How do you feel? Measuring User-Perceived Value for Rejecting Machine Decisions in Hate Speech Detection
PROCEEDINGS OF THE 2023 AAAI/ACM CONFERENCE ON AI, ETHICS, AND SOCIETY, AIES 2023(2023)
摘要
Hate speech moderation remains a challenging task for social media platforms. Human-AI collaborative systems offer the potential to combine the strengths of humans' reliability and the scalability of machine learning to tackle this issue effectively. While methods for task handover in human-AI collaboration exist that consider the costs of incorrect predictions, insufficient attention has been paid to accurately estimating these costs. In this work, we propose a value-sensitive rejection mechanism that automatically rejects machine decisions for human moderation based on users' value perceptions regarding machine decisions. We conduct a crowdsourced survey study with 160 participants to evaluate their perception of correct and incorrect machine decisions in the domain of hate speech detection, as well as occurrences where the system rejects making a prediction. Here, we introduce Magnitude Estimation, an unbounded scale, as the preferred method for measuring user (dis)agreement with machine decisions. Our results show that Magnitude Estimation can provide a reliable measurement of participants' perception of machine decisions. By integrating user-perceived value into human-AI collaboration, we further show that it can guide us in 1) determining when to accept or reject machine decisions to obtain the optimal total value a model can deliver and 2) selecting better classification models as compared to the more widely used target of model accuracy.
更多查看译文
关键词
value-sensitive machine learning,rejection,machine confidence,crowdsourcing,human-in-the-loop,hate speech
AI 理解论文
溯源树
样例
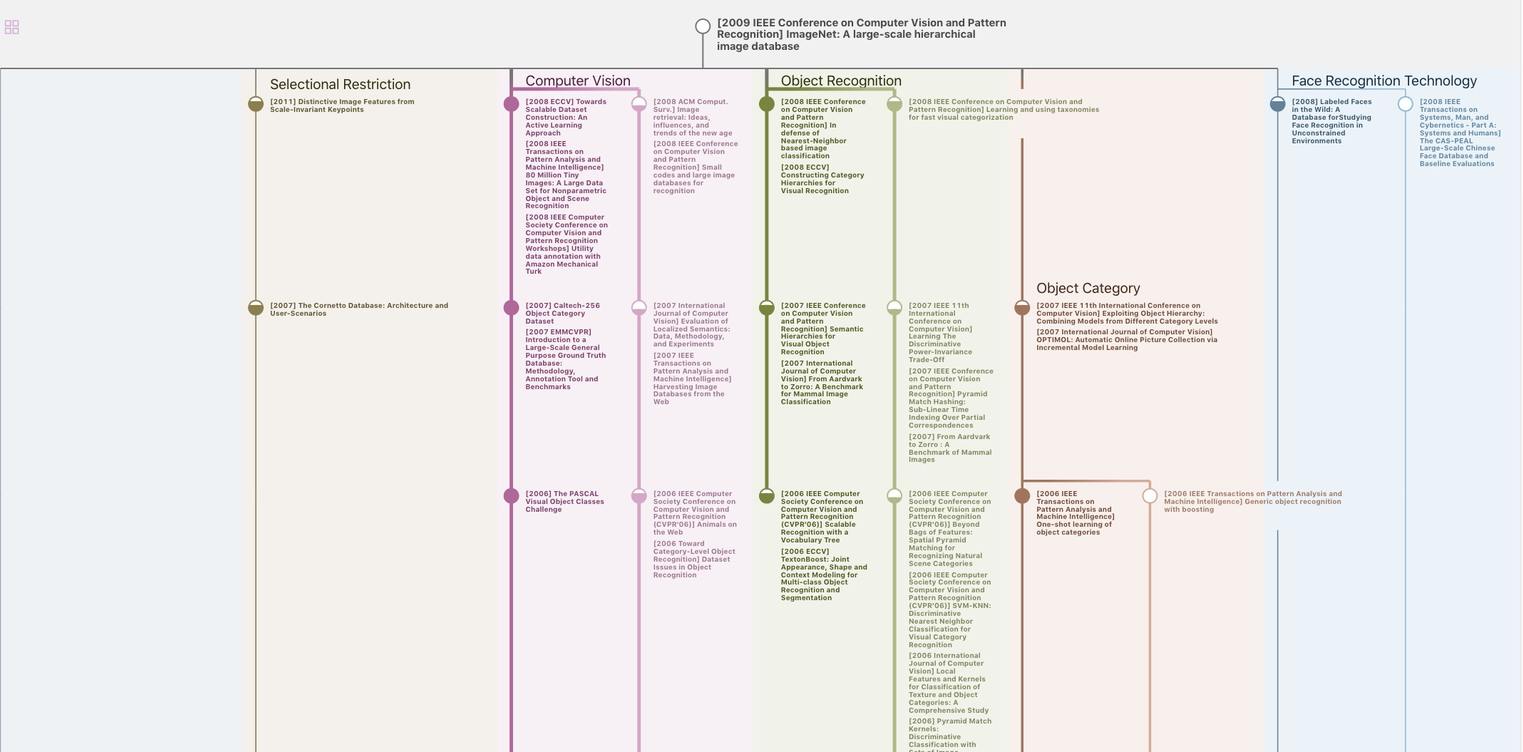
生成溯源树,研究论文发展脉络
Chat Paper
正在生成论文摘要