Cupid: Leveraging ChatGPT for More Accurate Duplicate Bug Report Detection
CoRR(2023)
摘要
Duplicate bug report detection (DBRD) is a long-standing challenge in both academia and industry. Over the past decades, researchers have proposed various approaches to detect duplicate bug reports more accurately. With the recent advancement of deep learning, researchers have also proposed several approaches that leverage deep learning models to detect duplicate bug reports. A recent benchmarking study on DBRD also reveals that the performance of deep learning-based approaches is not always better than the traditional approaches. However, traditional approaches have limitations, e.g., they are usually based on the bag-of-words model, which cannot capture the semantics of bug reports. To address these aforementioned challenges, we seek to leverage state-of-the-art large language model to improve the performance of the traditional DBRD approach. In this paper, we propose an approach called Cupid, which combines the best-performing traditional DBRD approach REP with the state-of-the-art large language model ChatGPT. Specifically, we first leverage ChatGPT under the zero-shot setting to get essential information on bug reports. We then use the essential information as the input of REP to detect duplicate bug reports. We conducted an evaluation on comparing Cupid with three existing approaches on three datasets. The experimental results show that Cupid achieves new state-of-the-art results, reaching Recall Rate@10 scores ranging from 0.59 to 0.67 across all the datasets analyzed. Our work highlights the potential of combining large language models to improve the performance of software engineering tasks.
更多查看译文
关键词
chatgpt
AI 理解论文
溯源树
样例
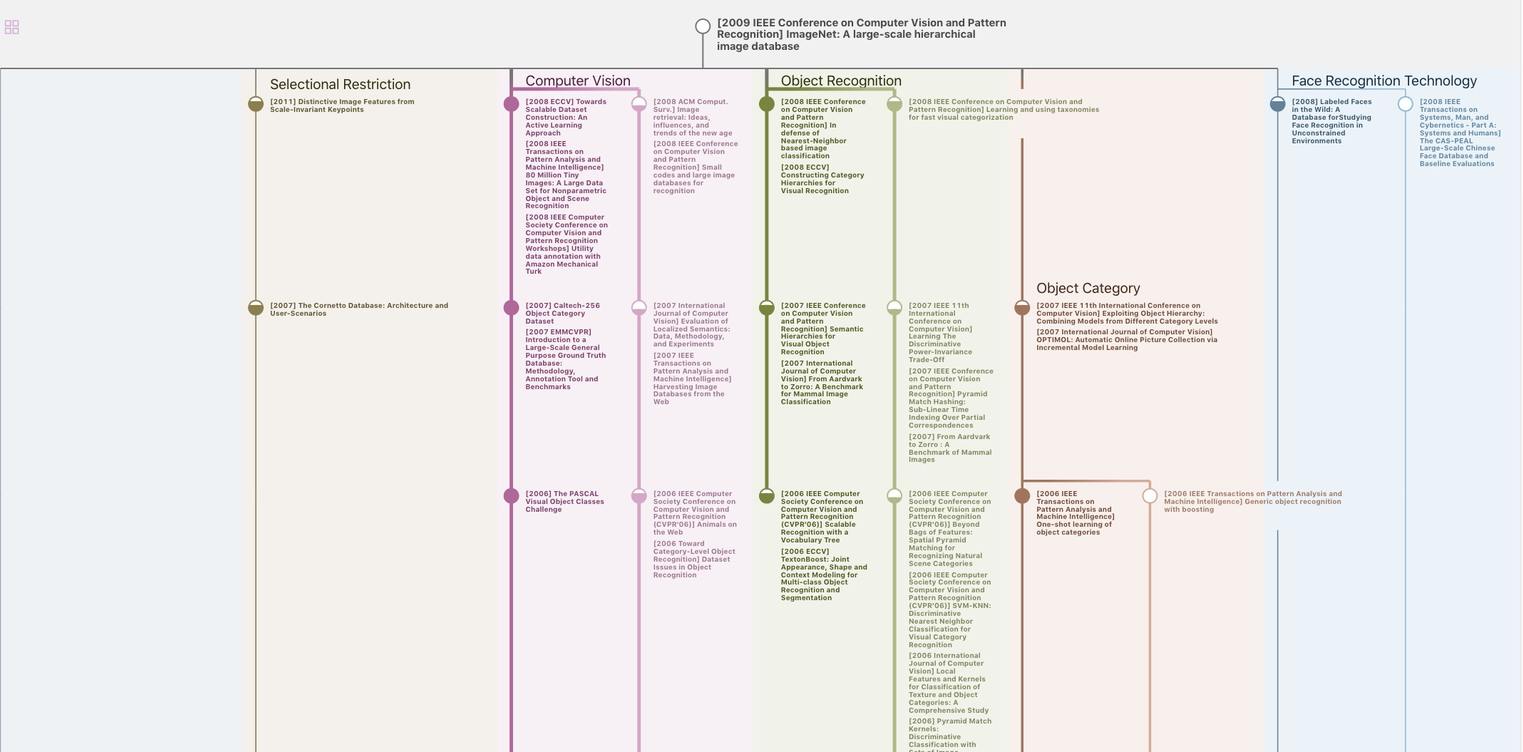
生成溯源树,研究论文发展脉络
Chat Paper
正在生成论文摘要