The Expressive Power of Tuning Only the Normalization Layers.
COLT(2023)
摘要
Feature normalization transforms such as Batch and Layer-Normalization have become indispensable ingredients of state-of-the-art deep neural networks. Recent studies on fine-tuning large pretrained models indicate that just tuning the parameters of these affine transforms can achieve high accuracy for downstream tasks. These findings open the questions about the expressive power of tuning the normalization layers of frozen networks. In this work, we take the first step towards this question and show that for random ReLU networks, fine-tuning only its normalization layers can reconstruct any target network that is $O(\sqrt{\text{width}})$ times smaller. We show that this holds even for randomly sparsified networks, under sufficient overparameterization, in agreement with prior empirical work.
更多查看译文
关键词
expressive power
AI 理解论文
溯源树
样例
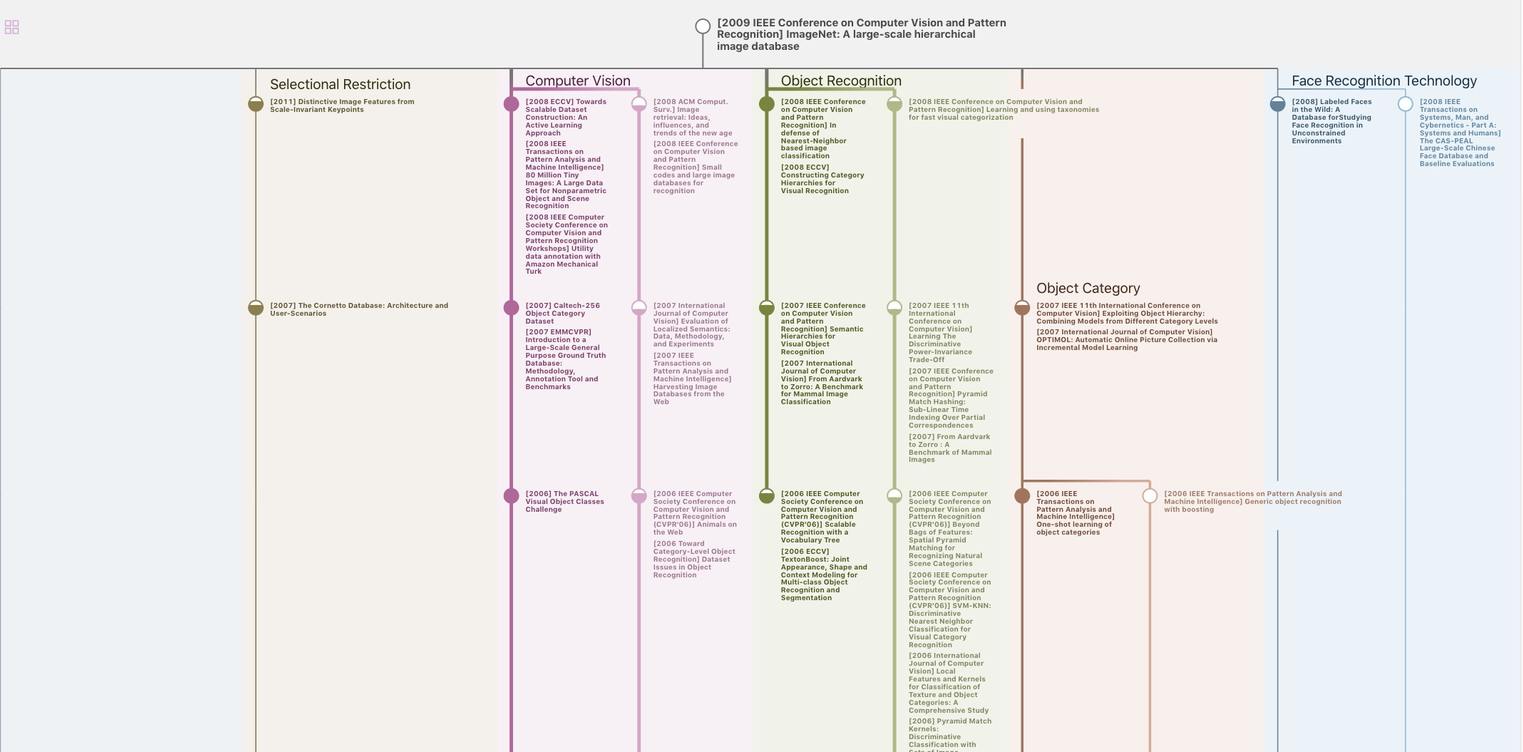
生成溯源树,研究论文发展脉络
Chat Paper
正在生成论文摘要