Predicting masked tokens in stochastic locations improves masked image modeling
CoRR(2023)
摘要
Self-supervised learning is a promising paradigm in deep learning that enables learning from unlabeled data by constructing pretext tasks that require learning useful representations. In natural language processing, the dominant pretext task has been masked language modeling (MLM), while in computer vision there exists an equivalent called Masked Image Modeling (MIM). However, MIM is challenging because it requires predicting semantic content in accurate locations. E.g, given an incomplete picture of a dog, we can guess that there is a tail, but we cannot determine its exact location. In this work, we propose FlexPredict, a stochastic model that addresses this challenge by incorporating location uncertainty into the model. Specifically, we condition the model on stochastic masked token positions to guide the model toward learning features that are more robust to location uncertainties. Our approach improves downstream performance on a range of tasks, e.g, compared to MIM baselines, FlexPredict boosts ImageNet linear probing by 1.6% with ViT-B and by 2.5% for semi-supervised video segmentation using ViT-L.
更多查看译文
关键词
stochastic locations,tokens,image
AI 理解论文
溯源树
样例
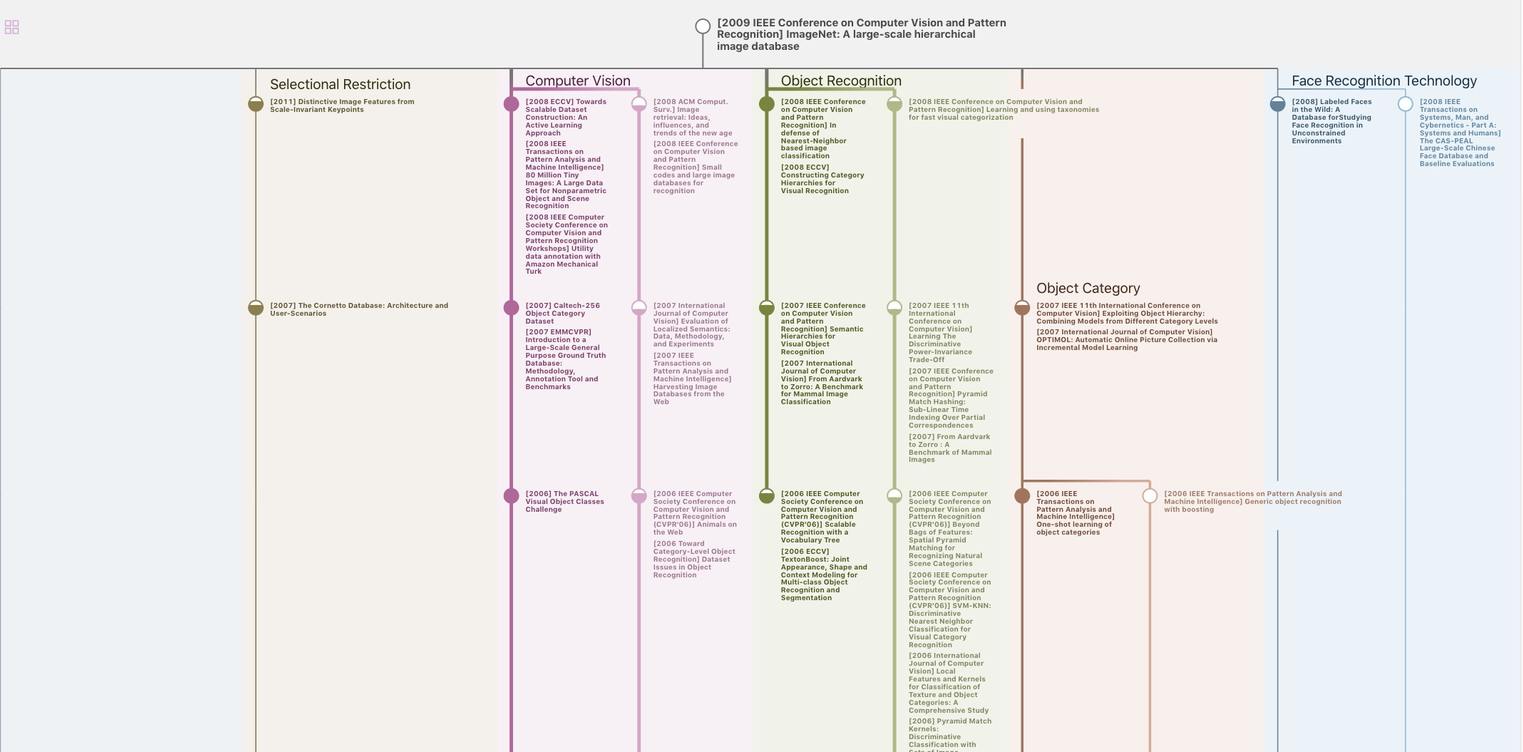
生成溯源树,研究论文发展脉络
Chat Paper
正在生成论文摘要