Mitigating Discriminatory Biases in Success Prediction Models for Venture Capitals
SDS(2023)
摘要
The fairness of machine learning-based decision support systems has become a critical issue, also in the field of predicting the success of venture capital investment startups. Inappropriate allocation of venture capital, fueled by discriminatory biases, can lead to missed investment opportunities and poor investment decisions. Despite numerous studies that have addressed the prevalence of biases in venture capital allocation and decision support models, few have addressed the importance of incorporating fairness into the modeling process. In this study, we leverage invariant feature representation learning to develop a startup success prediction model using Crunchbase data, while satisfying group fairness. Our results show that discriminatory bias can be significantly reduced with minimal impact on model performance. Additionally, we demonstrate the versatility of our approach by mitigating multiple biases simultaneously. This work highlights the significance of addressing fairness in decisionsupport models to ensure equitable outcomes in venture capital investments.
更多查看译文
关键词
model fairness, gradient reversal, venture capital, success modeling
AI 理解论文
溯源树
样例
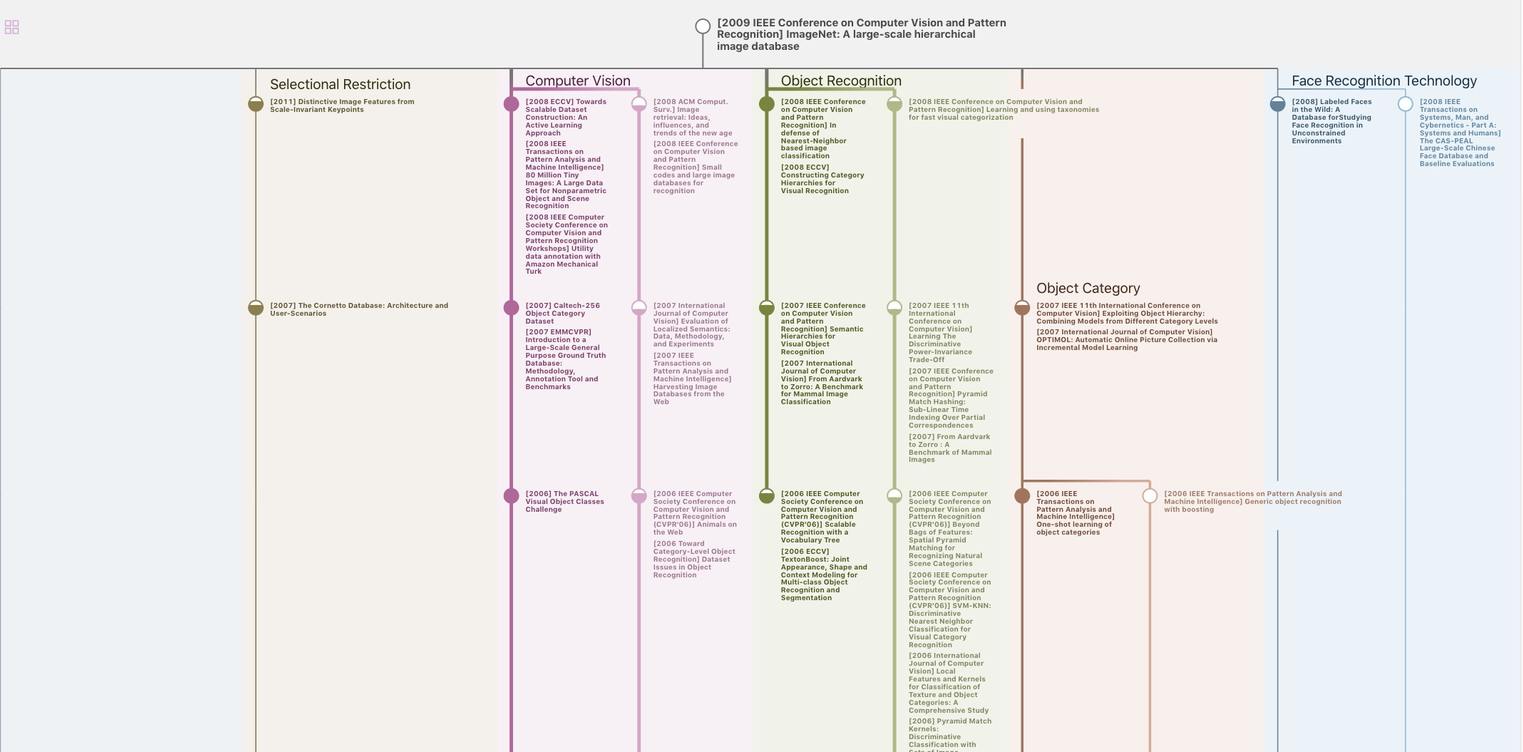
生成溯源树,研究论文发展脉络
Chat Paper
正在生成论文摘要