Enhancing Dynamic GCN for Node Attribute Forecasting with Meta Spatial-Temporal Learning (Student Abstract).
AAAI(2023)
摘要
Node attribute forecasting has recently attracted considerable attention. Recent attempts have thus far utilized dynamic graph convolutional network (GCN) to predict future node attributes. However, few prior works have noticed the complex spatial and temporal interaction between nodes, which will hamper the performance of dynamic GCN. In this paper, we propose a new dynamic GCN model named meta-DGCN, leveraging meta spatial-temporal tasks to enhance the ability of dynamic GCN for better capturing node attributes in the future. Experiments show that meta-DGCN effectively models comprehensive Spatio-temporal correlations between nodes and outperforms state-of-the-art baselines on various real-world datasets.
更多查看译文
关键词
node attribute forecasting,dynamic gcn,learning,spatial-temporal
AI 理解论文
溯源树
样例
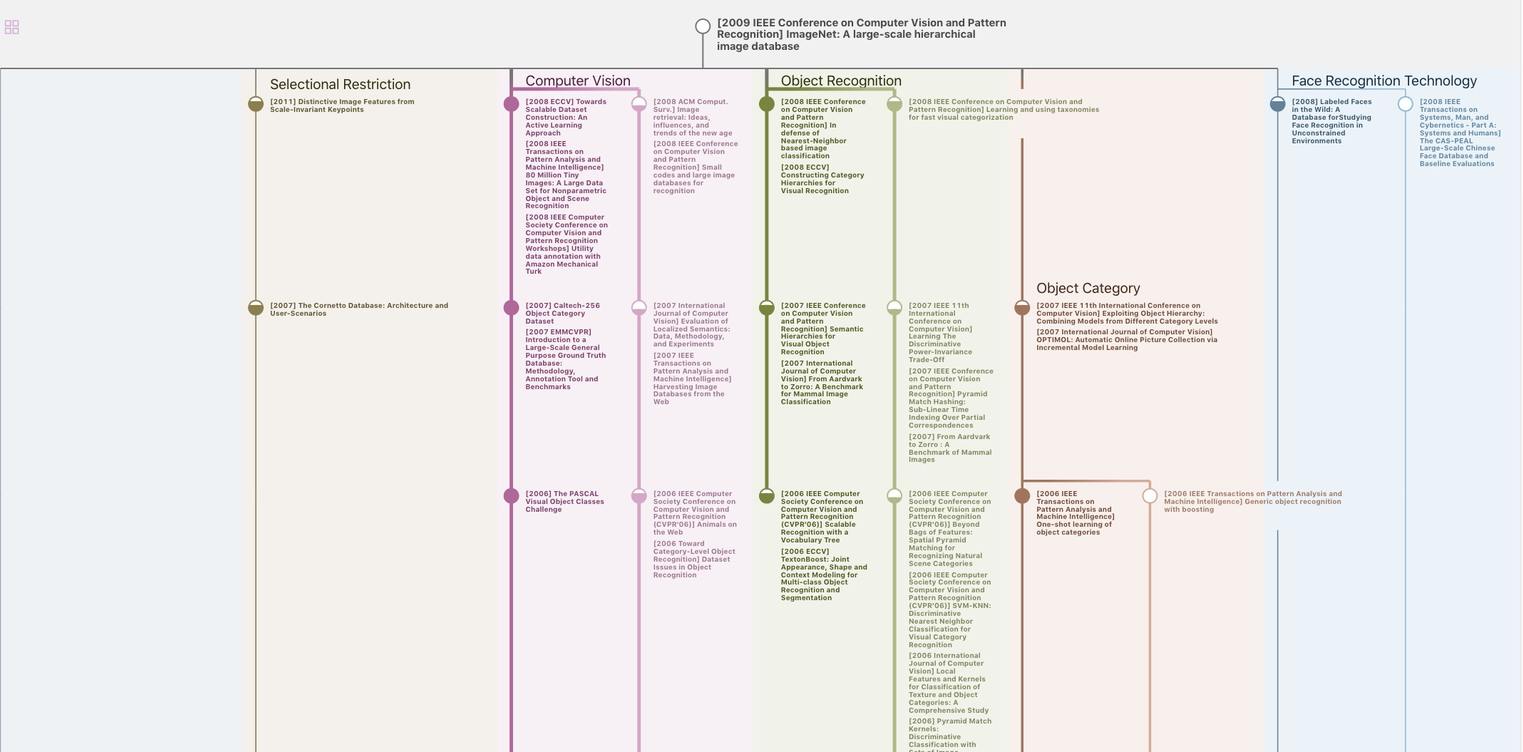
生成溯源树,研究论文发展脉络
Chat Paper
正在生成论文摘要