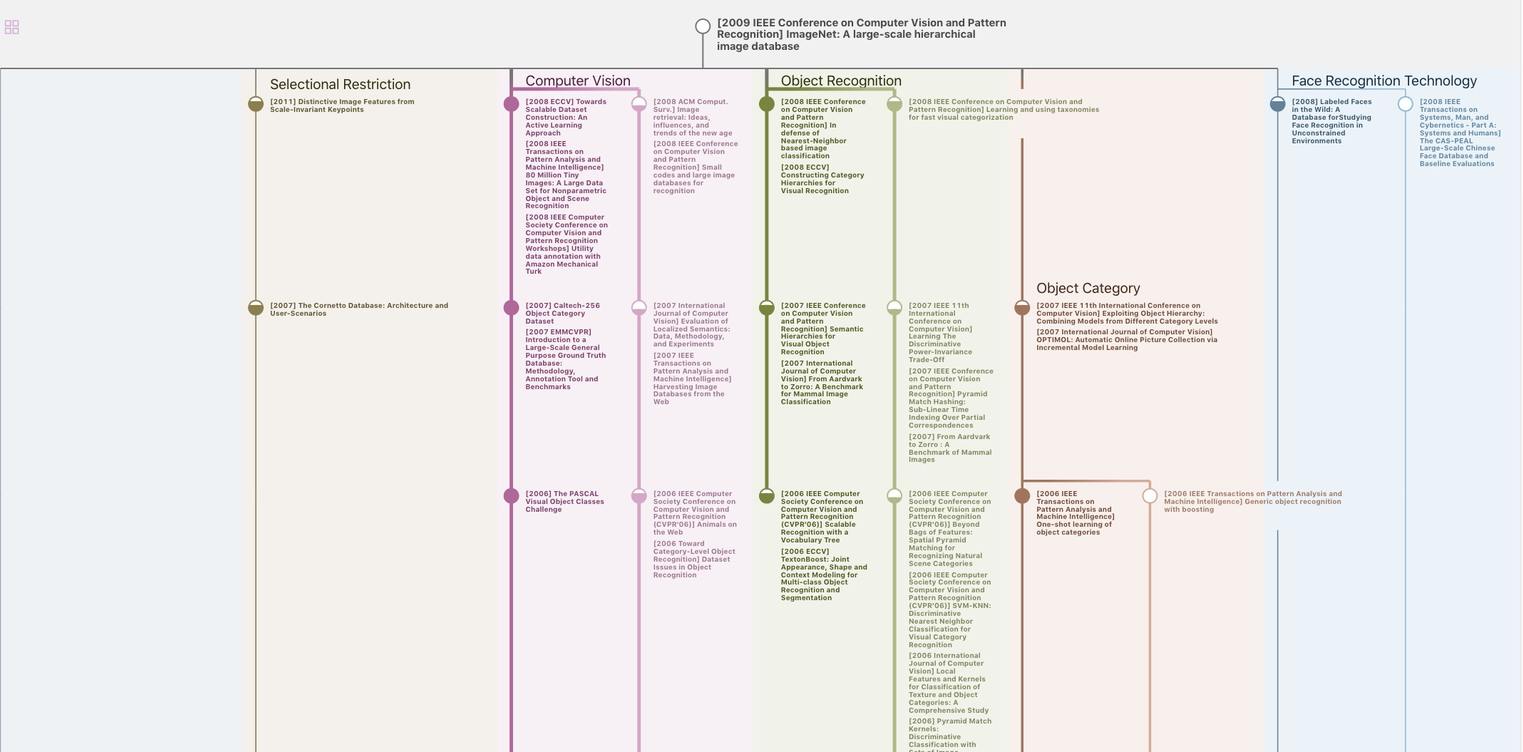
生成溯源树,研究论文发展脉络
Chat Paper
正在生成论文摘要