Open-NAS: A customizable search space for Neural Architecture Search
ICMLT(2023)
摘要
As we advance in the fast-growing era of Machine Learning, various new and more complex neural architectures are arising to tackle problem more efficiently. On the one hand, efficiently deploy them requires advanced knowledge and expertise, which is most of the time difficult to find on the labor market. On the other hand, searching for an optimized neural architecture is a time-consuming task when it is performed manually using a trial-and-error approach. Hence, a method and a tool support are needed to assist users of neural architectures, leading to an eagerness in the field of Automatic Machine Learning (AutoML). When it comes to Deep Learning, an important part of AutoML is the Neural Architecture Search (NAS). In this paper, we propose a formalization for a cell-based search space. The objectives of the proposed approach are to optimize the search-time and to be general enough to handle most of state-of-the-art Convolutional Neural Networks (CNN) architectures, as well as being customizable.
更多查看译文
关键词
Neural Architecture Search,Auto-ML,Evolutionary Algorithm,VGG,CIFAR-10,MNIST
AI 理解论文
溯源树
样例
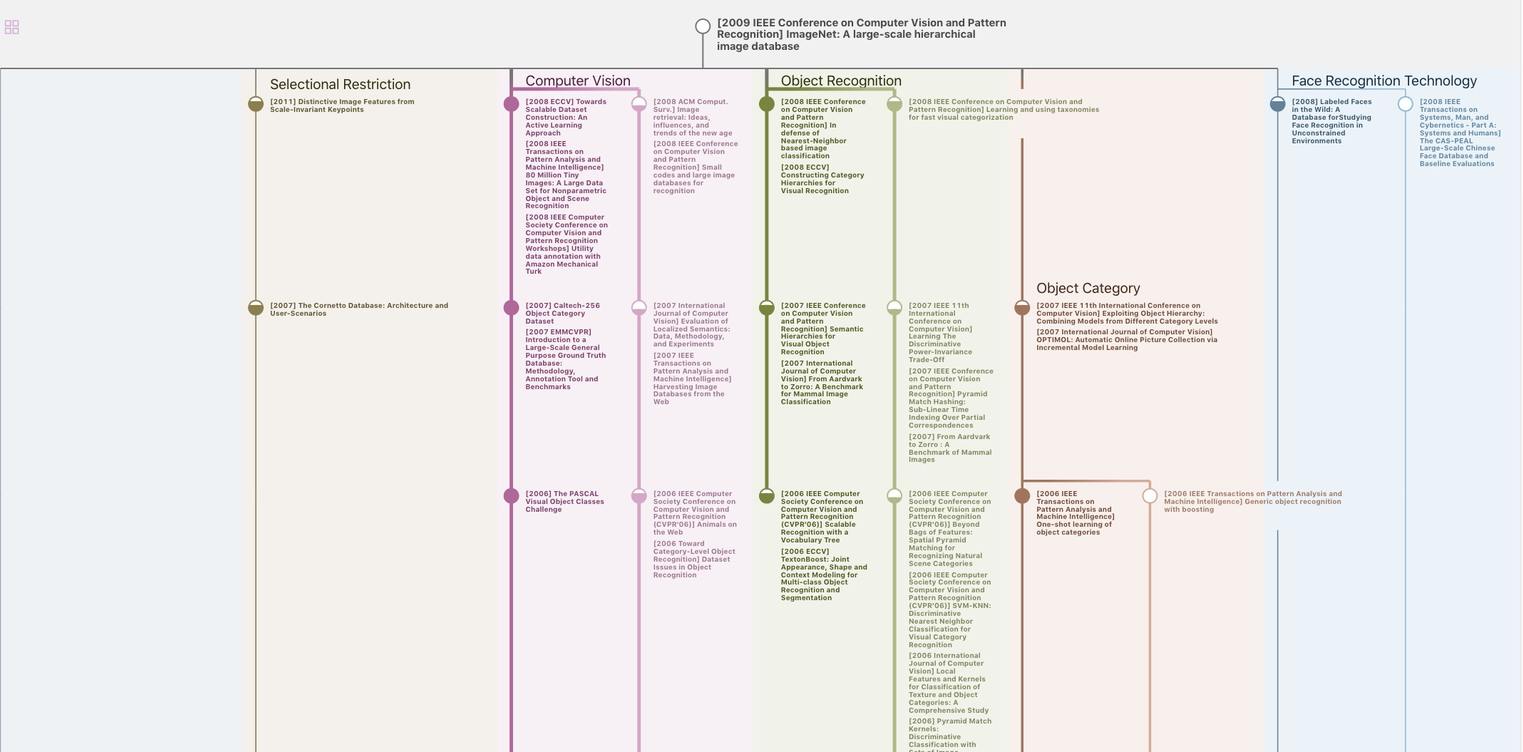
生成溯源树,研究论文发展脉络
Chat Paper
正在生成论文摘要