Distributed Projection-Free Online Learning for Smooth and Convex Losses.
AAAI(2023)
摘要
We investigate the problem of distributed online convex optimization with complicated constraints, in which the projection operation could be the computational bottleneck. To avoid projections, distributed online projection-free methods have been proposed and attain an O ( T 3/4 ) regret bound for general convex losses. However, they cannot utilize the smoothness condition, which has been exploited in the centralized setting to improve the regret. In this paper, we propose a new distributed online projection-free method with a tighter regret bound of O ( T 2/3 ) for smooth and convex losses. Specifically, we first provide a distributed extension of Follow-the-Perturbed-Leader so that the smoothness can be utilized in the distributed setting. Then, we reduce the computational cost via sampling and blocking techniques. In this way, our method only needs to solve one linear optimization per round on average. Finally, we conduct experiments on benchmark datasets to verify the effectiveness of our proposed method.
更多查看译文
关键词
convex losses,learning,projection-free
AI 理解论文
溯源树
样例
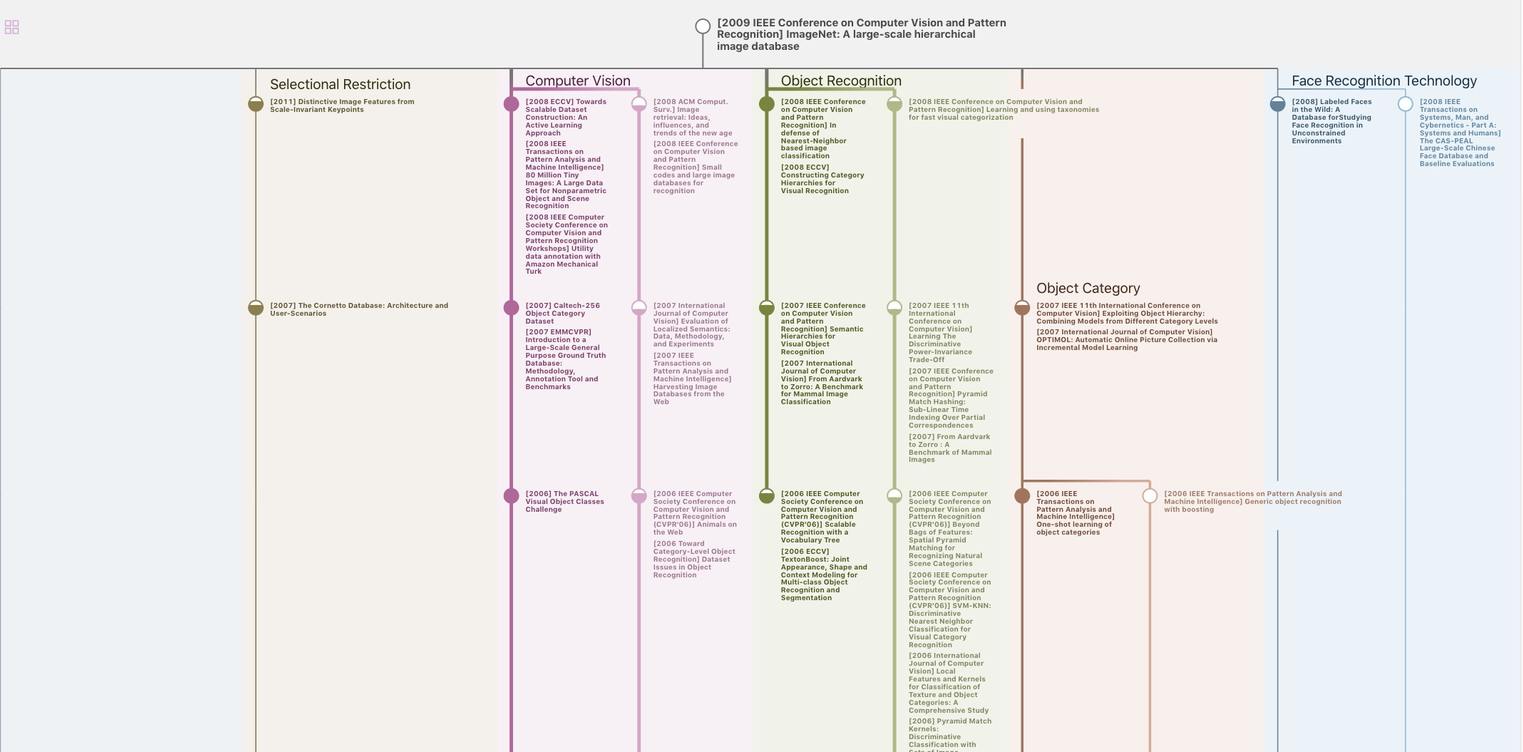
生成溯源树,研究论文发展脉络
Chat Paper
正在生成论文摘要