Computing Domain Abstractions for Optimal Classical Planning with Counterexample-Guided Abstraction Refinement.
ICAPS(2023)
摘要
Abstraction heuristics are the state of the art in optimal classical planning as heuristic search. A popular method for computing abstractions is the counterexample-guided abstraction refinement (CEGAR) principle, which has successfully been used for projections, which are the abstractions underlying pattern databases, and Cartesian abstractions. While projections are simple and fast to compute, Cartesian abstractions subsume projections and hence allow more fine-grained abstractions, however at the expense of efficiency. Domain abstractions are a third class of abstractions between projections and Cartesian abstractions in terms of generality. Yet, to the best of our knowledge, they are only briefly considered in the planning literature but have not been used for computing heuristics yet. We aim to close this gap and compute domain abstractions by using the CEGAR principle. Our empirical results show that domain abstractions compare favorably against projections and Cartesian abstractions.
更多查看译文
关键词
optimal classical planning,computing,counterexample-guided
AI 理解论文
溯源树
样例
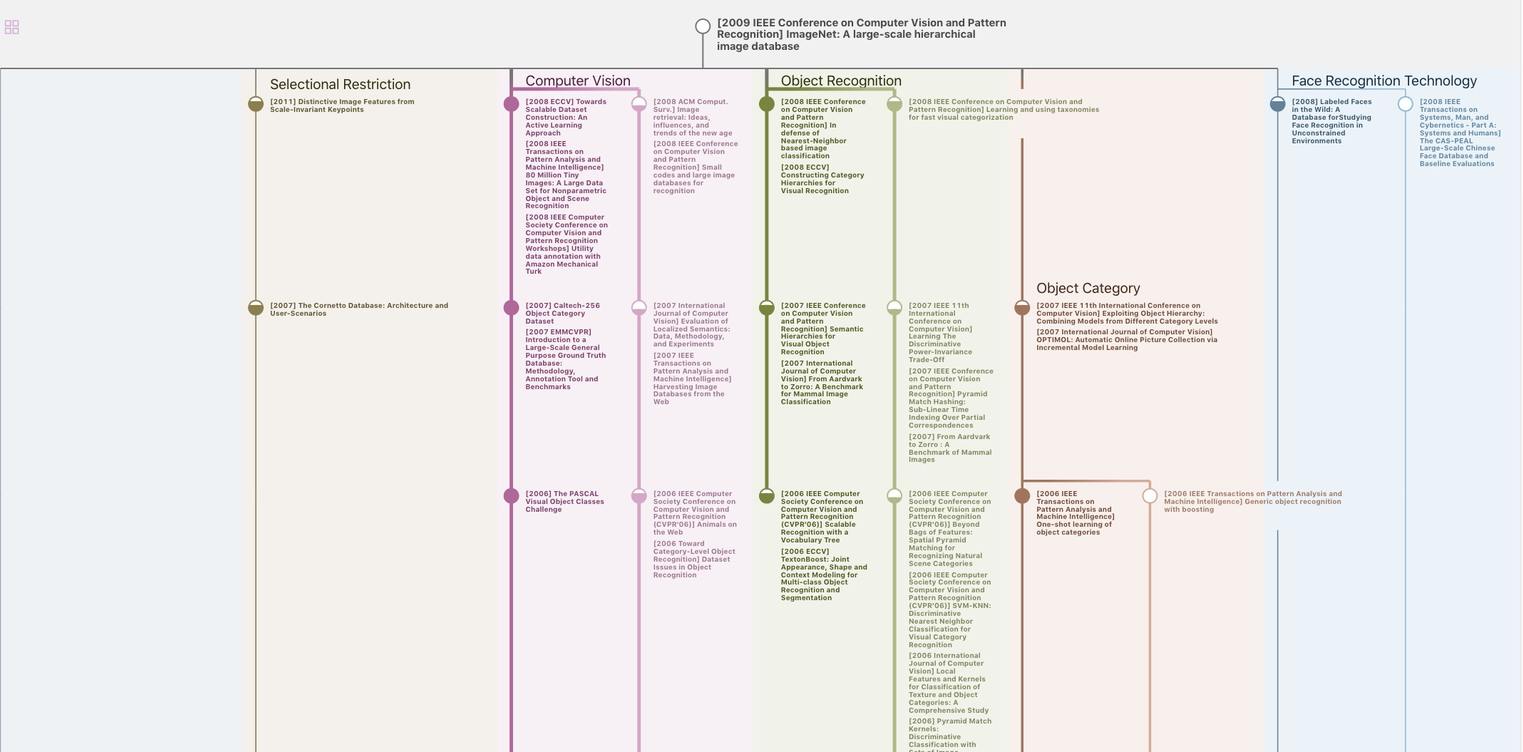
生成溯源树,研究论文发展脉络
Chat Paper
正在生成论文摘要