Radiomics-Informed Deep Learning for Classification of Atrial Fibrillation Sub-Types from Left-Atrium CT Volumes
MEDICAL IMAGE COMPUTING AND COMPUTER ASSISTED INTERVENTION, MICCAI 2023, PT VII(2023)
摘要
Atrial Fibrillation (AF) is characterized by rapid, irregular heartbeats, and can lead to fatal complications such as heart failure. The disease is divided into two sub-types based on severity, which can be automatically classified through CT volumes for disease screening of severe cases. However, existing classification approaches rely on generic radiomic features that may not be optimal for the task, whilst deep learning methods tend to over-fit to the high-dimensional volume inputs. In this work, we propose a novel radiomics-informed deep-learning method, RIDL, that combines the advantages of deep learning and radiomic approaches to improve AF sub-type classification. Unlike existing hybrid techniques that mostly rely on naive feature concatenation, we observe that radiomic feature selection methods can serve as an information prior, and propose supplementing low-level deep neural network (DNN) features with locally computed radiomic features. This reduces DNN over-fitting and allows local variations between radiomic features to be better captured. Furthermore, we ensure complementary information is learned by deep and radiomic features by designing a novel feature de-correlation loss. Combined, our method addresses the limitations of deep learning and radiomic approaches and outperforms state-of-the-art radiomic, deep learning, and hybrid approaches, achieving 86.9% AUC for the AF sub-type classification task. Code is available at https://github.com/xmed-lab/RIDL.
更多查看译文
关键词
Atrial Fibrillation,Radiomics,CT Imaging Analysis
AI 理解论文
溯源树
样例
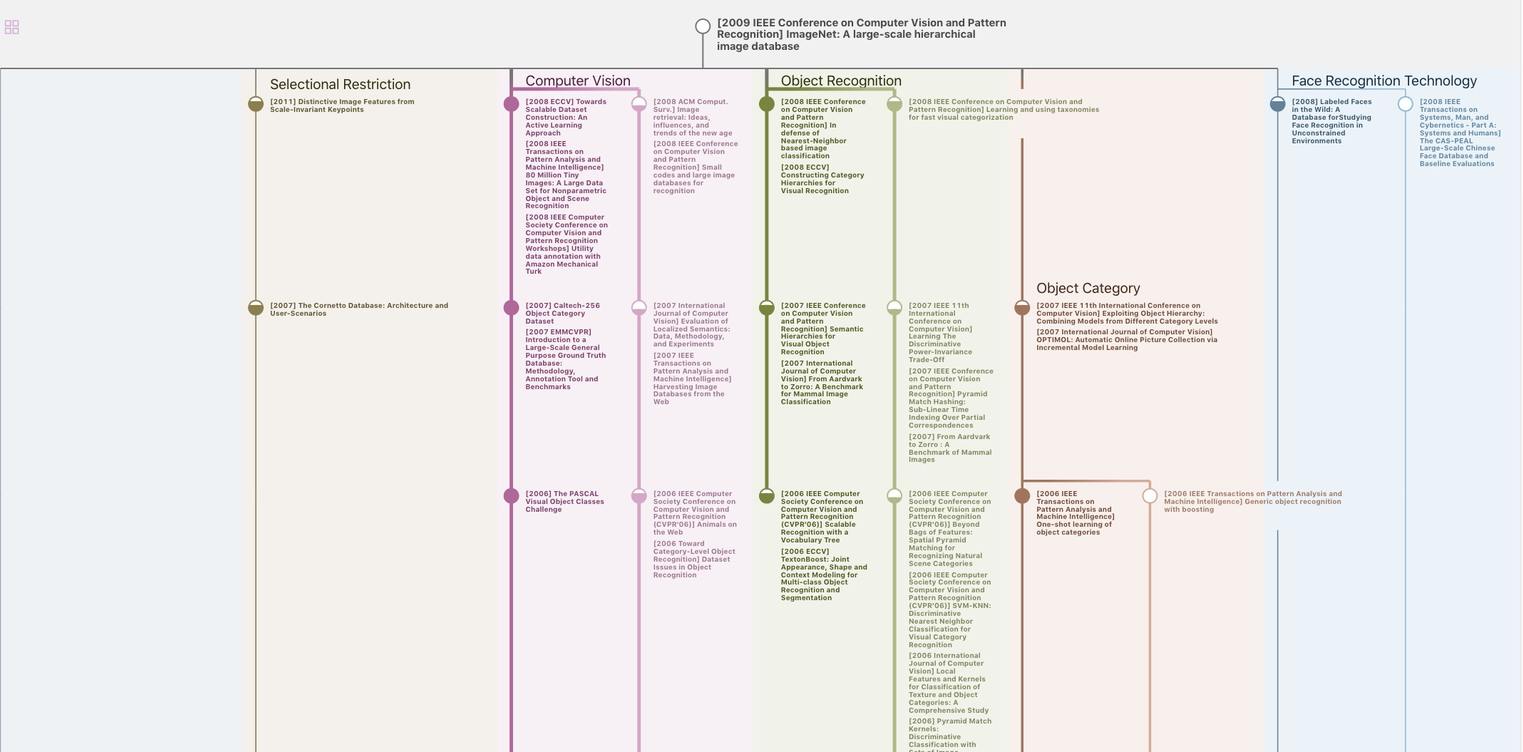
生成溯源树,研究论文发展脉络
Chat Paper
正在生成论文摘要