PAC Neural Prediction Set Learning to Quantify the Uncertainty of Generative Language Models
CoRR(2023)
摘要
Uncertainty learning and quantification of models are crucial tasks to enhance the trustworthiness of the models. Importantly, the recent surge of generative language models (GLMs) emphasizes the need for reliable uncertainty quantification due to the concerns on generating hallucinated facts. In this paper, we propose to learn neural prediction set models that comes with the probably approximately correct (PAC) guarantee for quantifying the uncertainty of GLMs. Unlike existing prediction set models, which are parameterized by a scalar value, we propose to parameterize prediction sets via neural networks, which achieves more precise uncertainty quantification but still satisfies the PAC guarantee. We demonstrate the efficacy of our method on four types of language datasets and six types of models by showing that our method improves the quantified uncertainty by $63\%$ on average, compared to a standard baseline method.
更多查看译文
关键词
prediction,uncertainty,models,learning,neural
AI 理解论文
溯源树
样例
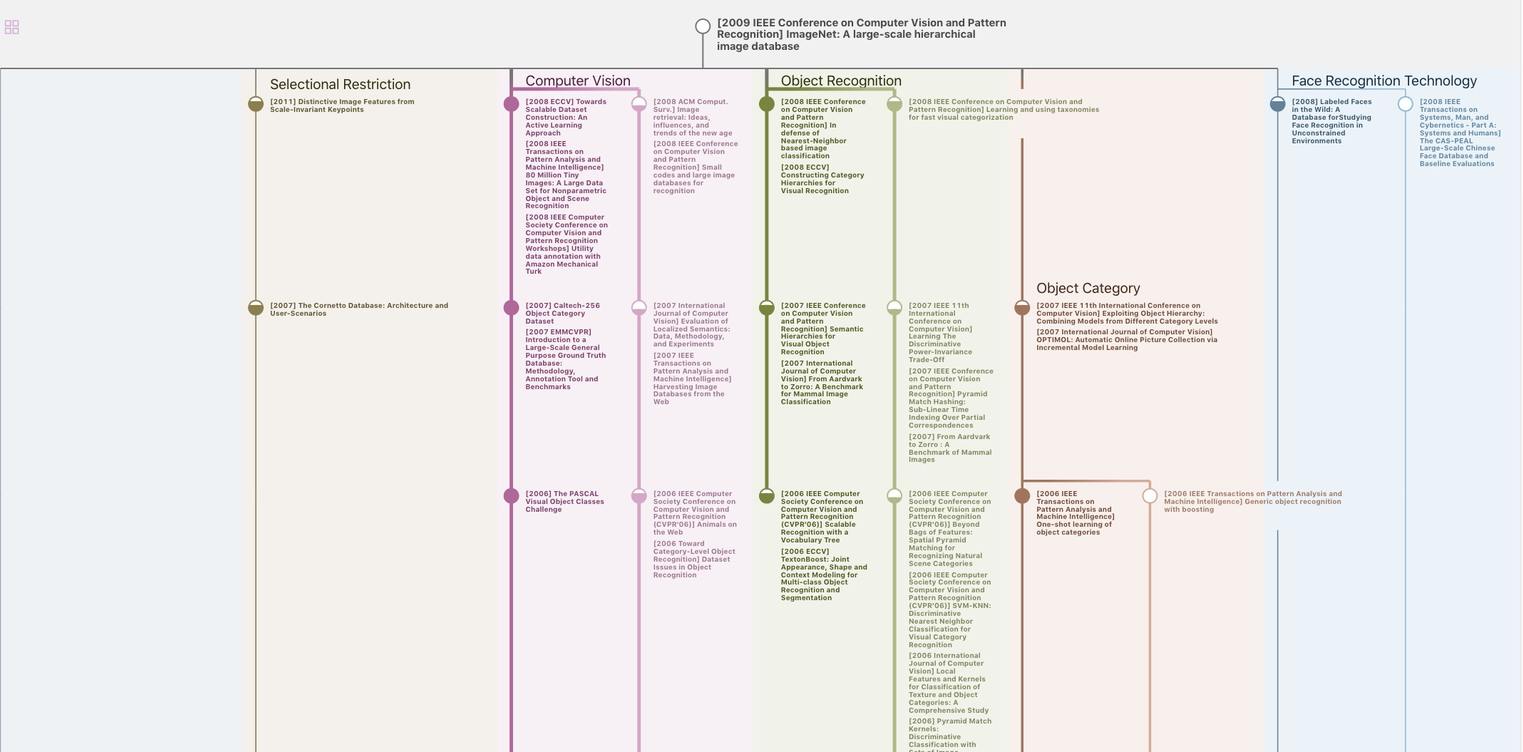
生成溯源树,研究论文发展脉络
Chat Paper
正在生成论文摘要