A Novel Scalable Framework to Reconstruct Vehicular Trajectories From Unreliable GPS Datasets
IEEE Transactions on Intelligent Transportation Systems(2023)
摘要
Vehicle trajectory data is paramount in many applications and research areas, such as vehicular networks and Intelligent Transportation Systems (ITS). However, data gathered from location acquisition devices generally contain positional errors that hinder its applicability, and therefore processing techniques are necessary to improve the quality of trajectory data. For instance, physical constraints of the road network that bounds the vehicles’ movement can be used to represent a trajectory better. Therefore, this paper proposes an efficient framework to reconstruct road-network constrained trajectories from GPS-based datasets. The framework employs novel processing algorithms and models to prepare even low sampled trajectories, which naturally present gaps, for real applications. Besides that, we present a novel real-world benchmark dataset to evaluate trajectory reconstruction and map-matching algorithms and perform extensive experimental evaluations using the new dataset and another one from the literature to compare the proposed framework to related work. The experimental results show that the proposed framework has a better time complexity and accuracy than the other methods in all evaluated scenarios.
更多查看译文
关键词
Index Terms-Vehicle trajectory, data mining, datasets, map-matching, trajectory reconstruction
AI 理解论文
溯源树
样例
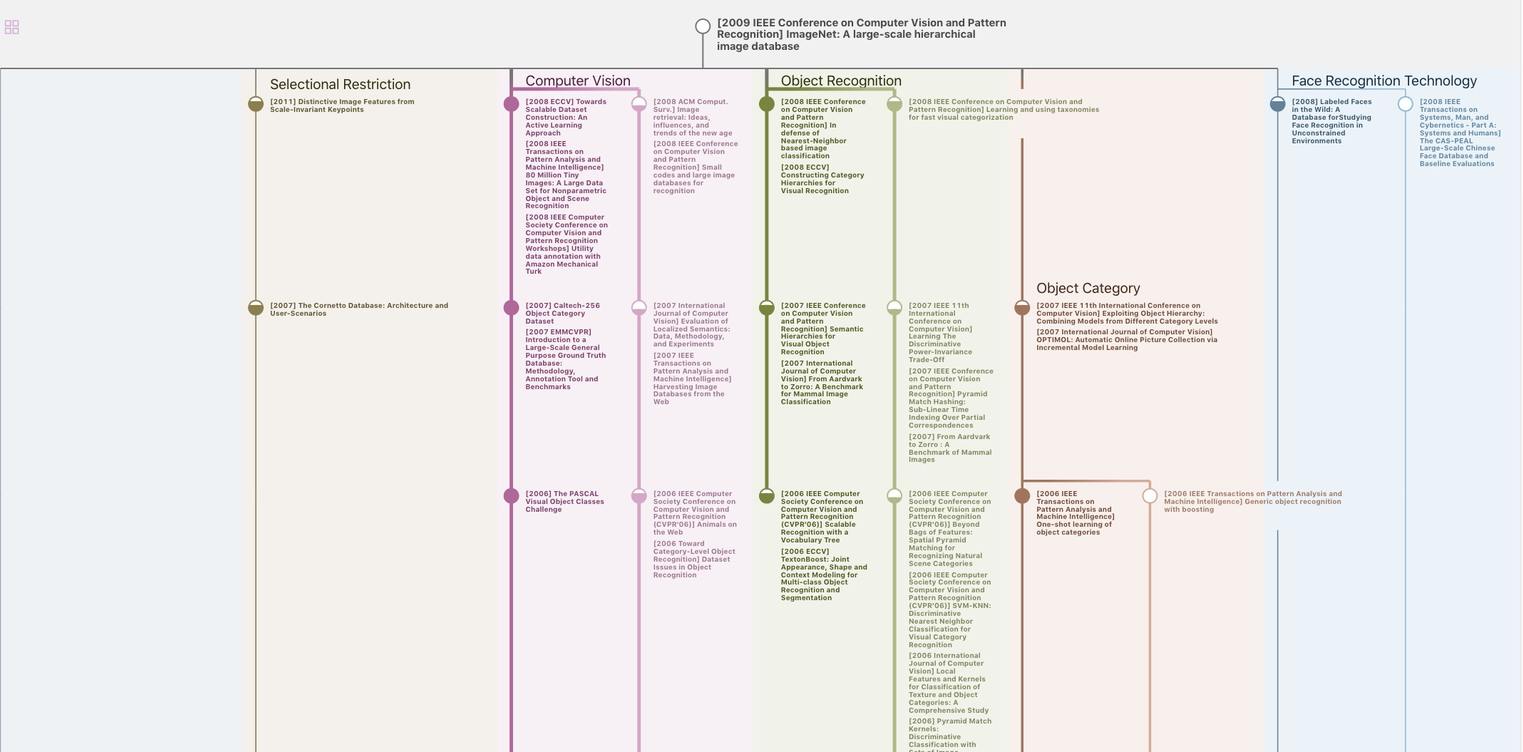
生成溯源树,研究论文发展脉络
Chat Paper
正在生成论文摘要