Large Language Models are Competitive Near Cold-start Recommenders for Language- and Item-based Preferences
PROCEEDINGS OF THE 17TH ACM CONFERENCE ON RECOMMENDER SYSTEMS, RECSYS 2023(2023)
摘要
Traditional recommender systems leverage users' item preference history to recommend novel content that users may like. However, modern dialog interfaces that allow users to express language-based preferences offer a fundamentally different modality for preference input. Inspired by recent successes of prompting paradigms for large language models (LLMs), we study their use for making recommendations from both item-based and language-based preferences in comparison to state-of-the-art item-based collaborative filtering (CF) methods. To support this investigation, we collect a new dataset consisting of both item-based and language-based preferences elicited from users along with their ratings on a variety of (biased) recommended items and (unbiased) random items. Among numerous experimental results, we find that LLMs provide competitive recommendation performance for pure language-based preferences (no item preferences) in the near cold-start case in comparison to item-based CF methods, despite having no supervised training for this specific task (zero-shot) or only a few labels (few-shot). This is particularly promising as language-based preference representations are more explainable and scrutable than item-based or vector-based representations.
更多查看译文
关键词
recommendation,transparency,scrutability,natural language
AI 理解论文
溯源树
样例
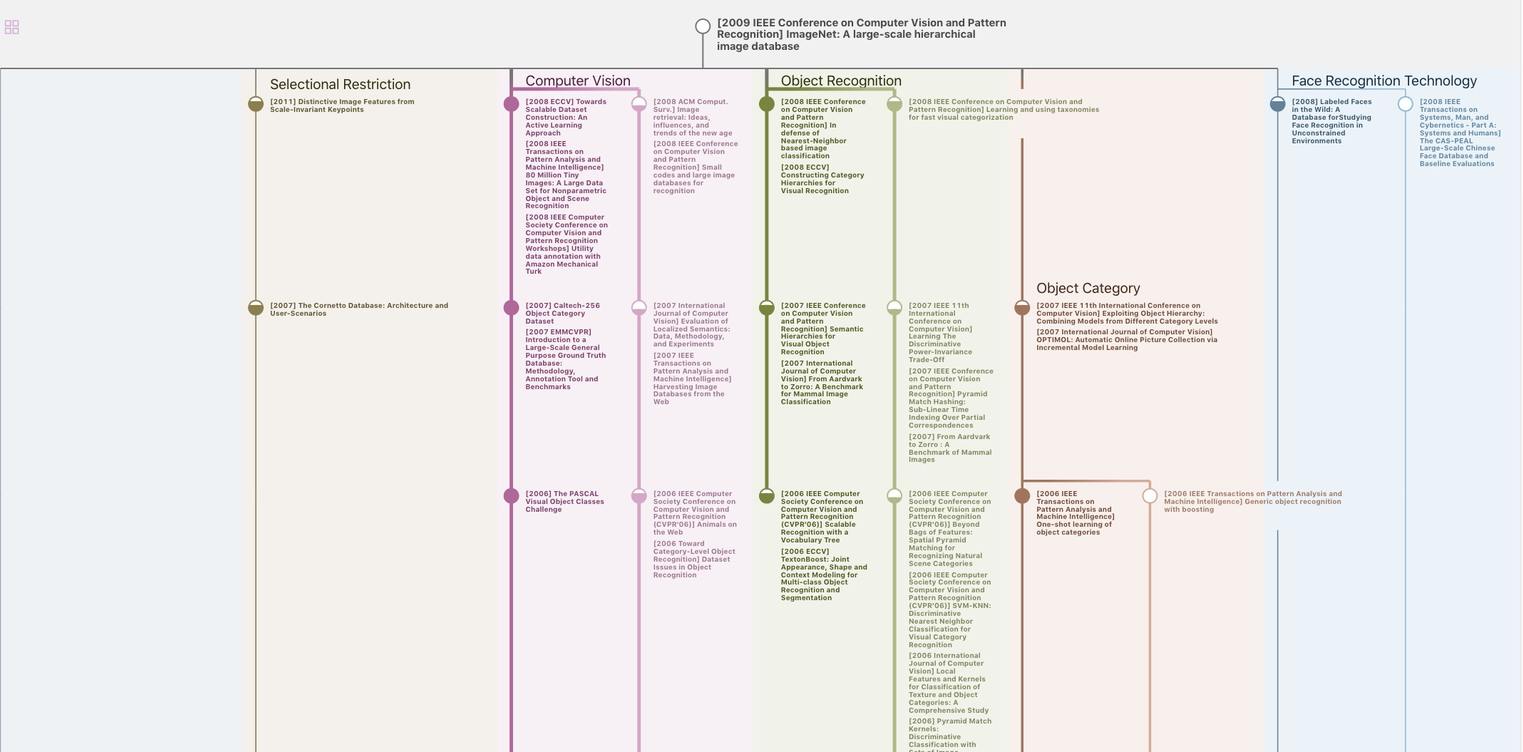
生成溯源树,研究论文发展脉络
Chat Paper
正在生成论文摘要