Target-independent XLA optimization using Reinforcement Learning
CoRR(2023)
摘要
An important challenge in Machine Learning compilers like XLA is multi-pass optimization and analysis. There has been recent interest chiefly in XLA target-dependent optimization on the graph-level, subgraph-level, and kernel-level phases. We specifically focus on target-independent optimization XLA HLO pass ordering: our approach aims at finding the optimal sequence of compiler optimization passes, which is decoupled from target-dependent optimization. However, there is little domain specific study in pass ordering for XLA HLO. To this end, we propose introducing deep Reinforcement Learning (RL) based search for optimal XLA HLO pass ordering. We also propose enhancements to the deep RL algorithms to further improve optimal search performance and open the research direction for domain-specific guidance for RL. We create an XLA Gym experimentation framework as a tool to enable RL algorithms to interact with the compiler for passing optimizations and thereby train agents. Overall, in our experimentation we observe an average of $13.3\%$ improvement in operation count reduction on a benchmark of GPT-2 training graphs and $10.4\%$ improvement on a diverse benchmark including GPT-2, BERT, and ResNet graphs using the proposed approach over the compiler's default phase ordering.
更多查看译文
关键词
reinforcement learning,optimization,target-independent
AI 理解论文
溯源树
样例
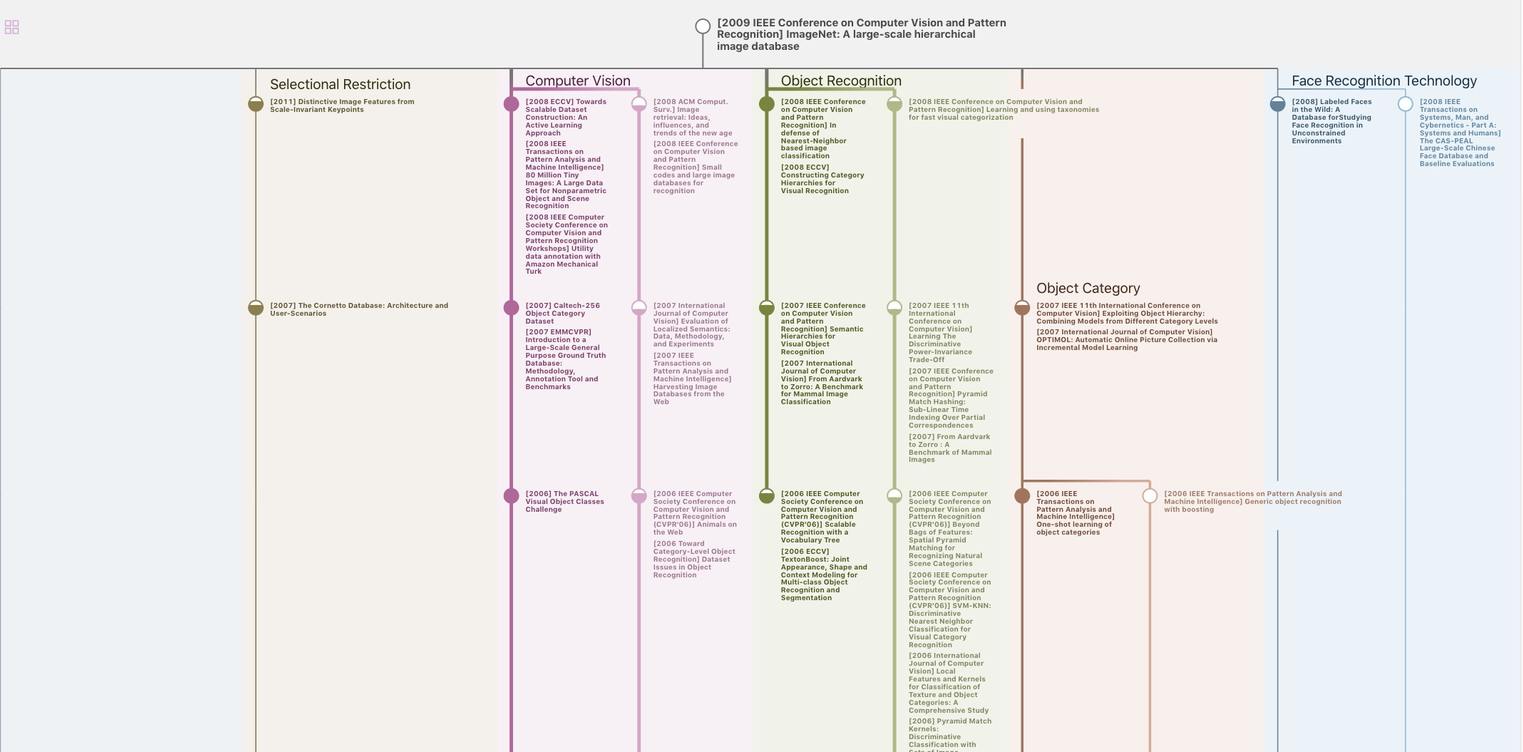
生成溯源树,研究论文发展脉络
Chat Paper
正在生成论文摘要