Relieving Coefficient Learning in Genetic Programming for Symbolic Regression via Correlation and Linear Scaling
GECCO(2023)
摘要
The difficulty of learning optimal coefficients in regression models using only genetic operators has long been a challenge in genetic programming for symbolic regression. As a simple but effective remedy it has been proposed to perform linear scaling of model outputs prior to a fitness evaluation. Recently, the use of a correlation coefficient-based fitness function with a post-processing linear scaling step for model alignment has been shown to outperform error-based fitness functions in generating symbolic regression models. In this study, we compare the impact of four evaluation strategies on relieving genetic programming (GP) from learning coefficients in symbolic regression and focusing on learning the more crucial model structure. The results from 12 datasets, including ten real-world tasks and two synthetic datasets, confirm that all these strategies assist GP to varying degrees in learning coefficients. Among the them, correlation fitness with one-time linear scaling as post-processing, due to be the most efficient while bringing notable benefits to the performance, is the recommended strategy to relieve GP from learning coefficients.
更多查看译文
关键词
Genetic Programming,Symbolic Regression,Fitness Function,Correlation,Linear Scaling
AI 理解论文
溯源树
样例
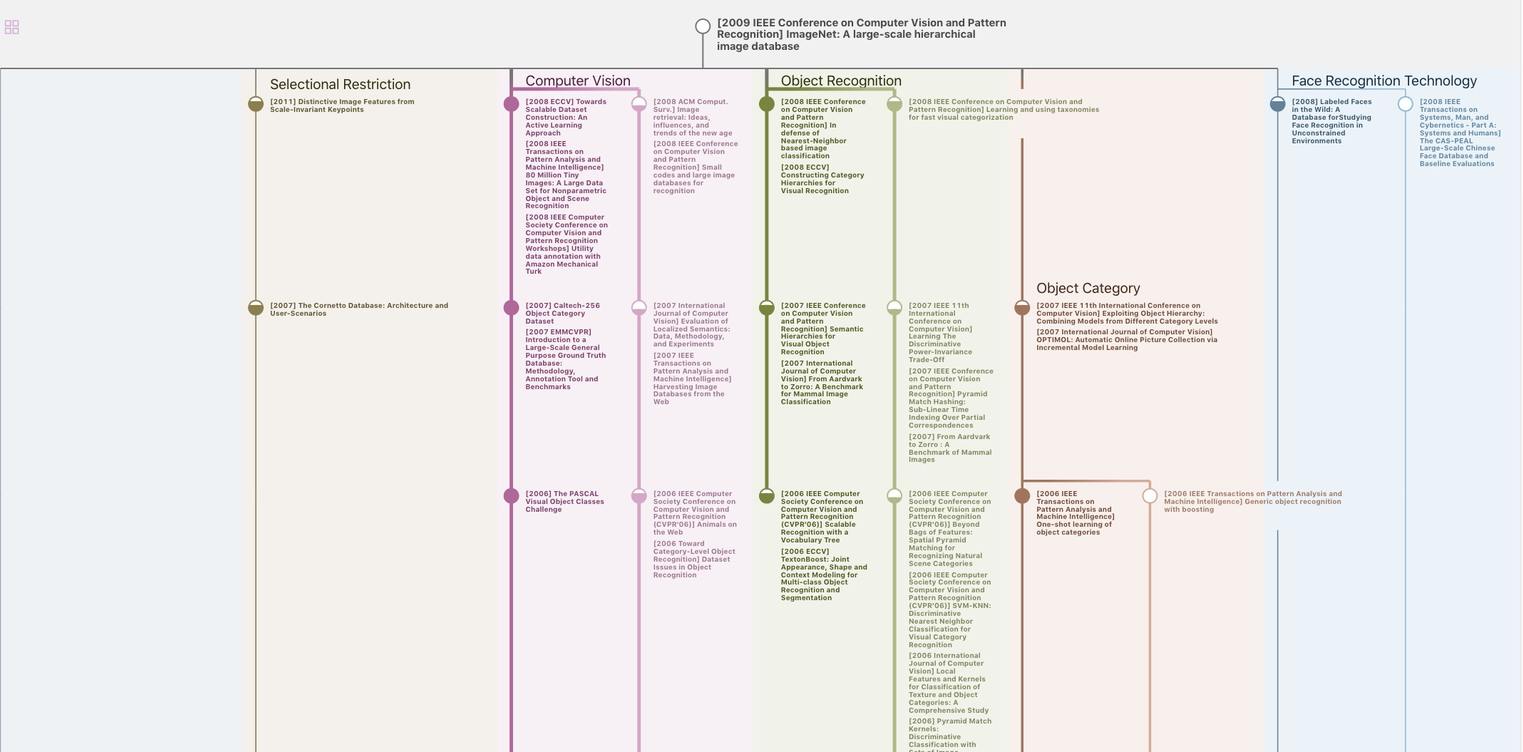
生成溯源树,研究论文发展脉络
Chat Paper
正在生成论文摘要