Protein intrinsically disordered region prediction by combining neural architecture search and multi-objective genetic algorithm
BMC biology(2023)
摘要
Background Intrinsically disordered regions (IDRs) are widely distributed in proteins and related to many important biological functions. Accurately identifying IDRs is of great significance for protein structure and function analysis. Because the long disordered regions (LDRs) and short disordered regions (SDRs) share different characteristics, the existing predictors fail to achieve better and more stable performance on datasets with different ratios between LDRs and SDRs. There are two main reasons. First, the existing predictors construct network structures based on their own experiences such as convolutional neural network (CNN) which is used to extract the feature of neighboring residues in protein, and long short-term memory (LSTM) is used to extract the long-distance dependencies feature of protein residues. But these networks cannot capture the hidden feature associated with the length-dependent between residues. Second, many algorithms based on deep learning have been proposed but the complementarity of the existing predictors is not fully explored and used. Results In this study, the neural architecture search (NAS) algorithm was employed to automatically construct the network structures so as to capture the hidden features in protein sequences. In order to stably predict both the LDRs and SDRs, the model constructed by NAS was combined with length-dependent models for capturing the unique features of SDRs or LDRs and general models for capturing the common features between LDRs and SDRs. A new predictor called IDP-Fusion was proposed. Conclusions Experimental results showed that IDP-Fusion can achieve more stable performance than the other existing predictors on independent test sets with different ratios between SDRs and LDRs.
更多查看译文
关键词
Intrinsically disordered regions (IDRs),Neural architecture search (NAS),Length-dependent models
AI 理解论文
溯源树
样例
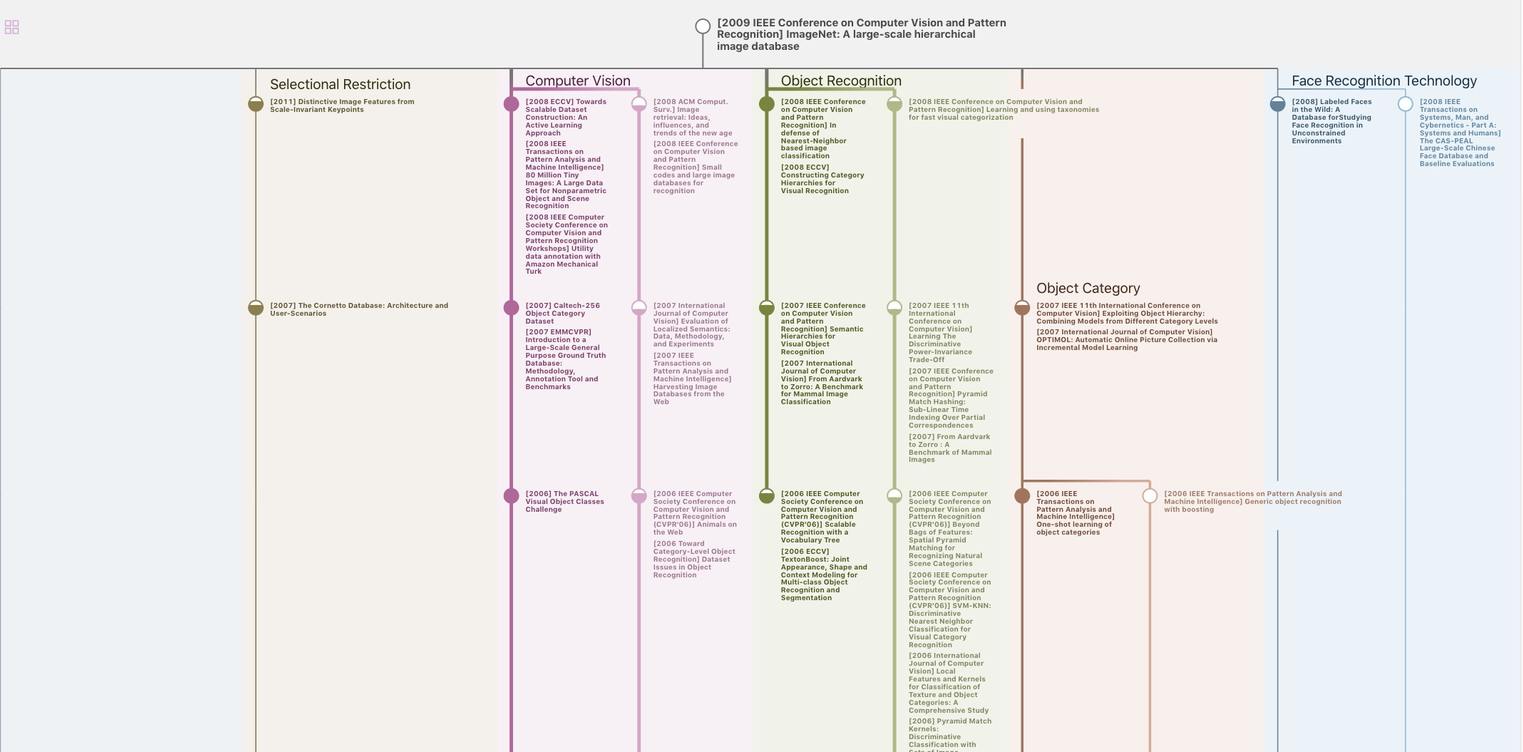
生成溯源树,研究论文发展脉络
Chat Paper
正在生成论文摘要