OCMGen: Extended Design Space Exploration with Efficient FPGA Memory Inference.
FCCM(2023)
摘要
Deep learning applications demand high memory storage and computational power to operate on millions of parameters. Field Programmable Gate Arrays (FPGAs), with high compute resources and the ability to store data on-chip in their distributed memory components such as Block RAM (BRAM) and Ultra RAM (URAM), are good candidates to deploy such memory-intensive applications [1]. However, without careful tailoring of the hardware design for a target device, current synthesis tools (e.g., Xilinx Vivado) can severely underutilize these RAM primitives reducing the usable on-chip memory (OCM). Consequently, this forces the accelerator to perform more frequent expensive off-chip accesses, limiting its performance.
更多查看译文
关键词
n/a
AI 理解论文
溯源树
样例
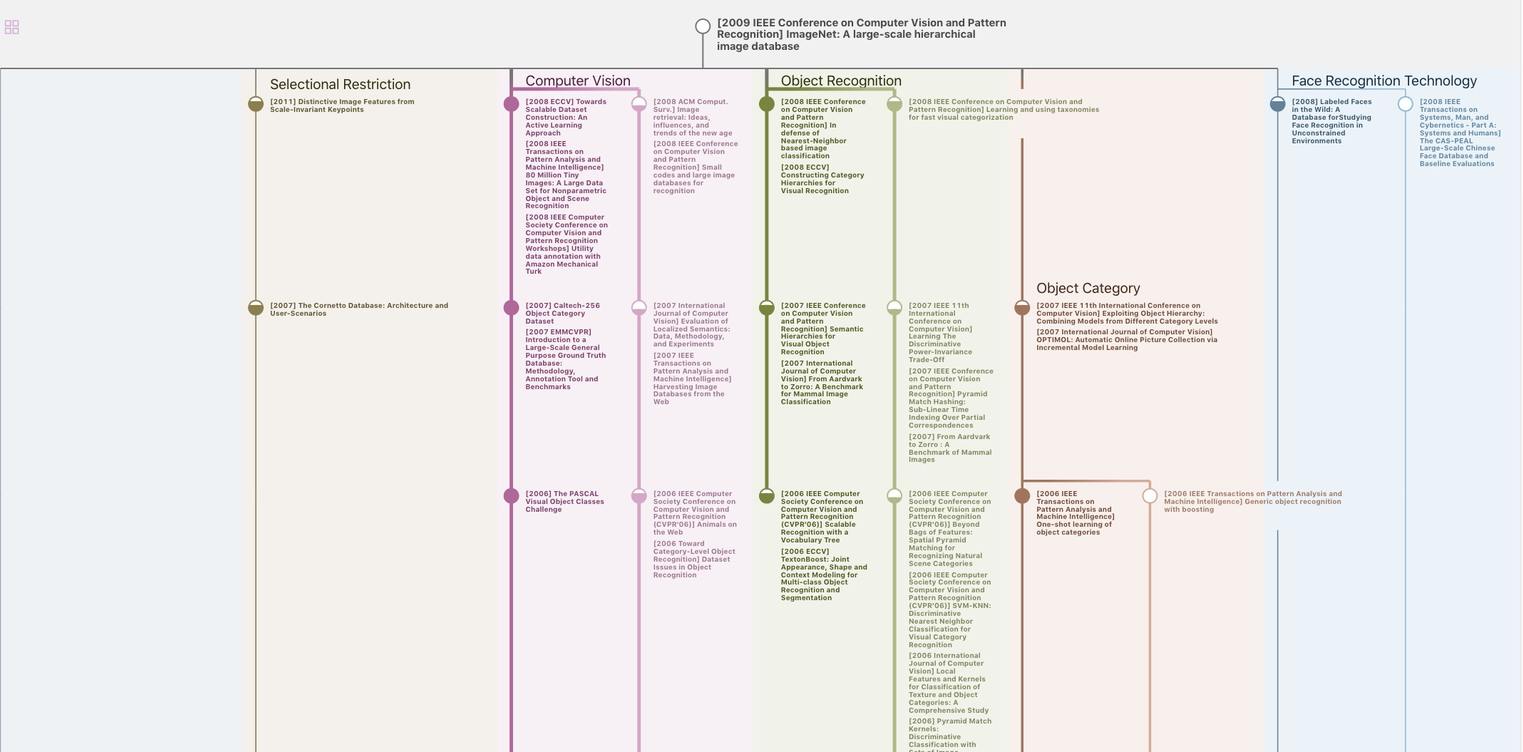
生成溯源树,研究论文发展脉络
Chat Paper
正在生成论文摘要