Towards Equitable Assignment: Data-Driven Delivery Zone Partition at Last-mile Logistics.
KDD(2023)
摘要
The popularity of online e-commerce has promoted the rapid development of last-mile logistics in recent years. In last-mile services, to ensure delivery efficiency and enhance user experience, the delivery zone is proposed to perform delivery task assignment, which is a fundamental part of last-mile delivery. Each courier is responsible for one delivery zone. Couriers will collect orders belonging to their delivery zones from the delivery station and deliver orders to customers. Existing delivery zone partition practices in last-mile logistics consist of manual experience-based and static optimization-based methods, which perform order amount balancing among different zone but suffer from dissatisfaction and inefficiency because of two limitations: (i) using order amount is not always a good balancing metric considering deliveries' various difficulties (e.g., residence or industrial park, with or without elevators); (ii) less considering couriers' familiarity and preference behaviors. To generate delivery zone partition with equitable workload assignment, in this paper, we propose E-partition, a data-driven delivery zone partition framework to achieve equitable workload assignment in last-mile logistics. We first design a learning-based workload prediction model to estimate service time given a partition plan that consists of unseen courier-zone matching scenarios. Then, a delivery zone partition algorithm is proposed to iterative optimize couriers' core-AOI (i.e., area of interest) generation and AOI assignment process. Extensive offline experimental results show that our model outperforms baselines in working time prediction and workload balancing performances. Real-world deployment results at JD Logistics also verify the effectiveness of equitable-assignment aware delivery zone partition, with a 2.2% increase in service on-time rate compared to state-of-practice partition solutions.
更多查看译文
关键词
Last-mile logistics,Delivery zone partition,Machine learning,Combinational optimization
AI 理解论文
溯源树
样例
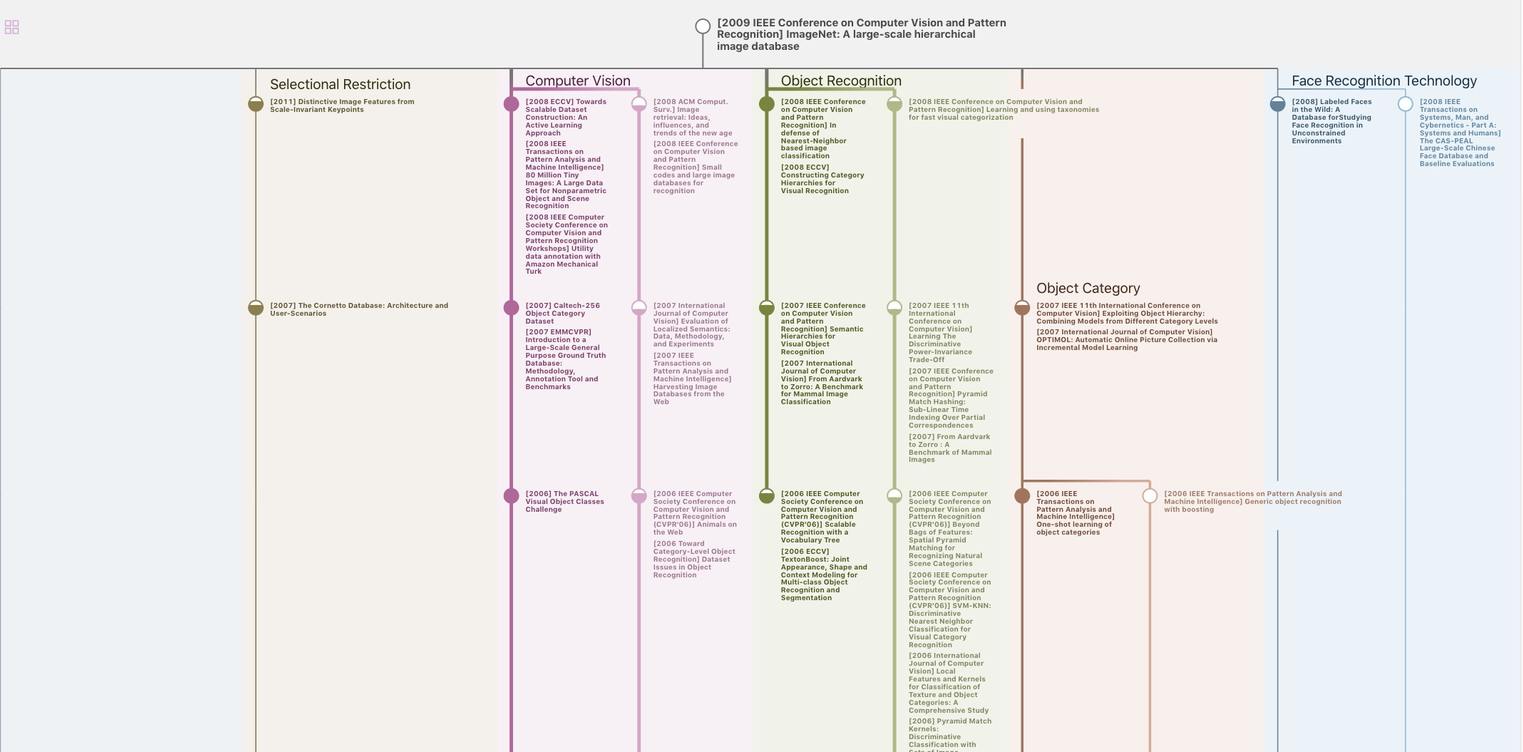
生成溯源树,研究论文发展脉络
Chat Paper
正在生成论文摘要