Addressing Underestimation Bias in CBR Through Case-Base Maintenance.
ICCBR(2023)
摘要
The knowledge containers perspective on CBR suggests that bias and fairness issues can be addressed in a number of different ways. In this paper we assess the use of case-base maintenance to ensure fairness. We present FairCBM, a strategy for removing cases that are causing biased classifications. We evaluate this strategy on five different datasets and show that it is effective for ensuring fairness with minimal impact on classification accuracy. FairCBM is also evaluated against an alternative metric learning strategy (similarity knowledge container); the evaluation shows that both strategies are equally effective. FairCBM has the benefit that it is quite transparent; by comparison the metric learning strategy is more opaque.
更多查看译文
关键词
underestimation bias,maintenance,case-base
AI 理解论文
溯源树
样例
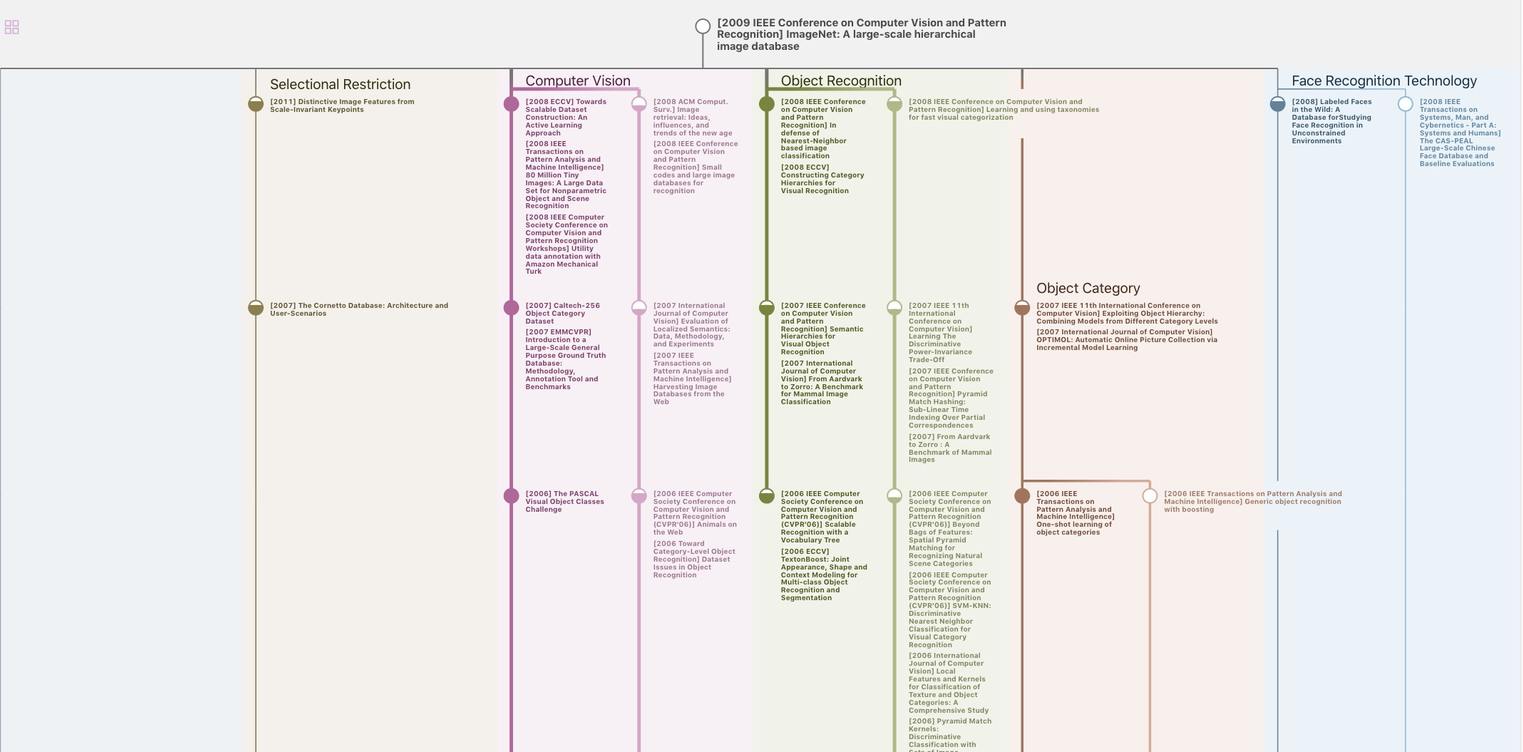
生成溯源树,研究论文发展脉络
Chat Paper
正在生成论文摘要