Large Deviations Anomaly Detection (LAD) for collection of multivariate time series data: Applications to COVID-19 data
JOURNAL OF COMPUTATIONAL SCIENCE(2023)
摘要
Time series anomaly detection is frequently used to identify extreme behaviors within a single time series. Identifying extreme trends in relation to a collection of other time series, on the other hand, is frequently of significant interest, such as in public health policy, social justice, and pandemic propagation. Using concepts from large deviations theory, we propose an algorithm that can scale to large collections of time series data. This paper expands on the LAD algorithm presented in Guggilam et al. (2022). The proposed algorithm is an online anomaly detection method for identifying anomalies in a collection of multivariate time series that takes advantage of the algorithm's ability to scale to high-dimensional data. We show how the proposed Large Deviations Anomaly Detection (LAD) algorithm can be used to identify regions with anomalous trends in COVID-19 cases, deaths, biweekly growth rates, vaccinations, and fatality rates. Several of the observed anomalous trends are associated with regions that have demonstrated poor response to the COVID pandemic.
更多查看译文
关键词
Large deviations,Anomaly detection,High-dimensional data,Multivariate time series,Time series database
AI 理解论文
溯源树
样例
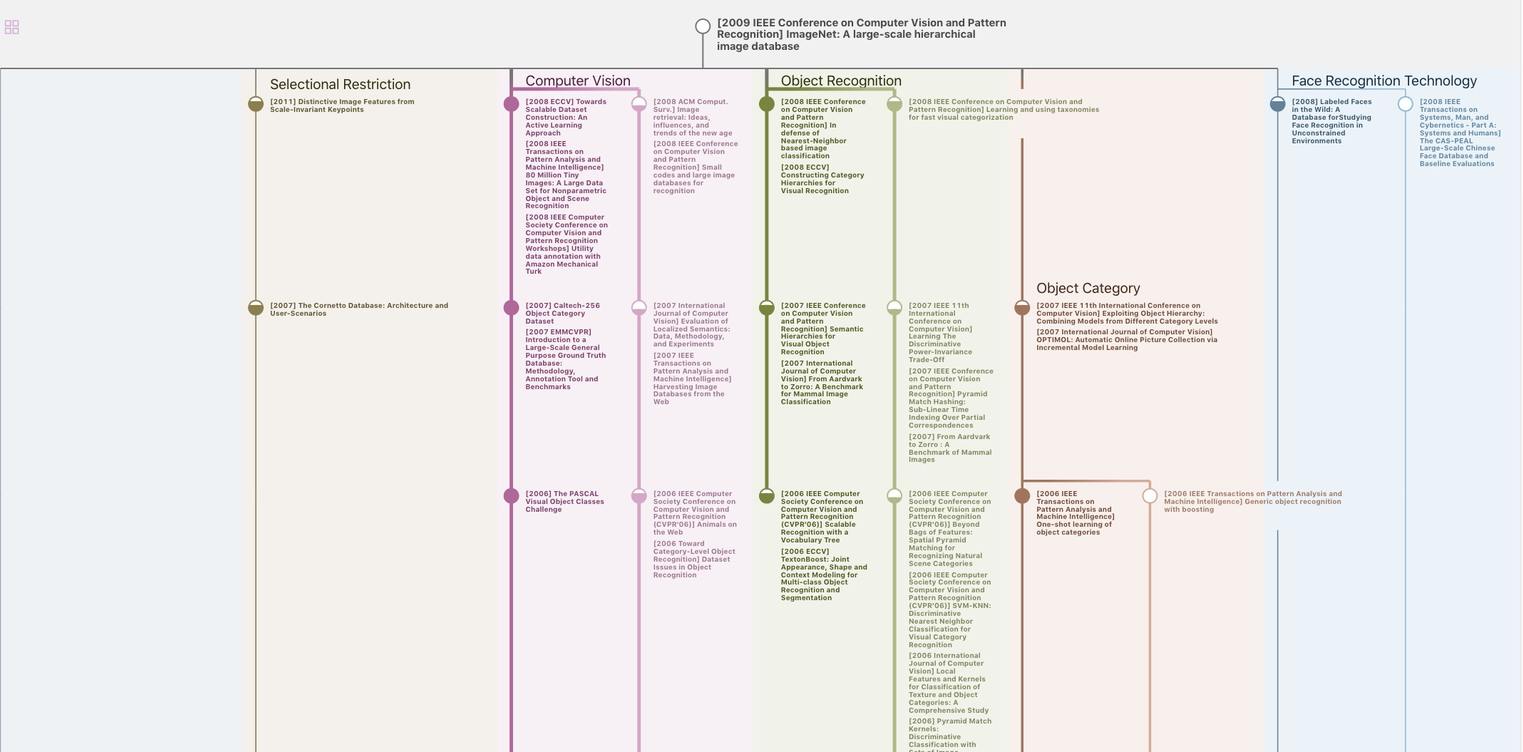
生成溯源树,研究论文发展脉络
Chat Paper
正在生成论文摘要