Analysis of Conventional, Near-Memory, and In-Memory DNN Accelerators.
ISPASS(2023)
摘要
Various DNN accelerators based on Conventional compute Hardware Accelerator (CHA), Near-Data-Processing (NDP) and Processing-in-Memory (PIM) paradigms have been proposed to meet the challenges of inferencing Deep Neural Networks (DNNs). To the best of our knowledge, this work aims to perform the first quantitative as well as qualitative comparison among the state-of-the-art accelerators from each digital DNN accelerator paradigm. Our study provides insights into selecting the best architecture for a given DNN workload. We have used workloads of the MLPerf Inference benchmark. We observe that for Fully Connected Layer (FCL) DNNs, PIM-based accelerator is 21x and 3x faster than CHA and NDP-based accelerator respectively. However, NDP is 9x and 2.5x more energy efficient than CHA and PIM for FCL. For Convolutional Neural Network (CNN) workloads, CHA is 10% and 5x faster than NDP and PIM-based accelerator respectively. Further, CHA is 1.5x and 6x more energy efficient than NDP and PIM-based accelerators respectively.
更多查看译文
关键词
DNN Accelerator,Processing in Memory,Near Data Processor
AI 理解论文
溯源树
样例
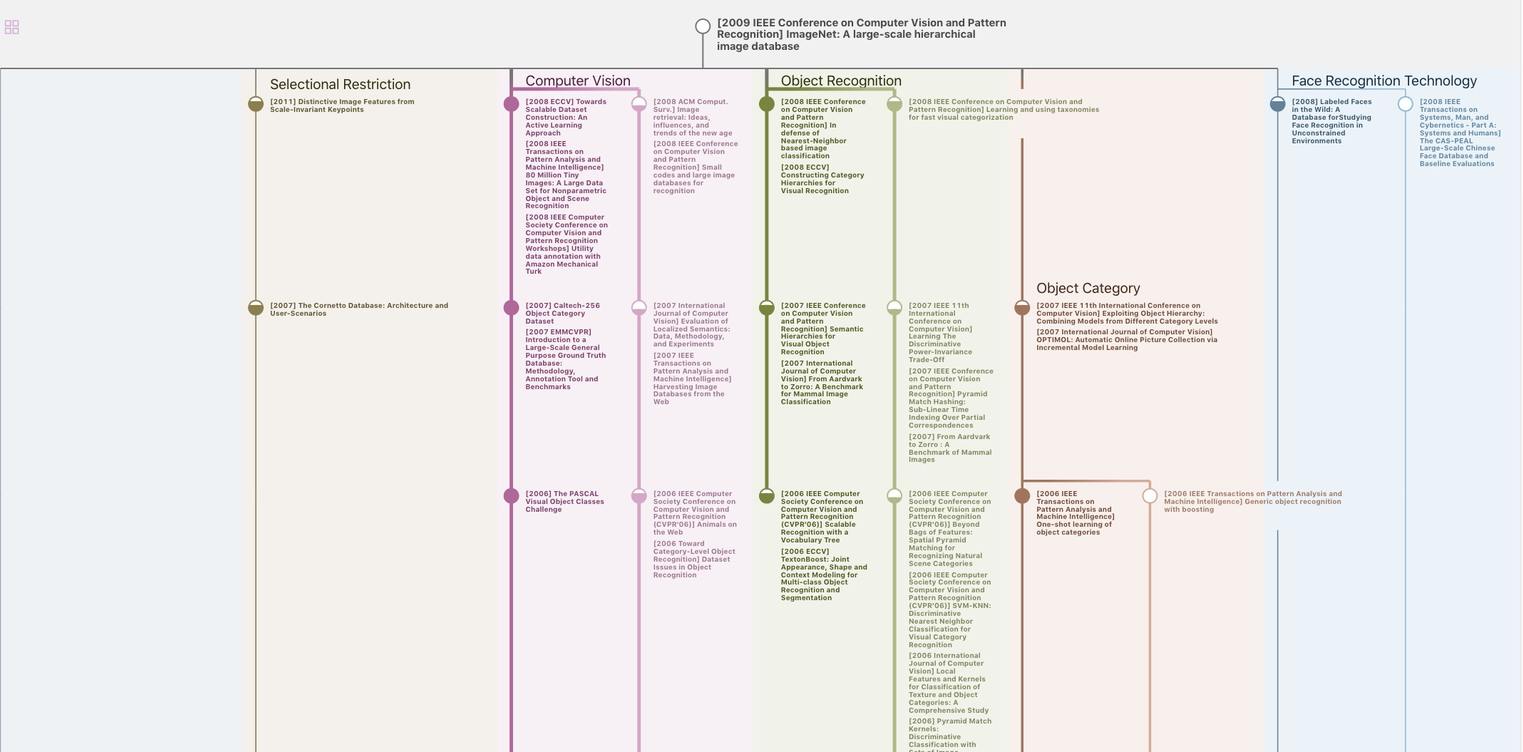
生成溯源树,研究论文发展脉络
Chat Paper
正在生成论文摘要