Learned Scalable Video Coding For Humans and Machines
CoRR(2023)
摘要
Video coding has traditionally been developed to support services such as video streaming, videoconferencing, digital TV, and so on. The main intent was to enable human viewing of the encoded content. However, with the advances in deep neural networks (DNNs), encoded video is increasingly being used for automatic video analytics performed by machines. In applications such as automatic traffic monitoring, analytics such as vehicle detection, tracking and counting, would run continuously, while human viewing could be required occasionally to review potential incidents. To support such applications, a new paradigm for video coding is needed that will facilitate efficient representation and compression of video for both machine and human use in a scalable manner. In this manuscript, we introduce the first end-to-end learnable video codec that supports a machine vision task in its base layer, while its enhancement layer supports input reconstruction for human viewing. The proposed system is constructed based on the concept of conditional coding to achieve better compression gains. Comprehensive experimental evaluations conducted on four standard video datasets demonstrate that our framework outperforms both state-of-the-art learned and conventional video codecs in its base layer, while maintaining comparable performance on the human vision task in its enhancement layer. We will provide the implementation of the proposed system at www.github.com upon completion of the review process.
更多查看译文
关键词
scalable video coding
AI 理解论文
溯源树
样例
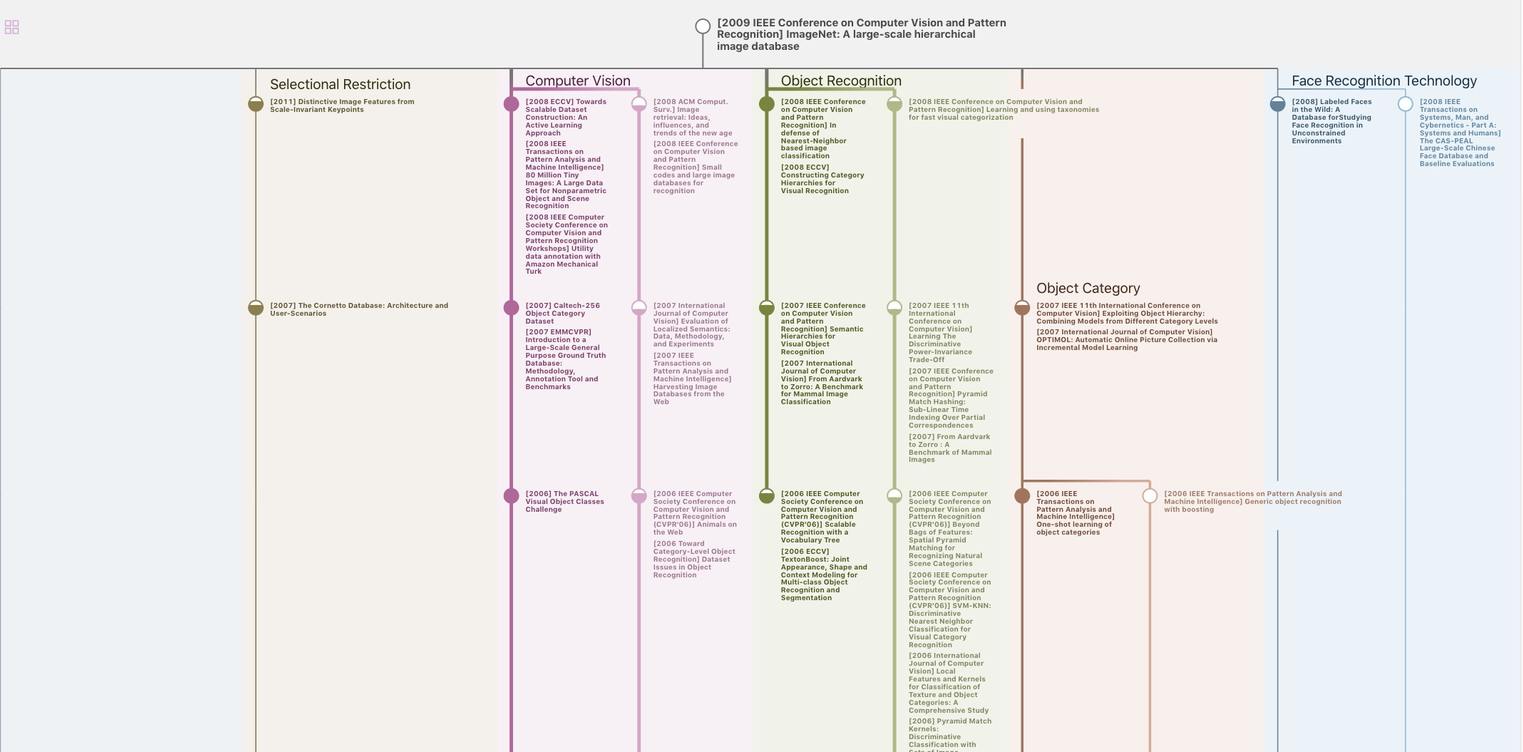
生成溯源树,研究论文发展脉络
Chat Paper
正在生成论文摘要