Sequential and Shared-Memory Parallel Algorithms for Partitioned Local Depths
CoRR(2023)
摘要
In this work, we design, analyze, and optimize sequential and shared-memory parallel algorithms for partitioned local depths (PaLD). Given a set of data points and pairwise distances, PaLD is a method for identifying strength of pairwise relationships based on relative distances, enabling the identification of strong ties within dense and sparse communities even if their sizes and within-community absolute distances vary greatly. We design two algorithmic variants that perform community structure analysis through triplet comparisons of pairwise distances. We present theoretical analyses of computation and communication costs and prove that the sequential algorithms are communication optimal, up to constant factors. We introduce performance optimization strategies that yield sequential speedups of up to $29\times$ over a baseline sequential implementation and parallel speedups of up to $19.4\times$ over optimized sequential implementations using up to $32$ threads on an Intel multicore CPU.
更多查看译文
关键词
parallel,algorithms,partitioned,shared-memory
AI 理解论文
溯源树
样例
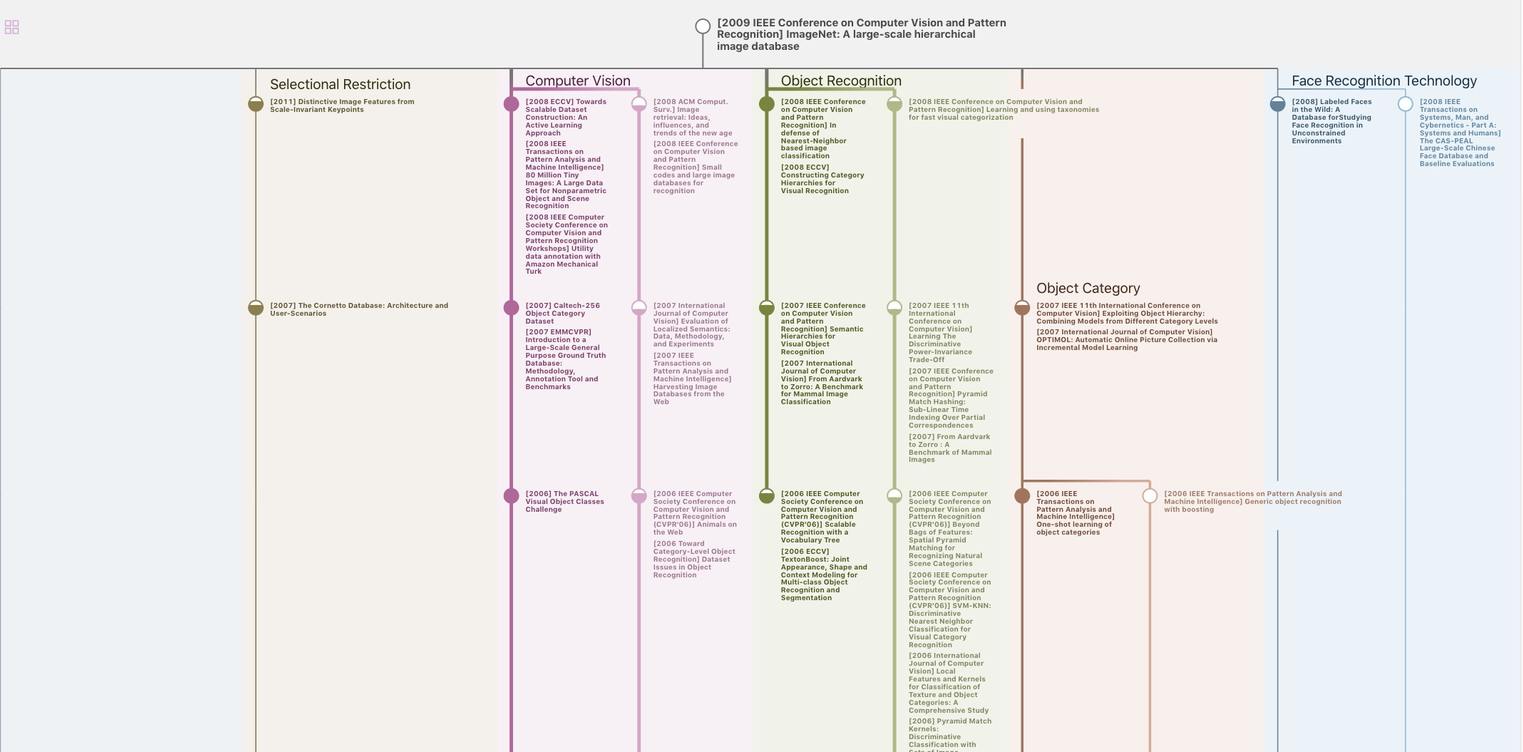
生成溯源树,研究论文发展脉络
Chat Paper
正在生成论文摘要