Time Series Continuous Modeling for Imputation and Forecasting with Implicit Neural Representations
arXiv (Cornell University)(2023)
摘要
We introduce a novel modeling approach for time series imputation and forecasting, tailored to address the challenges often encountered in real-world data, such as irregular samples, missing data, or unaligned measurements from multiple sensors. Our method relies on a continuous-time-dependent model of the series' evolution dynamics. It leverages adaptations of conditional, implicit neural representations for sequential data. A modulation mechanism, driven by a meta-learning algorithm, allows adaptation to unseen samples and extrapolation beyond observed time-windows for long-term predictions. The model provides a highly flexible and unified framework for imputation and forecasting tasks across a wide range of challenging scenarios. It achieves state-of-the-art performance on classical benchmarks and outperforms alternative time-continuous models.
更多查看译文
关键词
implicit neural representations,imputation,forecasting,modeling
AI 理解论文
溯源树
样例
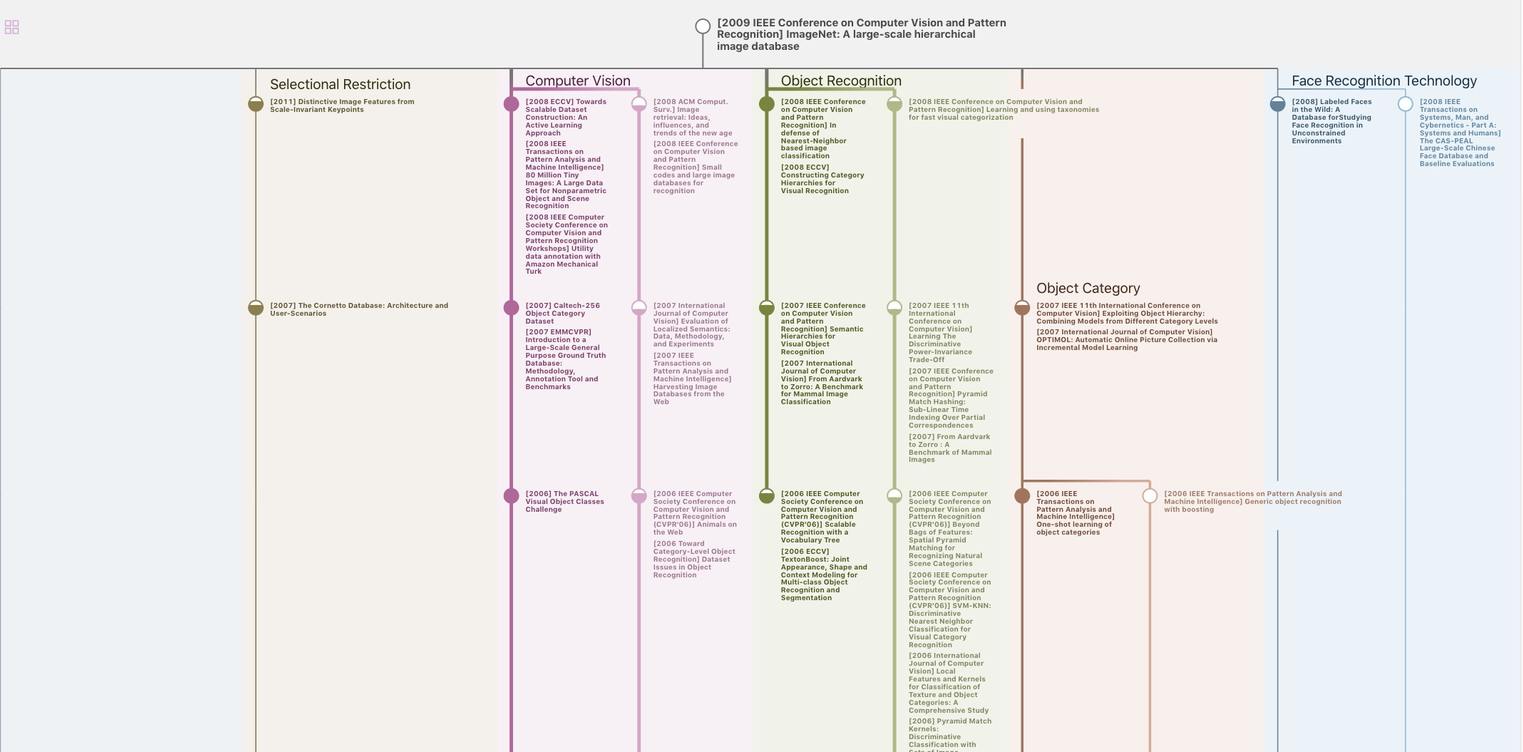
生成溯源树,研究论文发展脉络
Chat Paper
正在生成论文摘要