CasViGE: Learning robust point cloud registration with cascaded visual-geometric encoding
Computer Aided Geometric Design(2023)
摘要
In this work, we present Cascaded Visual-Geometric Encoding (CasViGE), a novel inter-modality feature learning method to improve point cloud registration via the visual information from RGB images. Registering point clouds based on solely the 3D geometric structure suffers from severe matching ambiguity caused by repeated geometric patterns and geometrically less discriminative regions. For this reason, recent methods attempt to inject the visual information from RGB images to obtain more accurate correspondences. They usually first extract the visual and the geometric features independently and then fuse them by point-wise concatenation. However, as 2D and 3D convolutions have different inductive biases, this simplistic method ignores the intrinsic correlation between the two modalities, which harms the distinctiveness of the point descriptors. To address this issue, CasViGE iteratively fuses the inter-modality features by leveraging the inductive biases of both 2D and 3D convolutions, which better considers the correlation between the two modalities. A geometry-centric encoding module first promotes the visual features with 3D convolutions in the geometric space to explicitly embed the geometric information. Next, a vision-centric encoding module casts the fused features back to the image space and enforces the local feature saliency and correlation with 2D convolutions. Extensive experiments on 3DMatch and 3DLoMatch benchmarks have demonstrated the efficacy of our method. Our CasViGE is plug-and-play and attains significant improvements consistently on various point cloud registration methods.
更多查看译文
关键词
robust point cloud registration,visual-geometric
AI 理解论文
溯源树
样例
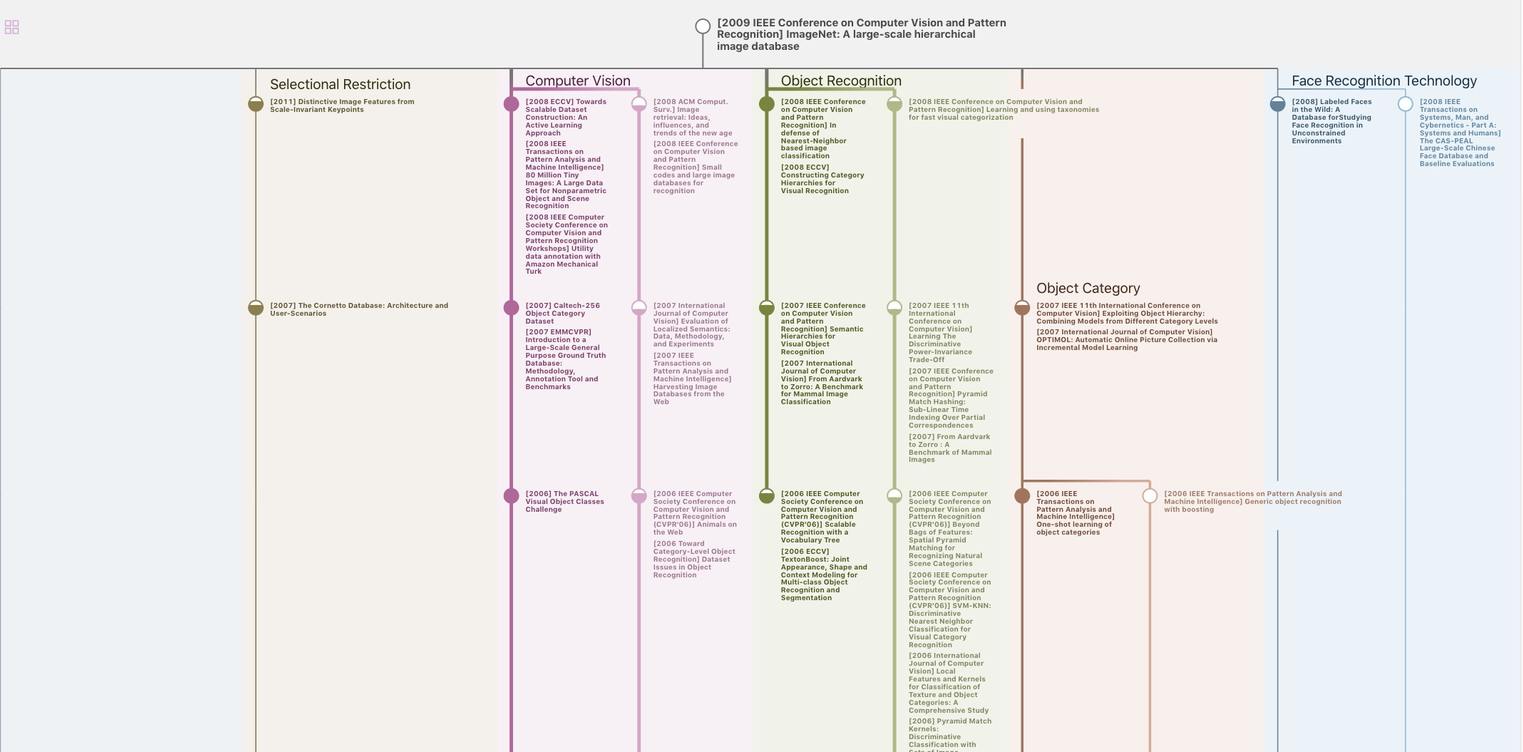
生成溯源树,研究论文发展脉络
Chat Paper
正在生成论文摘要