An efficient long-text semantic retrieval approach via utilizing presentation learning on short-text
COMPLEX & INTELLIGENT SYSTEMS(2024)
摘要
Although the short-text retrieval model by BERT achieves significant performance improvement, research on the efficiency and performance of long-text retrieval still faces challenges. Therefore, this study proposes an efficient long-text retrieval model based on BERT (called LTR-BERT). This model achieves speed improvement while retaining most of the long-text retrieval performance. In particular, The LTR-BERT model is trained by using the relevance between short texts. Then, the long text is segmented and stored off-line. In the retrieval stage, only the coding of the query and the matching scores are calculated, which speeds up the retrieval. Moreover, a query expansion strategy is designed to enhance the representation of the original query and reserve the encoding region for the query. It is beneficial for learning missing information in the representation stage. The interaction mechanism without training parameters takes into account the local semantic details and the whole relevance to ensure the accuracy of retrieval and further shorten the response time. Experiments are carried out on MS MARCO Document Ranking dataset, which is specially designed for long-text retrieval. Compared with the interaction-focused semantic matching method by BERT-CLS, the MRR@10 values of the proposed LTR-BERT method are increased by 2.74%. Moreover, the number of documents processed per millisecond increased by 333 times.
更多查看译文
关键词
Neural information retrieval,Long-text similarity,Pretrained language model,Efficiency
AI 理解论文
溯源树
样例
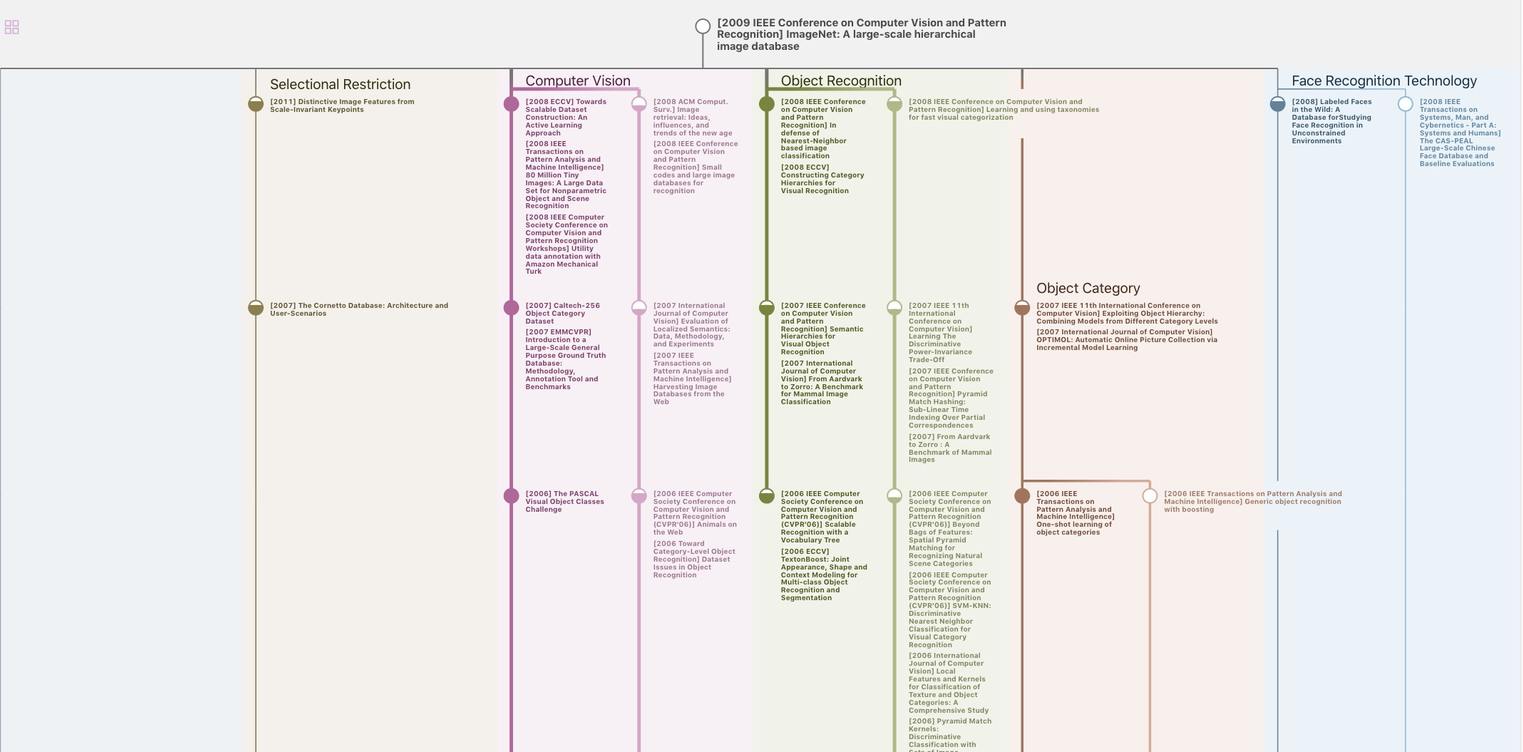
生成溯源树,研究论文发展脉络
Chat Paper
正在生成论文摘要