Class-Incremental Learning Based on Anomaly Detection.
IEEE Access(2023)
摘要
In Class-Incremental Learning (CIL), the memoryless replay CIL algorithm trains the network model by using the closed training set of the current task, while using knowledge distillation or finetuning constraints to keep the old knowledge. Due to the closed set recognition method used in the training process, the training set of each task are independent of each other, and the knowledge learned by the network model cannot effectively deal with the classes that do not appear in the corresponding task training set, that is, the class samples of other tasks cannot be effectively rejected during testing, resulting in the misrecognition of the same test sample in multiple tasks. This is the main factor that the performance of the memoryless replay CIL is limited. In this paper, from the perspective of open set recognition, a CIL algorithm ADCIL based on anomaly detection mechanism is proposed to enhance the discrimination ability of the network model between tasks, so that each task can learn the knowledge that is effective in the open space only through its own training set, and the robustness of the algorithm is greatly improved. It effectively prevents the catastrophic forgetting phenomenon in CIL.
更多查看译文
关键词
Anomaly detection,class increment learning,closed set recognition,catastrophic forgetting,deep learning,open set recognition
AI 理解论文
溯源树
样例
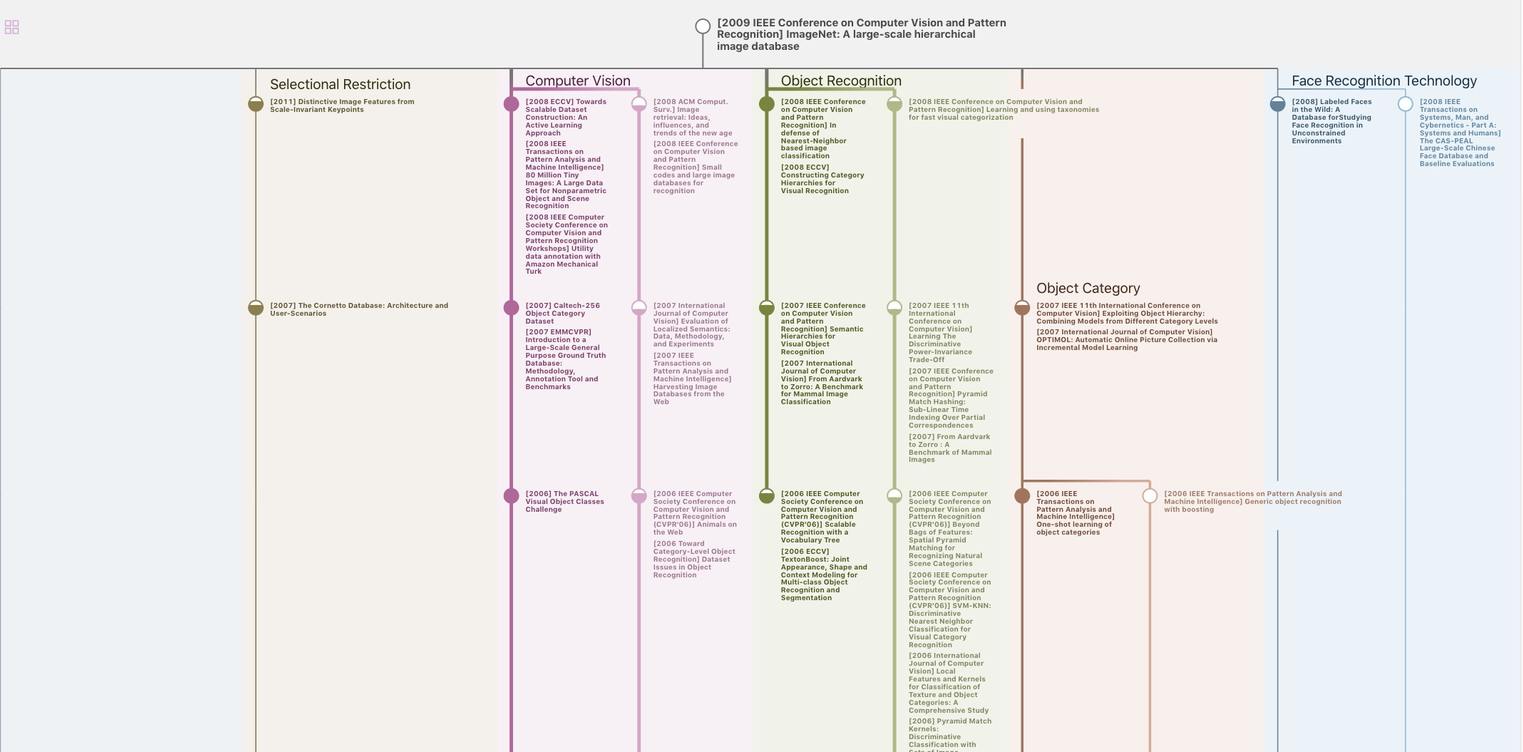
生成溯源树,研究论文发展脉络
Chat Paper
正在生成论文摘要