Multi-agent Game Domain: Monopoly
Synthesis lectures on computer vision(2023)
摘要
In the previous chapters we have looked at the visual domain and single-agent environments for action domains. In this chapter, we will apply the novelty framework to a multi-agent game environment. As an example of multi-agent game environment, we introduce a simulated version of the Monopoly board game. Monopoly is a multi-agent board game that involves four players taking turns by rolling a pair of unbiased dice and making decisions. The conventional Monopoly board consists of 40 square locations which include 22 real estate locations, 4 railroads, and 2 utility locations that players can buy, sell, or trade. Furthermore, there are squares that correspond to “Go,” a jail location, card locations, and the free parking location. Figure 7.1 shows all assets, their corresponding purchase prices, and color. We setup the Monopoly simulator to have one learning-based agent ( $$\alpha _{\mathcal{T}}$$ ) and three fixed-policy agents. These constitute the four players in the game. The objective of the learning-based agent ( $$\alpha _{\mathcal{T}}$$ ) is to learn winning strategies for Monopoly. Formally, the task $$\mathcal T$$ of the agent, $$\alpha _{\mathcal{T}}$$ , is defined as: given the observation space $$x_t \in \mathcal O$$ at time t, select an action $$a_t \in \mathcal A$$ to maximize the overall reward resulting in a higher win rate.
更多查看译文
关键词
game,multi-agent
AI 理解论文
溯源树
样例
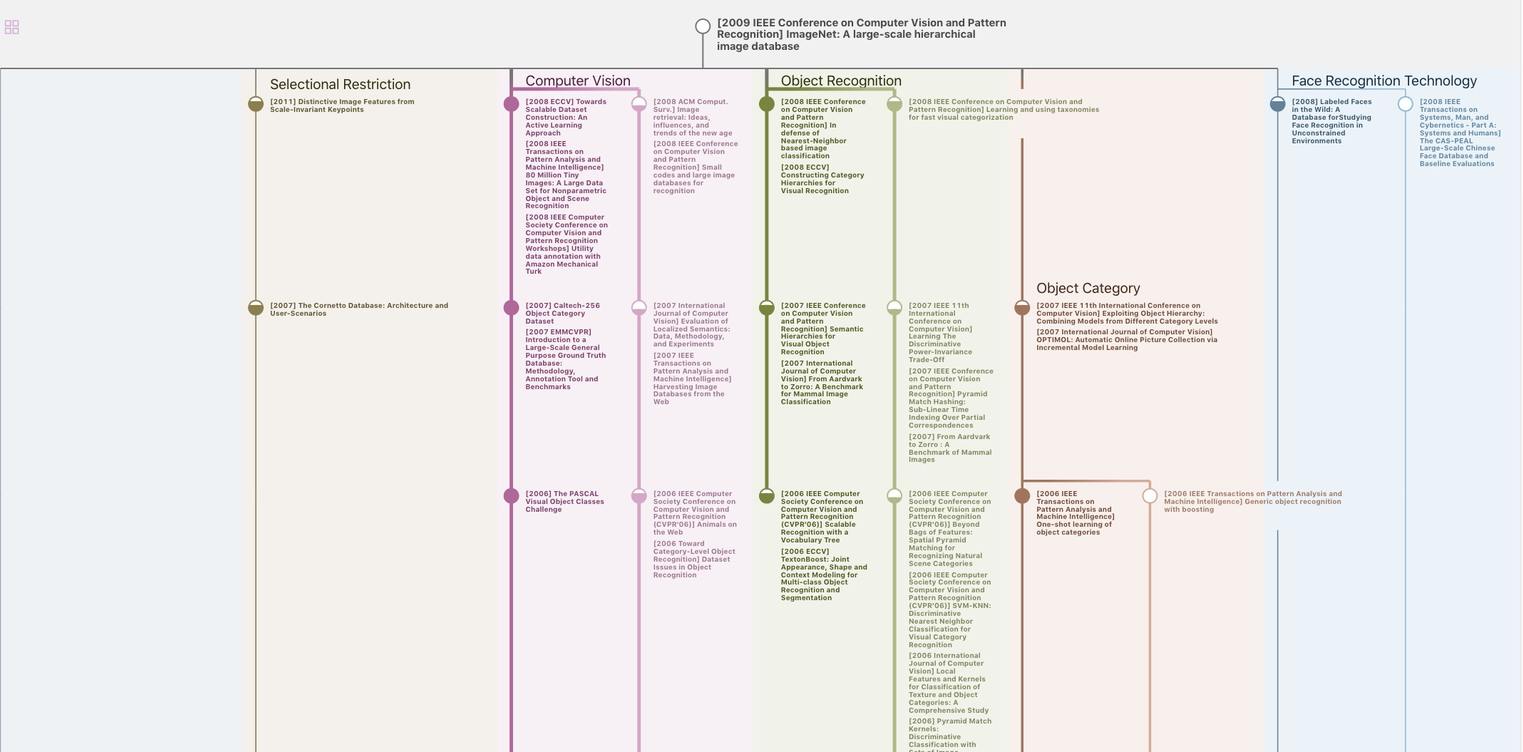
生成溯源树,研究论文发展脉络
Chat Paper
正在生成论文摘要