Measuring the Impact of Domain Factors in Self-Supervised Pre-Training
2023 IEEE International Conference on Acoustics, Speech, and Signal Processing Workshops (ICASSPW)(2023)
摘要
Human speech data comprises a rich set of domain factors such as accent, syntactic and semantic variety, or acoustic environment. Previous work explores the effect of domain mismatch in automatic speech recognition between pre-training and fine-tuning as a whole [1] but does not dissect the contribution of individual factors. In this paper, we present a controlled study to better understand the effect of such factors on the performance of pre-trained representations on automatic speech recognition. To do so, we pre-train models either on modified natural speech or synthesized audio, with a single domain factor modified, and then measure performance after fine-tuning. Results show that phonetic domain factors play an important role during pre-training while grammatical and syntactic factors are far less important. To our knowledge, this is the first study to better understand the domain characteristics of pre-trained sets in self-supervised pre-training for speech.
更多查看译文
关键词
speech recognition, self-supervised learning, domain mismatch
AI 理解论文
溯源树
样例
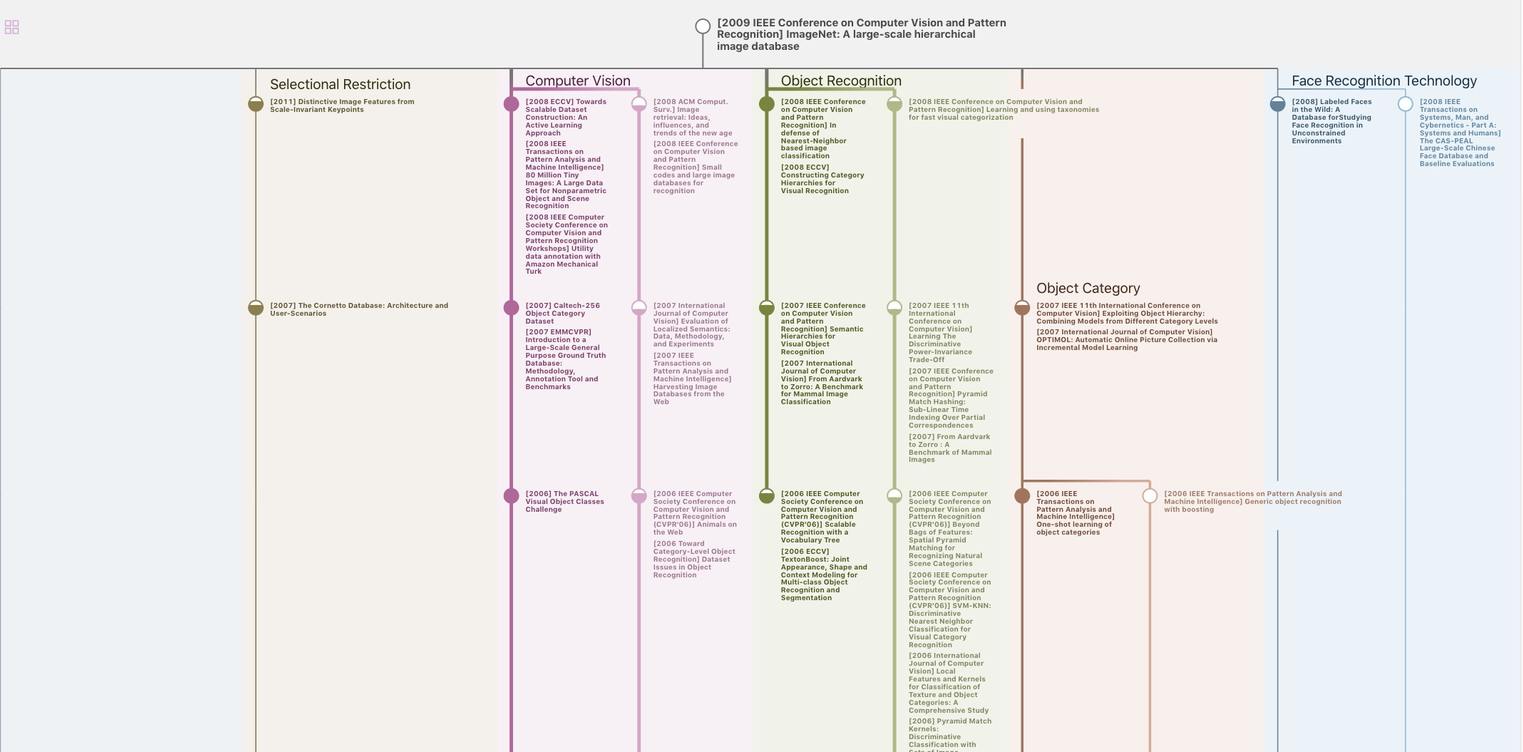
生成溯源树,研究论文发展脉络
Chat Paper
正在生成论文摘要