Agnostic Label-Only Membership Inference Attack.
NSS(2023)
摘要
In recent years we are witnessing the diffusion of AI systems based on powerful Machine Learning models which find application in many critical contexts such as medicine and financial market. In such contexts, it is important to design Trustworthy AI systems while guaranteeing privacy protection. However, some attacks on the privacy of Machine Learning models have been designed to show the threats of exposing such models. Membership Inference is one of the simplest privacy threats faced by Machine Learning models. It is based on the assumption that an adversary, observing the confidence of the model prediction, can infer whether a particular record was used for training the classifier. A variant, called Label-Only attack, exploits the adversary’s knowledge of the training data statistics to infer the record membership without accessing the confidence score of the prediction. In this paper, we propose a variant of the Label-Only attack, called Aloa, which estimates the prediction confidence exploiting a mechanism that is completely agnostic to the input data distributions. In fact, it requires neither statistical knowledge of the data nor the type of variables. Experimental results show better performance of our attack with respect to the competitors.
更多查看译文
关键词
membership,label-only
AI 理解论文
溯源树
样例
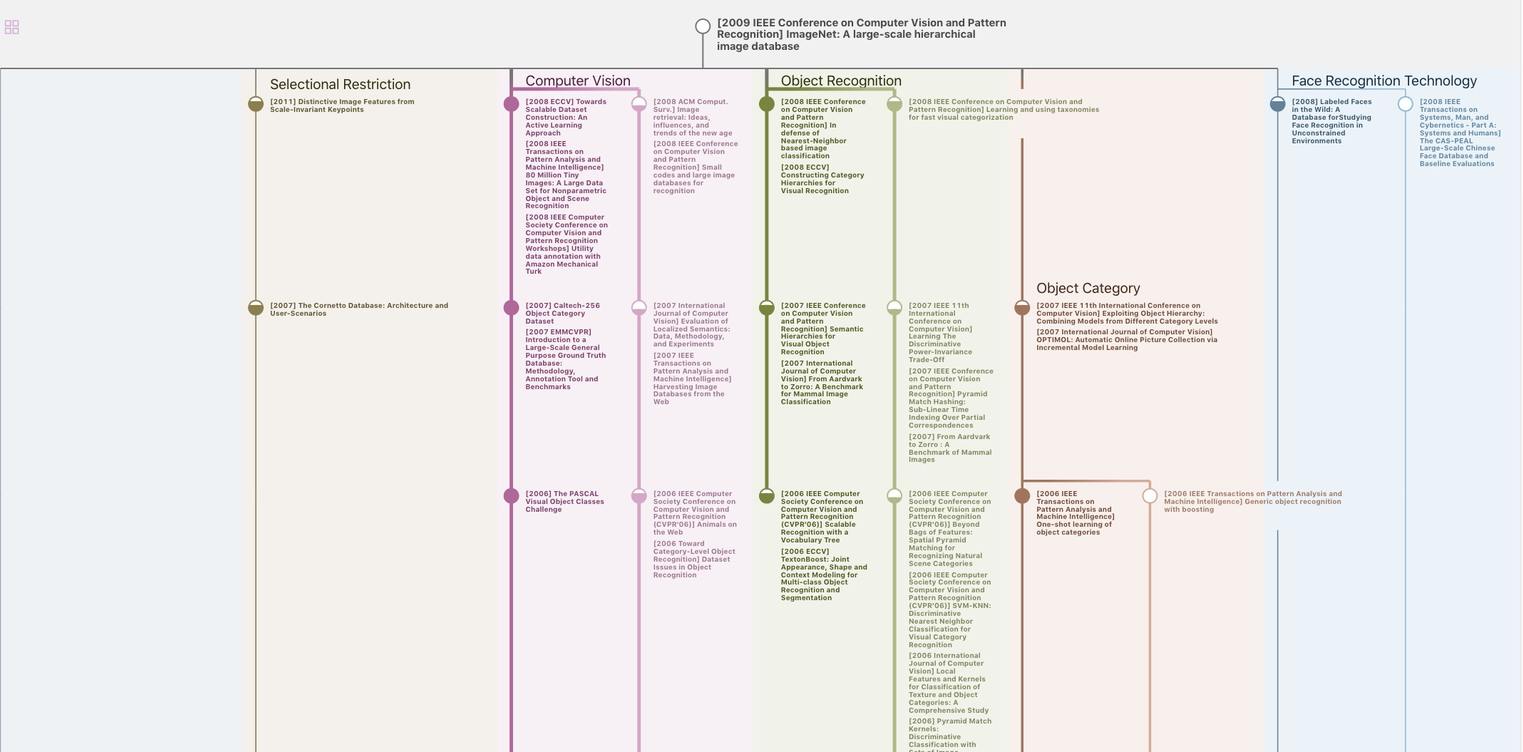
生成溯源树,研究论文发展脉络
Chat Paper
正在生成论文摘要