Machine Learning-Assisted Compact Modeling of W-Doped Indium Oxide Channel Transistor for Back-End-of-Line Applications
IEEE TRANSACTIONS ON ELECTRON DEVICES(2024)
摘要
Machine learning (ML)-assisted compact modeling framework is proposed for design-technology co-optimization (DTCO) of W-doped indium oxide (IWO) channel transistor. The IWO transistor is modeled based on experimentally calibrated technology computer-aided-design (TCAD) simulations to generate datasets for neural network (NN) training and testing. The fit ML model is simulated under various structural parameters (gate length, and width) and voltage conditions (gate voltage and drain voltage). On the one hand, two NNs are designed for current-voltage (I-V) and capacitance-voltage (C-V) predictions, and after training and evaluation, the Python-based NNs with trained weights and biases are ported into a compact model based on Verilog-A format. The accuracy of ML-assisted compact model is verified by comparing the results from SPICE and TCAD mixed-mode simulations for back-end-of-line (BEOL) applications such as 3-D inverter and 2T gain cell memory. This ML framework introduces a new approach for the DTCO of emerging devices, providing an efficient and accurate method to model and optimize their behavior while significantly reducing design iterations and time consumption.
更多查看译文
关键词
Index Terms-Compact model,emerging device,machine learning (ML),neural network (NN),oxide channel transistor,SPICE
AI 理解论文
溯源树
样例
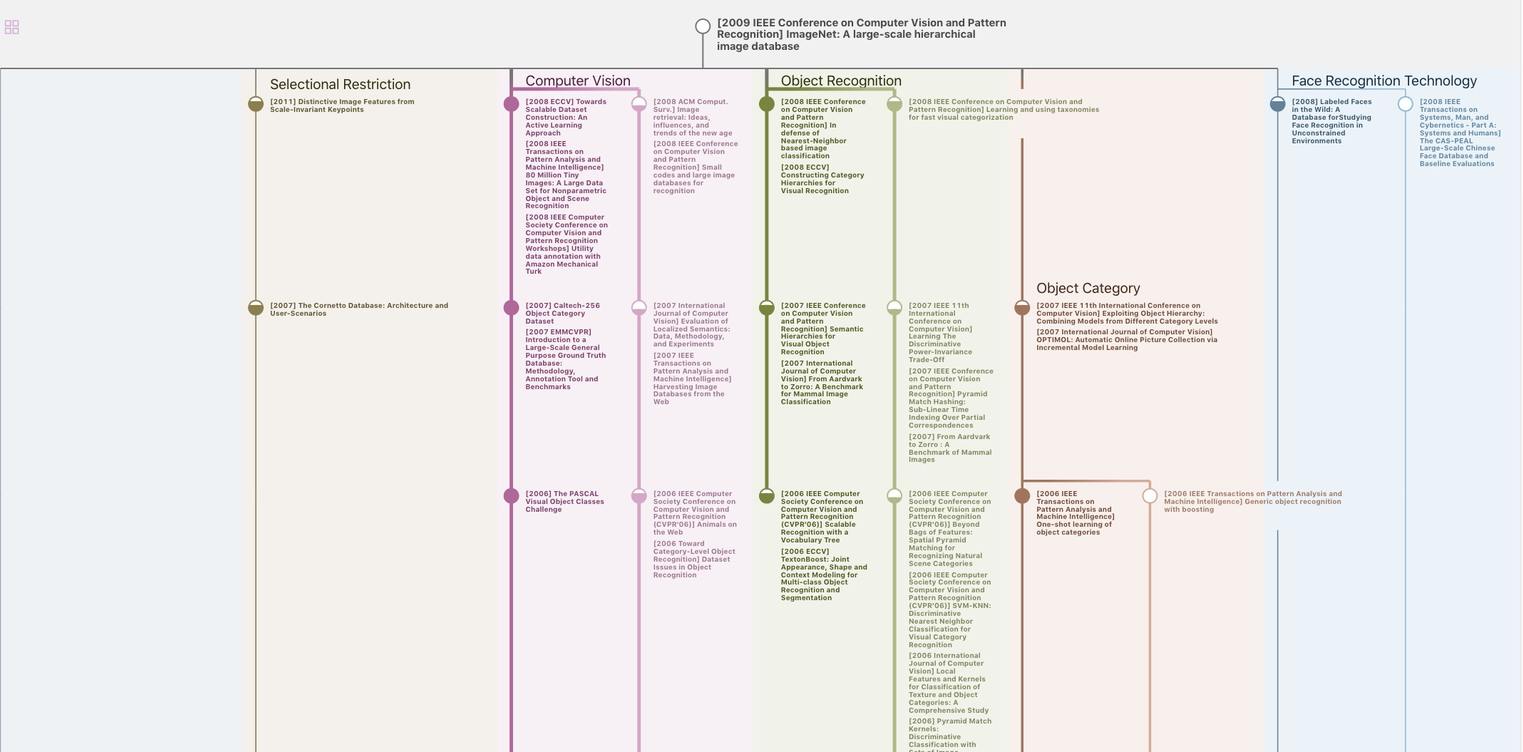
生成溯源树,研究论文发展脉络
Chat Paper
正在生成论文摘要