Weakly supervised real-time instance segmentation for ultrasound images of median nerves
Computers in Biology and Medicine(2023)
摘要
Medical ultrasound technology has garnered significant attention in recent years, with Ultrasound-guided regional anesthesia (UGRA) and carpal tunnel diagnosis (CTS) being two notable examples. Instance segmentation, based on deep learning approaches, is a promising choice to support the analysis of ultrasound data. However, many instance segmentation models cannot achieve the requirement of ultrasound technology e.g. real-time. Moreover, fully supervised instance segmentation models require large numbers of images and corresponding mask annotations for training, which can be time-consuming and labor-intensive in the case of medical ultrasound data. This paper proposes a novel weakly supervised framework, CoarseInst, to achieve real-time instance segmentation of ultrasound images with only box annotations. CoarseInst not only improves the network structure, but also proposes a two-stage “coarse-to-fine” training strategy. Specifically, median nerves are used as the target application for UGRA and CTS. CoarseInst consists of two stages, with pseudo mask labels generated in the coarse mask generation stage for self-training. An object enhancement block is incorporated to mitigate the performance loss caused by parameter reduction in this stage. Additionally, we introduce a pair of loss functions, the amplification loss, and the deflation loss, that work together to generate the masks. A center area mask searching algorithm is also proposed to generate labels for the deflation loss. In the self-training stage, a novel self-feature similarity loss is designed to generate more precise masks. Experimental results on a practical ultrasound dataset demonstrate that CoarseInst could achieve better performance than some state-of-the-art fully supervised works.
更多查看译文
关键词
segmentation,ultrasound images,real-time
AI 理解论文
溯源树
样例
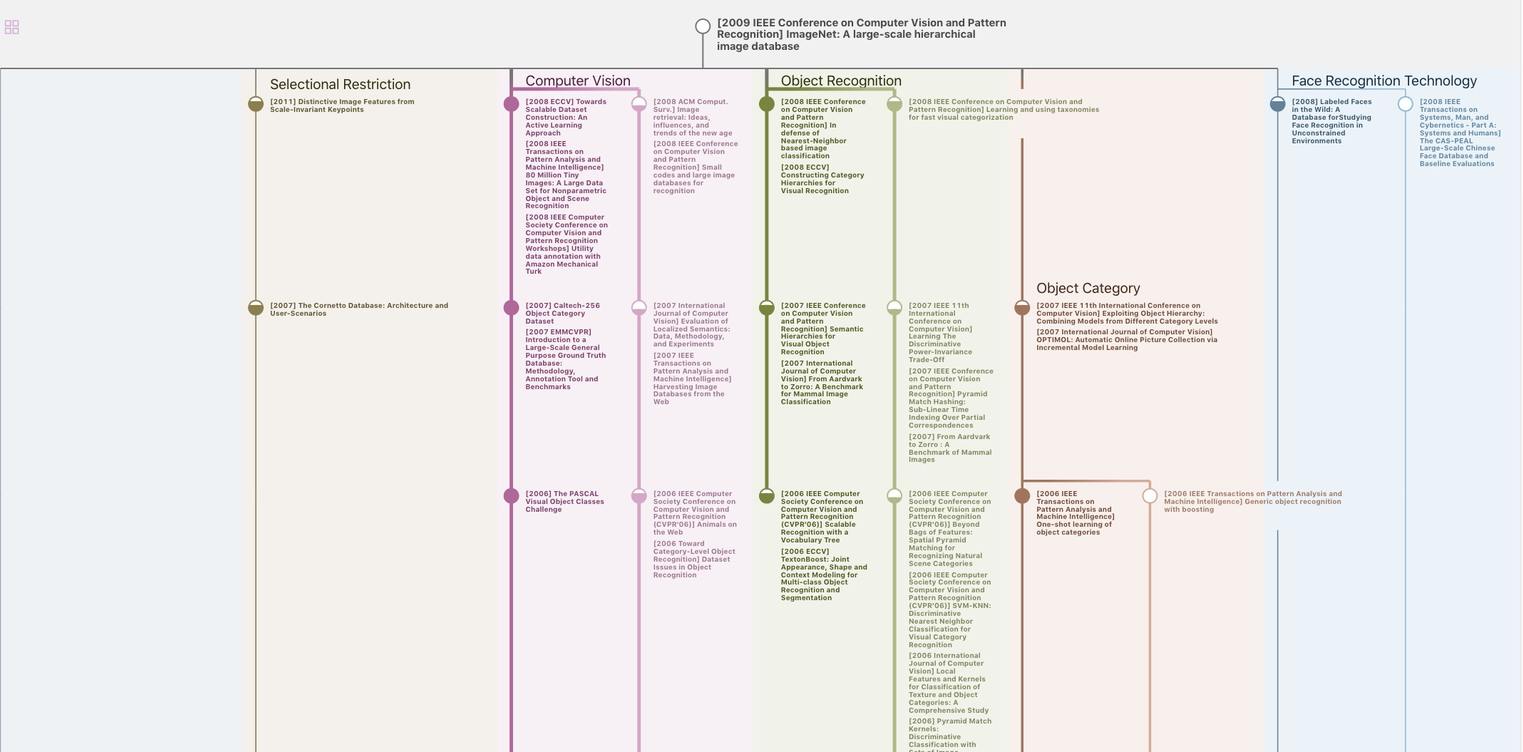
生成溯源树,研究论文发展脉络
Chat Paper
正在生成论文摘要