FactSheets for Hardware-Aware AI Models: A Case Study of Analog In Memory Computing AI Models
2023 IEEE International Conference on Software Services Engineering (SSE)(2023)
摘要
In the last few years, documenting and tracking the lineage of AI models has emerged as a important research area that can help to improve the transparency, traceability and overall effectiveness of a model when it is used or deployed by an entity that did not create it. This is a crucial step towards responsible AI in the services computing paradigm especially as AI-enabled software service engineering is becoming more prevalent and mainstream. Multiple documentation methods have been proposed and their adoption has slowly begun, but these methods tend to focus on the data science aspects of the model creation, such as the datasets used to design and train the model, the neural network structure of the model, the F1 score, the modal bias, etc. When adapted to the emerging AI hardware accelerators field of analog in-memory computing (IMC), additional documentation requirements need to be considered. Analog IMC accelerators offer increased area and power efficiency, which are paramount in IOT and edge resource-constrained environments. We use the AI FactSheets (FS) 360 documentation methodology to understand and evaluate the documentation needs in this emerging domain. To do so, we interviewed 12 participants who represent various roles throughout the lifecycle of designing, training, evaluating, deploying and consuming an analog-aware AI model. From these interviews we capture these roles' documentation and collaborative needs, develop FactSheets to meet those needs, and evaluate the quality of completed FactSheets. We show that the FactSheets methodology can be applied to Analog AI models to successfully create meaningful documentation that is suitable across multiple roles and a key step towards responsible AI models.
更多查看译文
关键词
Analog AI,In-memory Computing,AI documentation,AI Transparency,AI lifecycle,Responsible AI
AI 理解论文
溯源树
样例
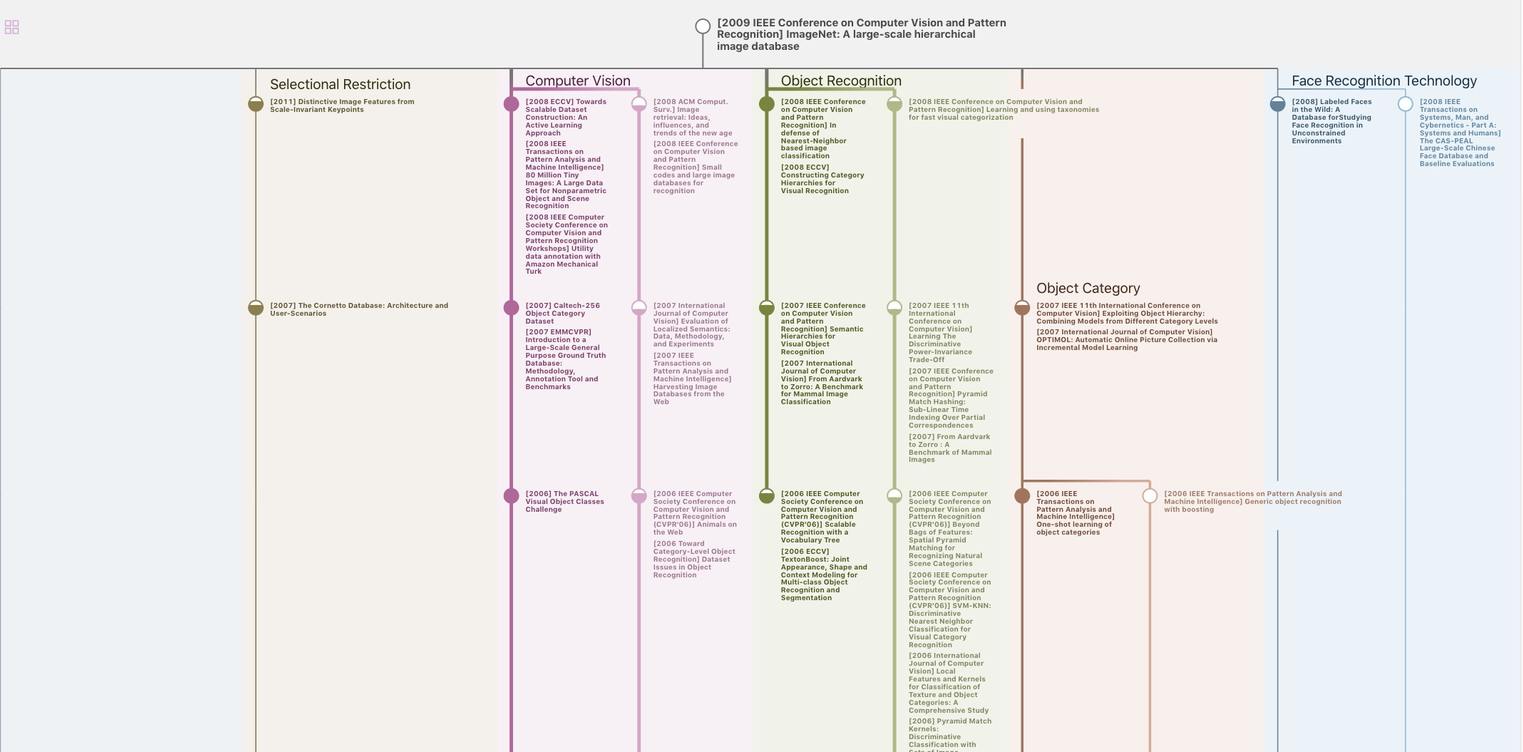
生成溯源树,研究论文发展脉络
Chat Paper
正在生成论文摘要