RM-FSP: Regret minimization optimizes neural fictitious self-play
Neurocomputing(2023)
摘要
To compute the optimal strategy in competitive games, algorithms have been developed to achieve the Nash equilibrium. Current deep learning algorithms have succeeded in many games; however, optimizing the algorithms to approach the Nash equilibrium in imperfect-information games like StarCraft and Poker remains challenging. Neural Fictitious Self-Play (NFSP) is an effective end-to-end algorithm to learn an approximate Nash equilibrium in imperfect-information games. However, because a player in NFSP trains its best response according to its opponents' past strategies, a discrepancy exists between the optimal strategy and the learned best response after the player updates its strategies. We call this discrepancy the optimality gap. During training, the optimality gap does not decay monotonically, which causes suboptimal results or unstable convergence of NFSP. We improve the performance of NFSP by allowing the optimality gap to decay monotonically. In this study, we propose Regret Minimization Fictitious Self-Play (RM-FSP), which applies a regret minimization method to compute NFSP's best response. The regret minimization method effectively converges the optimality gap monotonically and faster than in NFSP. We prove there will be a better learning bound than the original NFSP after applying regret minimization methods to NFSP. Experiments on three typical environments in OpenSpiel demonstrate that RM-FSP outperforms NFSP in both exploitability (discrepancy between the learned policy profile and the Nash equilibrium) and time efficiency.& COPY; 2023 Elsevier B.V. All rights reserved.
更多查看译文
关键词
Neural fictitious self-play,Regret minimization,Imperfect-information dynamic games,Reinforcement learning
AI 理解论文
溯源树
样例
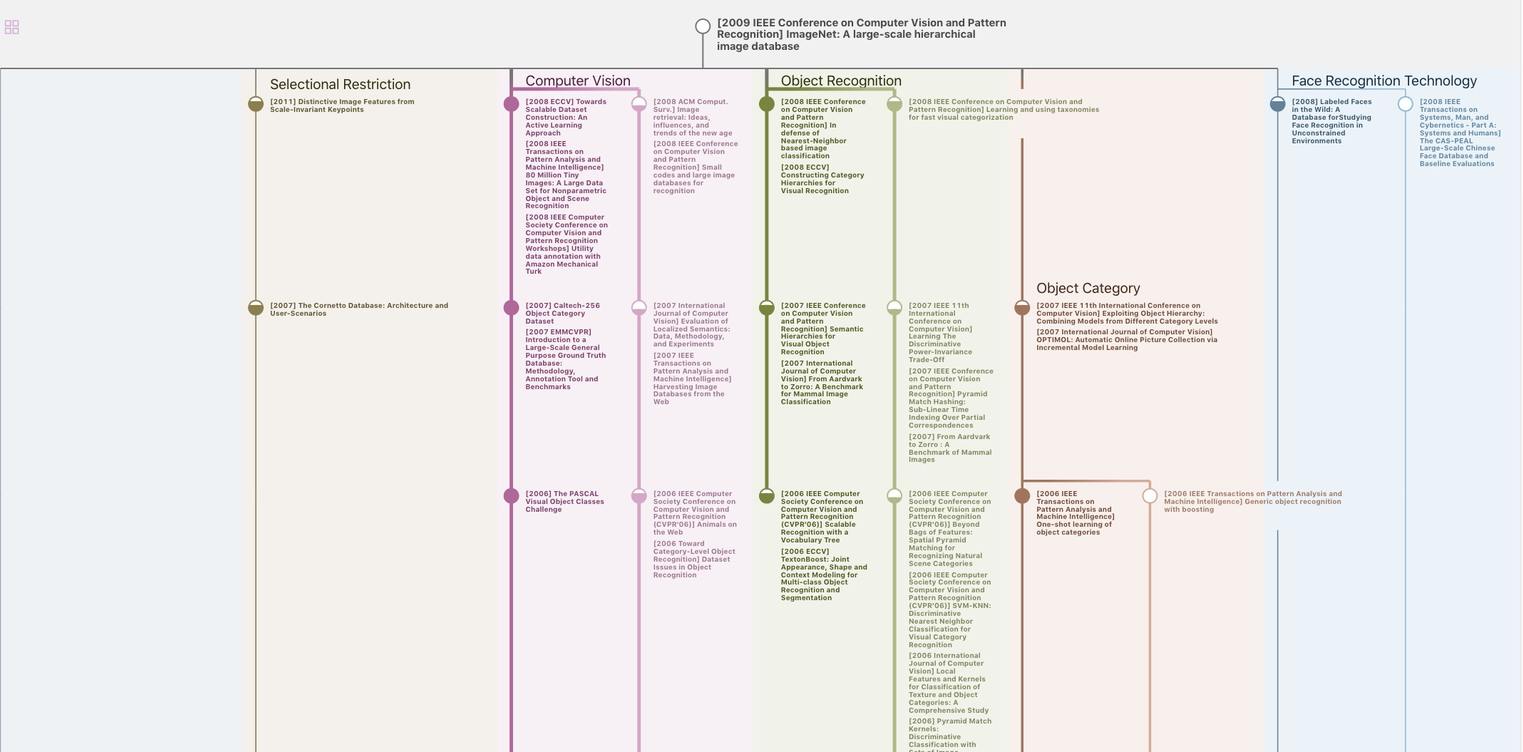
生成溯源树,研究论文发展脉络
Chat Paper
正在生成论文摘要