Learning from Positive and Unlabeled Multi-Instance Bags in Anomaly Detection.
KDD(2023)
摘要
In the multi-instance learning (MIL) setting instances are grouped together into bags. Labels are provided only for the bags and not on the level of individual instances. A positive bag label means that at least one instance inside the bag is positive, while a negative bag label restricts all the instances in the bag to be negative. MIL data naturally arises in many contexts, such as anomaly detection, where labels are rare and costly, and one often ends up annotating the label for sets of instances. Moreover, in many real-world anomaly detection problems, only positive labels are collected because they usually represent critical events. Such a setting, where only positive labels are provided along with unlabeled data, is called Positive and Unlabeled (PU) learning. Despite being useful for several use cases, there is no work dedicated to learning from positive and unlabeled data in a multi-instance setting for anomaly detection. Therefore, we propose the first method that learns from PU bags in anomaly detection. Our method uses an autoencoder as an underlying anomaly detector. We alter the autoencoder's objective function and propose a new loss that allows it to learn from positive and unlabeled bags of instances. We theoretically analyze this method. Experimentally, we evaluate our method on 30 datasets and show that it performs better than multiple baselines adapted to work in our setting.
更多查看译文
关键词
Multi-Instance Learning,PU Learning,Anomaly Detection
AI 理解论文
溯源树
样例
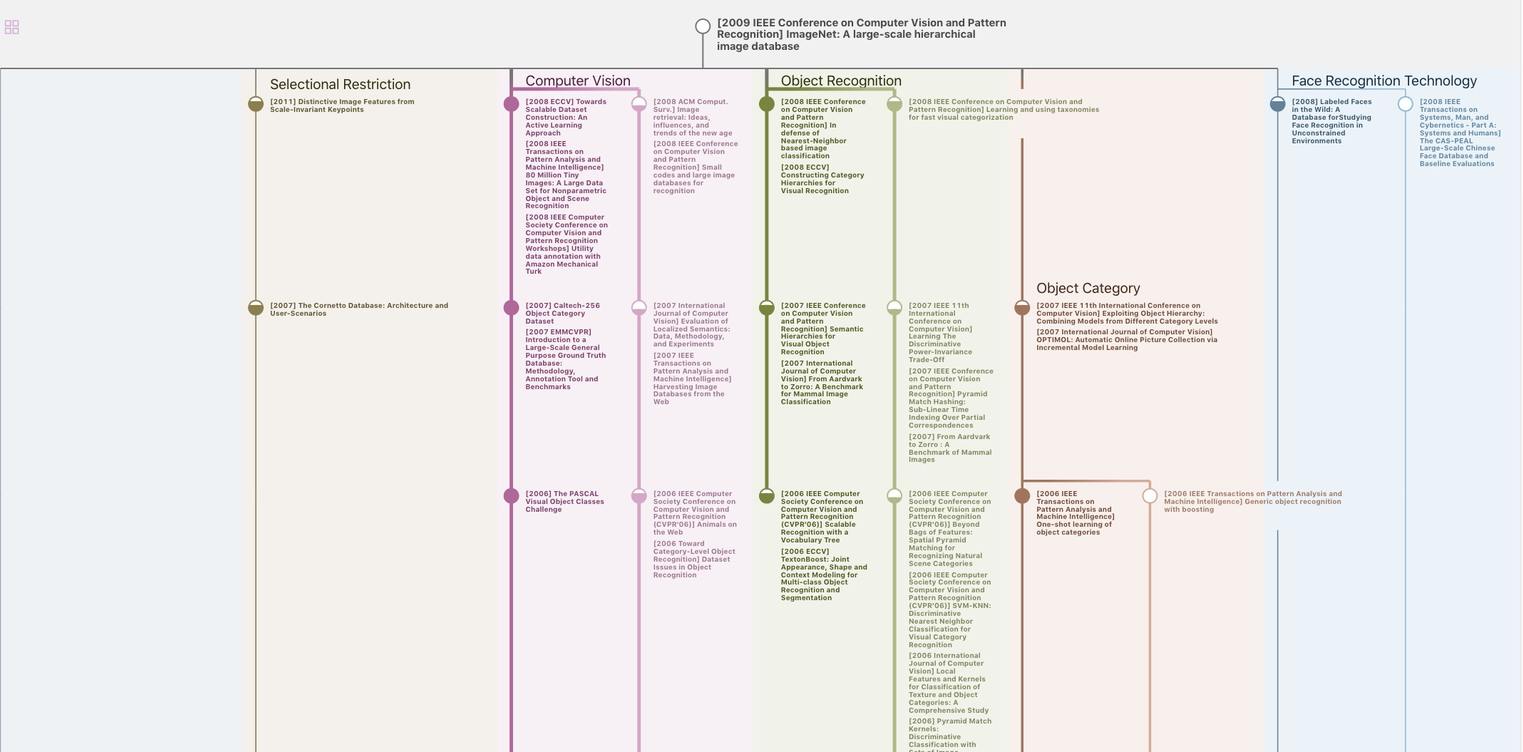
生成溯源树,研究论文发展脉络
Chat Paper
正在生成论文摘要