Multi-dimensional Bayesian network classifiers for partial label ranking
International Journal of Approximate Reasoning(2023)
摘要
The label ranking problem consists in learning preference models from training datasets labeled with (possibly incomplete) rankings of the class labels. The goal is then to predict a ranking for a given unlabeled instance. This work focuses on a more general interpretation where both the training dataset and the prediction given as output allow tied class labels, i.e., there is no particular preference between them. This problem is known as the partial label ranking problem. This paper tackles the partial label ranking problem by transforming the ranking with ties into a set of discrete variables representing the preference relations (ranked ahead, tied with, and ranked behind) between each pair of class labels. The posterior probabilities for each pair are then used to fill the values of a preference matrix. This preference matrix is the basis for solving the rank aggregation problem required to obtain the output ranking with ties. This paper aims to exploit the resemblance of this problem with multi-label and multi-dimensional classification by studying the use of Bayesian network classifiers to compute the posterior probabilities for the new class structure, i.e., pairs of class labels. In particular, binary relevance with naive Bayes and averaged one-dependence estimators between the new class structure are used to solve the partial label ranking problem. Furthermore, bivariate relationships between all the pairs of class labels are considered. However, the complexity of the model grows significantly, which makes it necessary to reduce the number of allowed bivariate relationships between pairs. Thus, a feature selection method is included to select the more relevant subset of bivariate relationships. The experimental evaluation shows that our proposals are competitive in accuracy with the current instance-based and decision tree induction algorithms. Moreover, they outperform the existing mixture-based probabilistic graphical models, while the algorithms proposed are much faster.
更多查看译文
关键词
Preference learning,(Partial) Label ranking,Naive Bayes,Averaged one-dependence estimators,(Multi-dimensional) Bayesian network classifiers
AI 理解论文
溯源树
样例
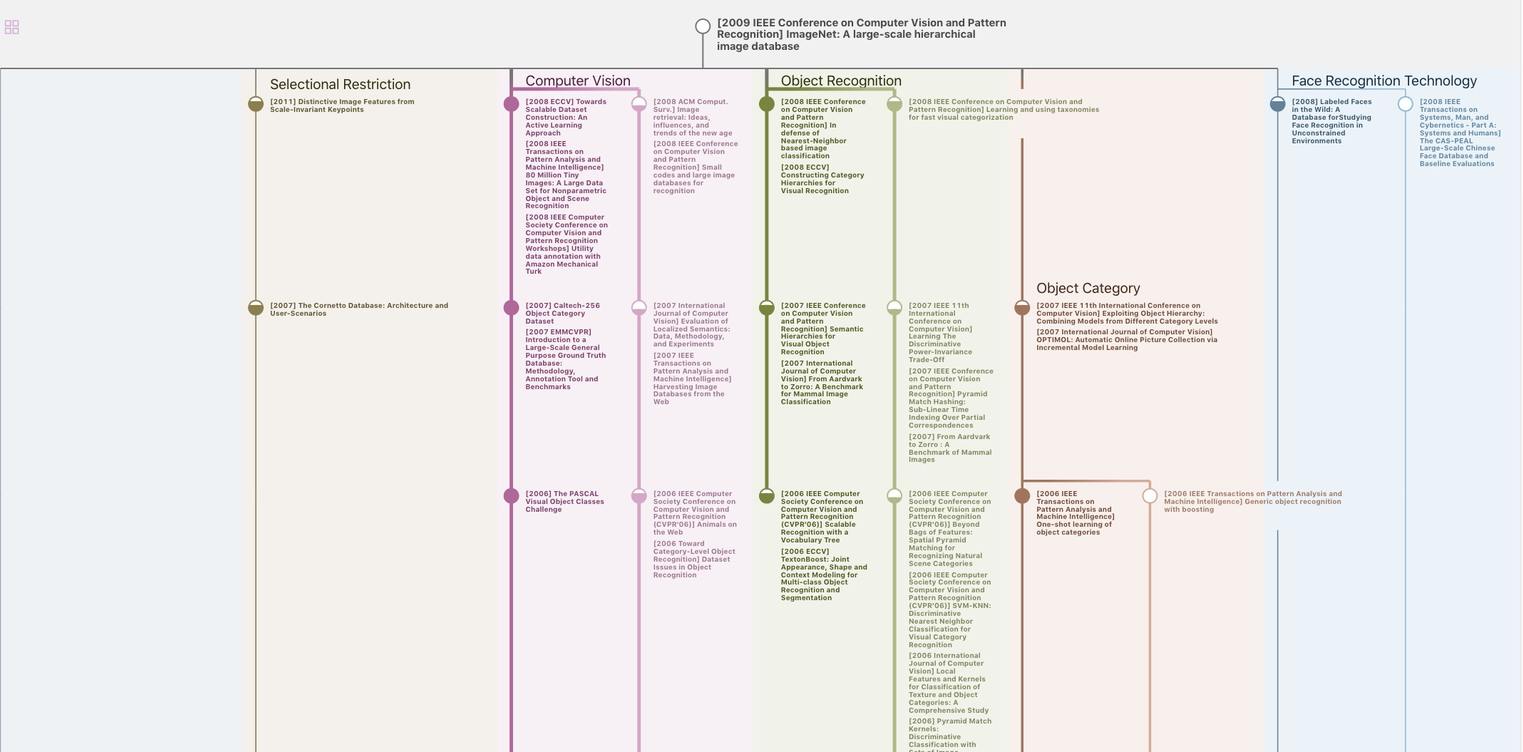
生成溯源树,研究论文发展脉络
Chat Paper
正在生成论文摘要