Interpretable Distribution-Invariant Fairness Measures for Continuous Scores
CoRR(2023)
摘要
Measures of algorithmic fairness are usually discussed in the context of binary decisions. We extend the approach to continuous scores. So far, ROC-based measures have mainly been suggested for this purpose. Other existing methods depend heavily on the distribution of scores, are unsuitable for ranking tasks, or their effect sizes are not interpretable. Here, we propose a distributionally invariant version of fairness measures for continuous scores with a reasonable interpretation based on the Wasserstein distance. Our measures are easily computable and well suited for quantifying and interpreting the strength of group disparities as well as for comparing biases across different models, datasets, or time points. We derive a link between the different families of existing fairness measures for scores and show that the proposed distributionally invariant fairness measures outperform ROC-based fairness measures because they are more explicit and can quantify significant biases that ROC-based fairness measures miss. Finally, we demonstrate their effectiveness through experiments on the most commonly used fairness benchmark datasets.
更多查看译文
关键词
fairness,distribution-invariant
AI 理解论文
溯源树
样例
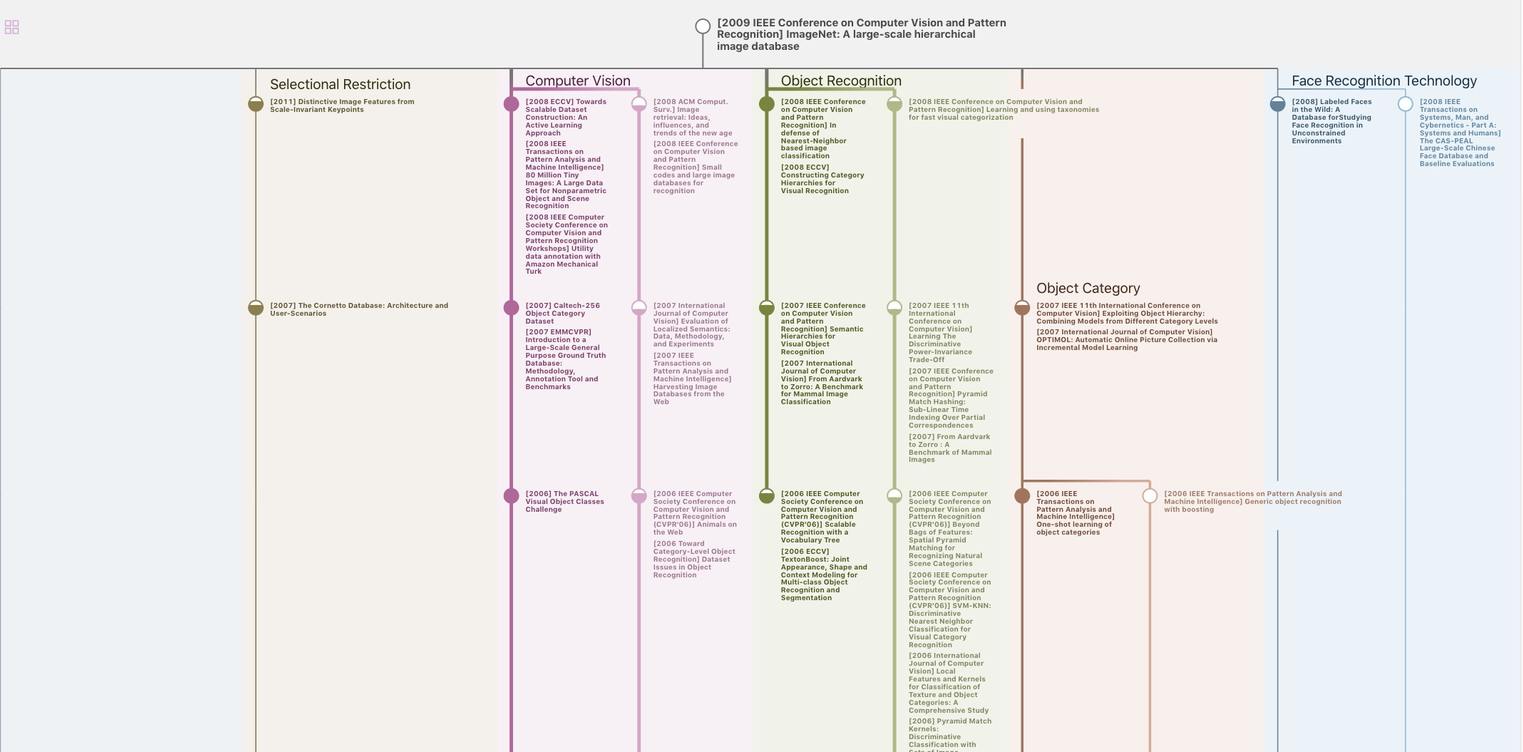
生成溯源树,研究论文发展脉络
Chat Paper
正在生成论文摘要