UniAP: Unifying Inter- and Intra-Layer Automatic Parallelism by Mixed Integer Quadratic Programming
CoRR(2023)
摘要
Distributed learning is commonly used for training deep learning models,
especially large models. In distributed learning, manual parallelism (MP)
methods demand considerable human effort and have limited flexibility. Hence,
automatic parallelism (AP) methods have recently been proposed for automating
the parallel strategy optimization process. Existing AP methods suffer from
sub-optimal solutions because they do not jointly optimize the two categories
of parallel strategies (i.e., inter-layer parallelism and intra-layer
parallelism). In this paper, we propose a novel AP method called UniAP, which
unifies inter- and intra-layer automatic parallelism by mixed integer quadratic
programming. To the best of our knowledge, UniAP is the first parallel method
that can jointly optimize the two categories of parallel strategies to find an
optimal solution. Experimental results show that UniAP outperforms
state-of-the-art methods by up to 1.71× in throughput and reduces
strategy optimization time by up to 107× across five Transformer-based
models.
更多查看译文
关键词
integer quadratic programming,intra-layer
AI 理解论文
溯源树
样例
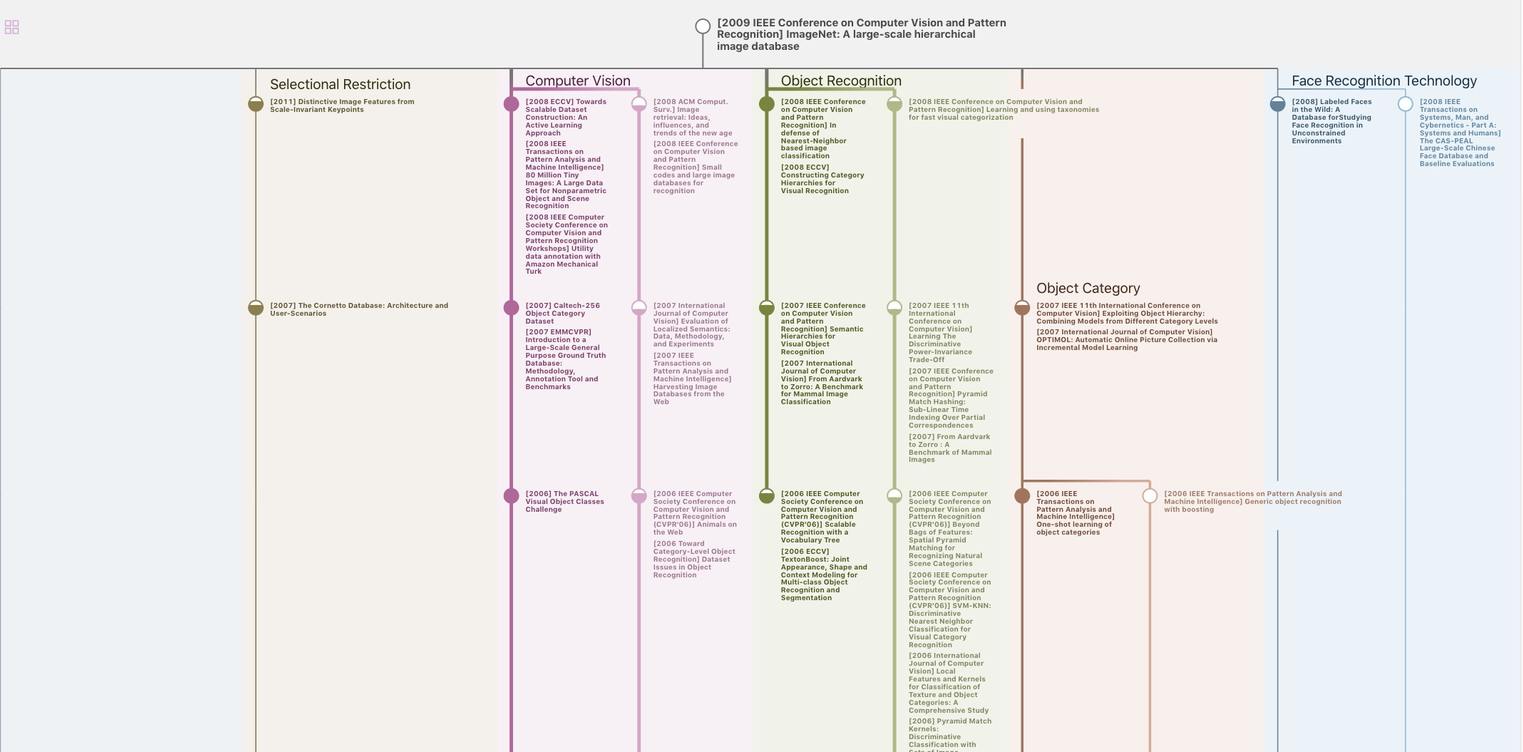
生成溯源树,研究论文发展脉络
Chat Paper
正在生成论文摘要